This library contains classes for launching graphs and executing operations.
-The @{$get_started/get_started} guide has
-examples of how a graph is launched in a @{tf.Session}.
+@{$programmers_guide/low_level_intro$This guide} has examples of how a graph
+is launched in a @{tf.Session}.
## Session management
An example using `placeholder` and feeding to train on MNIST data can be found
in
-[`tensorflow/examples/tutorials/mnist/fully_connected_feed.py`](https://www.tensorflow.org/code/tensorflow/examples/tutorials/mnist/fully_connected_feed.py),
-and is described in the @{$mechanics$MNIST tutorial}.
+[`tensorflow/examples/tutorials/mnist/fully_connected_feed.py`](https://www.tensorflow.org/code/tensorflow/examples/tutorials/mnist/fully_connected_feed.py).
## `QueueRunner`
This document shows how to create a cluster of TensorFlow servers, and how to
distribute a computation graph across that cluster. We assume that you are
-familiar with the @{$get_started/get_started$basic concepts} of
-writing TensorFlow programs.
+familiar with the @{$programmers_guide/low_level_intro$basic concepts} of
+writing low level TensorFlow programs.
## Hello distributed TensorFlow!
This document describes the system architecture that makes possible this
combination of scale and flexibility. It assumes that you have basic familiarity
with TensorFlow programming concepts such as the computation graph, operations,
-and sessions. See @{$get_started/get_started$Getting Started}
+and sessions. See @{$programmers_guide/low_level_intro$this document}
for an introduction to these topics. Some familiarity
with @{$distributed$distributed TensorFlow}
will also be helpful.
+++ /dev/null
-# Creating Estimators in tf.estimator
-
-The tf.estimator framework makes it easy to construct and train machine
-learning models via its high-level Estimator API. `Estimator`
-offers classes you can instantiate to quickly configure common model types such
-as regressors and classifiers:
-
-* @{tf.estimator.LinearClassifier}:
- Constructs a linear classification model.
-* @{tf.estimator.LinearRegressor}:
- Constructs a linear regression model.
-* @{tf.estimator.DNNClassifier}:
- Construct a neural network classification model.
-* @{tf.estimator.DNNRegressor}:
- Construct a neural network regression model.
-* @{tf.estimator.DNNLinearCombinedClassifier}:
- Construct a neural network and linear combined classification model.
-* @{tf.estimator.DNNLinearCombinedRegressor}:
- Construct a neural network and linear combined regression model.
-
-But what if none of `tf.estimator`'s predefined model types meets your needs?
-Perhaps you need more granular control over model configuration, such as
-the ability to customize the loss function used for optimization, or specify
-different activation functions for each neural network layer. Or maybe you're
-implementing a ranking or recommendation system, and neither a classifier nor a
-regressor is appropriate for generating predictions.
-
-This tutorial covers how to create your own `Estimator` using the building
-blocks provided in `tf.estimator`, which will predict the ages of
-[abalones](https://en.wikipedia.org/wiki/Abalone) based on their physical
-measurements. You'll learn how to do the following:
-
-* Instantiate an `Estimator`
-* Construct a custom model function
-* Configure a neural network using `tf.feature_column` and `tf.layers`
-* Choose an appropriate loss function from `tf.losses`
-* Define a training op for your model
-* Generate and return predictions
-
-## Prerequisites
-
-This tutorial assumes you already know tf.estimator API basics, such as
-feature columns, input functions, and `train()`/`evaluate()`/`predict()`
-operations. If you've never used tf.estimator before, or need a refresher,
-you should first review the following tutorials:
-
-* @{$get_started/estimator$tf.estimator Quickstart}: Quick introduction to
- training a neural network using tf.estimator.
-* @{$wide$TensorFlow Linear Model Tutorial}: Introduction to
- feature columns, and an overview on building a linear classifier in
- tf.estimator.
-* @{$input_fn$Building Input Functions with tf.estimator}: Overview of how
- to construct an input_fn to preprocess and feed data into your models.
-
-## An Abalone Age Predictor {#abalone-predictor}
-
-It's possible to estimate the age of an
-[abalone](https://en.wikipedia.org/wiki/Abalone) (sea snail) by the number of
-rings on its shell. However, because this task requires cutting, staining, and
-viewing the shell under a microscope, it's desirable to find other measurements
-that can predict age.
-
-The [Abalone Data Set](https://archive.ics.uci.edu/ml/datasets/Abalone) contains
-the following
-[feature data](https://archive.ics.uci.edu/ml/machine-learning-databases/abalone/abalone.names)
-for abalone:
-
-| Feature | Description |
-| -------------- | --------------------------------------------------------- |
-| Length | Length of abalone (in longest direction; in mm) |
-| Diameter | Diameter of abalone (measurement perpendicular to length; in mm)|
-| Height | Height of abalone (with its meat inside shell; in mm) |
-| Whole Weight | Weight of entire abalone (in grams) |
-| Shucked Weight | Weight of abalone meat only (in grams) |
-| Viscera Weight | Gut weight of abalone (in grams), after bleeding |
-| Shell Weight | Weight of dried abalone shell (in grams) |
-
-The label to predict is number of rings, as a proxy for abalone age.
-
-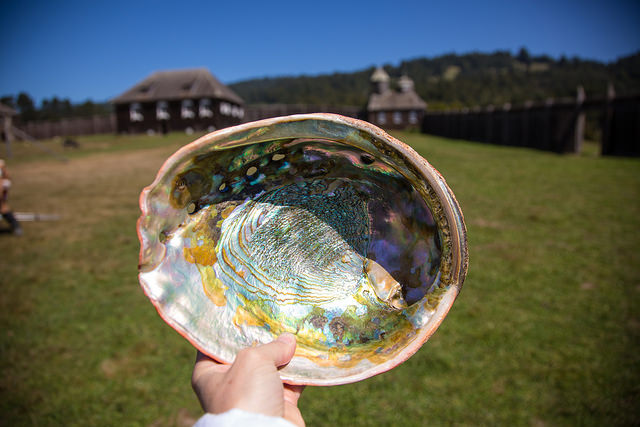
-**[“Abalone shell”](https://www.flickr.com/photos/thenickster/16641048623/) (by [Nicki Dugan
-Pogue](https://www.flickr.com/photos/thenickster/), CC BY-SA 2.0)**
-
-## Setup
-
-This tutorial uses three data sets.
-[`abalone_train.csv`](http://download.tensorflow.org/data/abalone_train.csv)
-contains labeled training data comprising 3,320 examples.
-[`abalone_test.csv`](http://download.tensorflow.org/data/abalone_test.csv)
-contains labeled test data for 850 examples.
-[`abalone_predict`](http://download.tensorflow.org/data/abalone_predict.csv)
-contains 7 examples on which to make predictions.
-
-The following sections walk through writing the `Estimator` code step by step;
-the [full, final code is available
-here](https://www.tensorflow.org/code/tensorflow/examples/tutorials/estimators/abalone.py).
-
-## Loading Abalone CSV Data into TensorFlow Datasets
-
-To feed the abalone dataset into the model, you'll need to download and load the
-CSVs into TensorFlow `Dataset`s. First, add some standard Python and TensorFlow
-imports, and set up FLAGS:
-
-```python
-from __future__ import absolute_import
-from __future__ import division
-from __future__ import print_function
-
-import argparse
-import sys
-import tempfile
-
-# Import urllib
-from six.moves import urllib
-
-import numpy as np
-import tensorflow as tf
-
-FLAGS = None
-```
-
-Enable logging:
-
-```python
-tf.logging.set_verbosity(tf.logging.INFO)
-```
-
-Then define a function to load the CSVs (either from files specified in
-command-line options, or downloaded from
-[tensorflow.org](https://www.tensorflow.org/)):
-
-```python
-def maybe_download(train_data, test_data, predict_data):
- """Maybe downloads training data and returns train and test file names."""
- if train_data:
- train_file_name = train_data
- else:
- train_file = tempfile.NamedTemporaryFile(delete=False)
- urllib.request.urlretrieve(
- "http://download.tensorflow.org/data/abalone_train.csv",
- train_file.name)
- train_file_name = train_file.name
- train_file.close()
- print("Training data is downloaded to %s" % train_file_name)
-
- if test_data:
- test_file_name = test_data
- else:
- test_file = tempfile.NamedTemporaryFile(delete=False)
- urllib.request.urlretrieve(
- "http://download.tensorflow.org/data/abalone_test.csv", test_file.name)
- test_file_name = test_file.name
- test_file.close()
- print("Test data is downloaded to %s" % test_file_name)
-
- if predict_data:
- predict_file_name = predict_data
- else:
- predict_file = tempfile.NamedTemporaryFile(delete=False)
- urllib.request.urlretrieve(
- "http://download.tensorflow.org/data/abalone_predict.csv",
- predict_file.name)
- predict_file_name = predict_file.name
- predict_file.close()
- print("Prediction data is downloaded to %s" % predict_file_name)
-
- return train_file_name, test_file_name, predict_file_name
-```
-
-Finally, create `main()` and load the abalone CSVs into `Datasets`, defining
-flags to allow users to optionally specify CSV files for training, test, and
-prediction datasets via the command line (by default, files will be downloaded
-from [tensorflow.org](https://www.tensorflow.org/)):
-
-```python
-def main(unused_argv):
- # Load datasets
- abalone_train, abalone_test, abalone_predict = maybe_download(
- FLAGS.train_data, FLAGS.test_data, FLAGS.predict_data)
-
- # Training examples
- training_set = tf.contrib.learn.datasets.base.load_csv_without_header(
- filename=abalone_train, target_dtype=np.int, features_dtype=np.float64)
-
- # Test examples
- test_set = tf.contrib.learn.datasets.base.load_csv_without_header(
- filename=abalone_test, target_dtype=np.int, features_dtype=np.float64)
-
- # Set of 7 examples for which to predict abalone ages
- prediction_set = tf.contrib.learn.datasets.base.load_csv_without_header(
- filename=abalone_predict, target_dtype=np.int, features_dtype=np.float64)
-
-if __name__ == "__main__":
- parser = argparse.ArgumentParser()
- parser.register("type", "bool", lambda v: v.lower() == "true")
- parser.add_argument(
- "--train_data", type=str, default="", help="Path to the training data.")
- parser.add_argument(
- "--test_data", type=str, default="", help="Path to the test data.")
- parser.add_argument(
- "--predict_data",
- type=str,
- default="",
- help="Path to the prediction data.")
- FLAGS, unparsed = parser.parse_known_args()
- tf.app.run(main=main, argv=[sys.argv[0]] + unparsed)
-```
-
-## Instantiating an Estimator
-
-When defining a model using one of tf.estimator's provided classes, such as
-`DNNClassifier`, you supply all the configuration parameters right in the
-constructor, e.g.:
-
-```python
-my_nn = tf.estimator.DNNClassifier(feature_columns=[age, height, weight],
- hidden_units=[10, 10, 10],
- activation_fn=tf.nn.relu,
- dropout=0.2,
- n_classes=3,
- optimizer="Adam")
-```
-
-You don't need to write any further code to instruct TensorFlow how to train the
-model, calculate loss, or return predictions; that logic is already baked into
-the `DNNClassifier`.
-
-By contrast, when you're creating your own estimator from scratch, the
-constructor accepts just two high-level parameters for model configuration,
-`model_fn` and `params`:
-
-```python
-nn = tf.estimator.Estimator(model_fn=model_fn, params=model_params)
-```
-
-* `model_fn`: A function object that contains all the aforementioned logic to
- support training, evaluation, and prediction. You are responsible for
- implementing that functionality. The next section, [Constructing the
- `model_fn`](#constructing-modelfn) covers creating a model function in
- detail.
-
-* `params`: An optional dict of hyperparameters (e.g., learning rate, dropout)
- that will be passed into the `model_fn`.
-
-Note: Just like `tf.estimator`'s predefined regressors and classifiers, the
-`Estimator` initializer also accepts the general configuration arguments
-`model_dir` and `config`.
-
-For the abalone age predictor, the model will accept one hyperparameter:
-learning rate. Define `LEARNING_RATE` as a constant at the beginning of your
-code (highlighted in bold below), right after the logging configuration:
-
-<pre class="prettyprint"><code class="lang-python">tf.logging.set_verbosity(tf.logging.INFO)
-
-<strong># Learning rate for the model
-LEARNING_RATE = 0.001</strong></code></pre>
-
-Note: Here, `LEARNING_RATE` is set to `0.001`, but you can tune this value as
-needed to achieve the best results during model training.
-
-Then, add the following code to `main()`, which creates the dict `model_params`
-containing the learning rate and instantiates the `Estimator`:
-
-```python
-# Set model params
-model_params = {"learning_rate": LEARNING_RATE}
-
-# Instantiate Estimator
-nn = tf.estimator.Estimator(model_fn=model_fn, params=model_params)
-```
-
-## Constructing the `model_fn` {#constructing-modelfn}
-
-The basic skeleton for an `Estimator` API model function looks like this:
-
-```python
-def model_fn(features, labels, mode, params):
- # Logic to do the following:
- # 1. Configure the model via TensorFlow operations
- # 2. Define the loss function for training/evaluation
- # 3. Define the training operation/optimizer
- # 4. Generate predictions
- # 5. Return predictions/loss/train_op/eval_metric_ops in EstimatorSpec object
- return EstimatorSpec(mode, predictions, loss, train_op, eval_metric_ops)
-```
-
-The `model_fn` must accept three arguments:
-
-* `features`: A dict containing the features passed to the model via
- `input_fn`.
-* `labels`: A `Tensor` containing the labels passed to the model via
- `input_fn`. Will be empty for `predict()` calls, as these are the values the
- model will infer.
-* `mode`: One of the following @{tf.estimator.ModeKeys} string values
- indicating the context in which the model_fn was invoked:
- * `tf.estimator.ModeKeys.TRAIN` The `model_fn` was invoked in training
- mode, namely via a `train()` call.
- * `tf.estimator.ModeKeys.EVAL`. The `model_fn` was invoked in
- evaluation mode, namely via an `evaluate()` call.
- * `tf.estimator.ModeKeys.PREDICT`. The `model_fn` was invoked in
- predict mode, namely via a `predict()` call.
-
-`model_fn` may also accept a `params` argument containing a dict of
-hyperparameters used for training (as shown in the skeleton above).
-
-The body of the function performs the following tasks (described in detail in the
-sections that follow):
-
-* Configuring the model—here, for the abalone predictor, this will be a neural
- network.
-* Defining the loss function used to calculate how closely the model's
- predictions match the target values.
-* Defining the training operation that specifies the `optimizer` algorithm to
- minimize the loss values calculated by the loss function.
-
-The `model_fn` must return a @{tf.estimator.EstimatorSpec}
-object, which contains the following values:
-
-* `mode` (required). The mode in which the model was run. Typically, you will
- return the `mode` argument of the `model_fn` here.
-
-* `predictions` (required in `PREDICT` mode). A dict that maps key names of
- your choice to `Tensor`s containing the predictions from the model, e.g.:
-
- ```python
- predictions = {"results": tensor_of_predictions}
- ```
-
- In `PREDICT` mode, the dict that you return in `EstimatorSpec` will then be
- returned by `predict()`, so you can construct it in the format in which
- you'd like to consume it.
-
-
-* `loss` (required in `EVAL` and `TRAIN` mode). A `Tensor` containing a scalar
- loss value: the output of the model's loss function (discussed in more depth
- later in [Defining loss for the model](#defining-loss)) calculated over all
- the input examples. This is used in `TRAIN` mode for error handling and
- logging, and is automatically included as a metric in `EVAL` mode.
-
-* `train_op` (required only in `TRAIN` mode). An Op that runs one step of
- training.
-
-* `eval_metric_ops` (optional). A dict of name/value pairs specifying the
- metrics that will be calculated when the model runs in `EVAL` mode. The name
- is a label of your choice for the metric, and the value is the result of
- your metric calculation. The @{tf.metrics}
- module provides predefined functions for a variety of common metrics. The
- following `eval_metric_ops` contains an `"accuracy"` metric calculated using
- `tf.metrics.accuracy`:
-
- ```python
- eval_metric_ops = {
- "accuracy": tf.metrics.accuracy(labels, predictions)
- }
- ```
-
- If you do not specify `eval_metric_ops`, only `loss` will be calculated
- during evaluation.
-
-### Configuring a neural network with `tf.feature_column` and `tf.layers`
-
-Constructing a [neural
-network](https://en.wikipedia.org/wiki/Artificial_neural_network) entails
-creating and connecting the input layer, the hidden layers, and the output
-layer.
-
-The input layer is a series of nodes (one for each feature in the model) that
-will accept the feature data that is passed to the `model_fn` in the `features`
-argument. If `features` contains an n-dimensional `Tensor` with all your feature
-data, then it can serve as the input layer.
-If `features` contains a dict of @{$linear#feature-columns-and-transformations$feature columns} passed to
-the model via an input function, you can convert it to an input-layer `Tensor`
-with the @{tf.feature_column.input_layer} function.
-
-```python
-input_layer = tf.feature_column.input_layer(
- features=features, feature_columns=[age, height, weight])
-```
-
-As shown above, `input_layer()` takes two required arguments:
-
-* `features`. A mapping from string keys to the `Tensors` containing the
- corresponding feature data. This is exactly what is passed to the `model_fn`
- in the `features` argument.
-* `feature_columns`. A list of all the `FeatureColumns` in the model—`age`,
- `height`, and `weight` in the above example.
-
-The input layer of the neural network then must be connected to one or more
-hidden layers via an [activation
-function](https://en.wikipedia.org/wiki/Activation_function) that performs a
-nonlinear transformation on the data from the previous layer. The last hidden
-layer is then connected to the output layer, the final layer in the model.
-`tf.layers` provides the `tf.layers.dense` function for constructing fully
-connected layers. The activation is controlled by the `activation` argument.
-Some options to pass to the `activation` argument are:
-
-* `tf.nn.relu`. The following code creates a layer of `units` nodes fully
- connected to the previous layer `input_layer` with a
- [ReLU activation function](https://en.wikipedia.org/wiki/Rectifier_\(neural_networks\))
- (@{tf.nn.relu}):
-
- ```python
- hidden_layer = tf.layers.dense(
- inputs=input_layer, units=10, activation=tf.nn.relu)
- ```
-
-* `tf.nn.relu6`. The following code creates a layer of `units` nodes fully
- connected to the previous layer `hidden_layer` with a ReLU 6 activation
- function (@{tf.nn.relu6}):
-
- ```python
- second_hidden_layer = tf.layers.dense(
- inputs=hidden_layer, units=20, activation=tf.nn.relu)
- ```
-
-* `None`. The following code creates a layer of `units` nodes fully connected
- to the previous layer `second_hidden_layer` with *no* activation function,
- just a linear transformation:
-
- ```python
- output_layer = tf.layers.dense(
- inputs=second_hidden_layer, units=3, activation=None)
- ```
-
-Other activation functions are possible, e.g.:
-
-```python
-output_layer = tf.layers.dense(inputs=second_hidden_layer,
- units=10,
- activation_fn=tf.sigmoid)
-```
-
-The above code creates the neural network layer `output_layer`, which is fully
-connected to `second_hidden_layer` with a sigmoid activation function
-(@{tf.sigmoid}). For a list of predefined
-activation functions available in TensorFlow, see the @{$python/nn#activation_functions$API docs}.
-
-Putting it all together, the following code constructs a full neural network for
-the abalone predictor, and captures its predictions:
-
-```python
-def model_fn(features, labels, mode, params):
- """Model function for Estimator."""
-
- # Connect the first hidden layer to input layer
- # (features["x"]) with relu activation
- first_hidden_layer = tf.layers.dense(features["x"], 10, activation=tf.nn.relu)
-
- # Connect the second hidden layer to first hidden layer with relu
- second_hidden_layer = tf.layers.dense(
- first_hidden_layer, 10, activation=tf.nn.relu)
-
- # Connect the output layer to second hidden layer (no activation fn)
- output_layer = tf.layers.dense(second_hidden_layer, 1)
-
- # Reshape output layer to 1-dim Tensor to return predictions
- predictions = tf.reshape(output_layer, [-1])
- predictions_dict = {"ages": predictions}
- ...
-```
-
-Here, because you'll be passing the abalone `Datasets` using `numpy_input_fn`
-as shown below, `features` is a dict `{"x": data_tensor}`, so
-`features["x"]` is the input layer. The network contains two hidden
-layers, each with 10 nodes and a ReLU activation function. The output layer
-contains no activation function, and is
-@{tf.reshape} to a one-dimensional
-tensor to capture the model's predictions, which are stored in
-`predictions_dict`.
-
-### Defining loss for the model {#defining-loss}
-
-The `EstimatorSpec` returned by the `model_fn` must contain `loss`: a `Tensor`
-representing the loss value, which quantifies how well the model's predictions
-reflect the label values during training and evaluation runs. The @{tf.losses}
-module provides convenience functions for calculating loss using a variety of
-metrics, including:
-
-* `absolute_difference(labels, predictions)`. Calculates loss using the
- [absolute-difference
- formula](https://en.wikipedia.org/wiki/Deviation_\(statistics\)#Unsigned_or_absolute_deviation)
- (also known as L<sub>1</sub> loss).
-
-* `log_loss(labels, predictions)`. Calculates loss using the [logistic loss
- forumula](https://en.wikipedia.org/wiki/Loss_functions_for_classification#Logistic_loss)
- (typically used in logistic regression).
-
-* `mean_squared_error(labels, predictions)`. Calculates loss using the [mean
- squared error](https://en.wikipedia.org/wiki/Mean_squared_error) (MSE; also
- known as L<sub>2</sub> loss).
-
-The following example adds a definition for `loss` to the abalone `model_fn`
-using `mean_squared_error()` (in bold):
-
-<pre class="prettyprint"><code class="lang-python">def model_fn(features, labels, mode, params):
- """Model function for Estimator."""
-
- # Connect the first hidden layer to input layer
- # (features["x"]) with relu activation
- first_hidden_layer = tf.layers.dense(features["x"], 10, activation=tf.nn.relu)
-
- # Connect the second hidden layer to first hidden layer with relu
- second_hidden_layer = tf.layers.dense(
- first_hidden_layer, 10, activation=tf.nn.relu)
-
- # Connect the output layer to second hidden layer (no activation fn)
- output_layer = tf.layers.dense(second_hidden_layer, 1)
-
- # Reshape output layer to 1-dim Tensor to return predictions
- predictions = tf.reshape(output_layer, [-1])
- predictions_dict = {"ages": predictions}
-
-
- <strong># Calculate loss using mean squared error
- loss = tf.losses.mean_squared_error(labels, predictions)</strong>
- ...</code></pre>
-
-See the @{tf.losses$API guide} for a
-full list of loss functions and more details on supported arguments and usage.
-
-Supplementary metrics for evaluation can be added to an `eval_metric_ops` dict.
-The following code defines an `rmse` metric, which calculates the root mean
-squared error for the model predictions. Note that the `labels` tensor is cast
-to a `float64` type to match the data type of the `predictions` tensor, which
-will contain real values:
-
-```python
-eval_metric_ops = {
- "rmse": tf.metrics.root_mean_squared_error(
- tf.cast(labels, tf.float64), predictions)
-}
-```
-
-### Defining the training op for the model
-
-The training op defines the optimization algorithm TensorFlow will use when
-fitting the model to the training data. Typically when training, the goal is to
-minimize loss. A simple way to create the training op is to instantiate a
-`tf.train.Optimizer` subclass and call the `minimize` method.
-
-The following code defines a training op for the abalone `model_fn` using the
-loss value calculated in [Defining Loss for the Model](#defining-loss), the
-learning rate passed to the function in `params`, and the gradient descent
-optimizer. For `global_step`, the convenience function
-@{tf.train.get_global_step} takes care of generating an integer variable:
-
-```python
-optimizer = tf.train.GradientDescentOptimizer(
- learning_rate=params["learning_rate"])
-train_op = optimizer.minimize(
- loss=loss, global_step=tf.train.get_global_step())
-```
-
-For a full list of optimizers, and other details, see the
-@{$python/train#optimizers$API guide}.
-
-### The complete abalone `model_fn`
-
-Here's the final, complete `model_fn` for the abalone age predictor. The
-following code configures the neural network; defines loss and the training op;
-and returns a `EstimatorSpec` object containing `mode`, `predictions_dict`, `loss`,
-and `train_op`:
-
-```python
-def model_fn(features, labels, mode, params):
- """Model function for Estimator."""
-
- # Connect the first hidden layer to input layer
- # (features["x"]) with relu activation
- first_hidden_layer = tf.layers.dense(features["x"], 10, activation=tf.nn.relu)
-
- # Connect the second hidden layer to first hidden layer with relu
- second_hidden_layer = tf.layers.dense(
- first_hidden_layer, 10, activation=tf.nn.relu)
-
- # Connect the output layer to second hidden layer (no activation fn)
- output_layer = tf.layers.dense(second_hidden_layer, 1)
-
- # Reshape output layer to 1-dim Tensor to return predictions
- predictions = tf.reshape(output_layer, [-1])
-
- # Provide an estimator spec for `ModeKeys.PREDICT`.
- if mode == tf.estimator.ModeKeys.PREDICT:
- return tf.estimator.EstimatorSpec(
- mode=mode,
- predictions={"ages": predictions})
-
- # Calculate loss using mean squared error
- loss = tf.losses.mean_squared_error(labels, predictions)
-
- # Calculate root mean squared error as additional eval metric
- eval_metric_ops = {
- "rmse": tf.metrics.root_mean_squared_error(
- tf.cast(labels, tf.float64), predictions)
- }
-
- optimizer = tf.train.GradientDescentOptimizer(
- learning_rate=params["learning_rate"])
- train_op = optimizer.minimize(
- loss=loss, global_step=tf.train.get_global_step())
-
- # Provide an estimator spec for `ModeKeys.EVAL` and `ModeKeys.TRAIN` modes.
- return tf.estimator.EstimatorSpec(
- mode=mode,
- loss=loss,
- train_op=train_op,
- eval_metric_ops=eval_metric_ops)
-```
-
-## Running the Abalone Model
-
-You've instantiated an `Estimator` for the abalone predictor and defined its
-behavior in `model_fn`; all that's left to do is train, evaluate, and make
-predictions.
-
-Add the following code to the end of `main()` to fit the neural network to the
-training data and evaluate accuracy:
-
-```python
-train_input_fn = tf.estimator.inputs.numpy_input_fn(
- x={"x": np.array(training_set.data)},
- y=np.array(training_set.target),
- num_epochs=None,
- shuffle=True)
-
-# Train
-nn.train(input_fn=train_input_fn, steps=5000)
-
-# Score accuracy
-test_input_fn = tf.estimator.inputs.numpy_input_fn(
- x={"x": np.array(test_set.data)},
- y=np.array(test_set.target),
- num_epochs=1,
- shuffle=False)
-
-ev = nn.evaluate(input_fn=test_input_fn)
-print("Loss: %s" % ev["loss"])
-print("Root Mean Squared Error: %s" % ev["rmse"])
-```
-
-Note: The above code uses input functions to feed feature (`x`) and label (`y`)
-`Tensor`s into the model for both training (`train_input_fn`) and evaluation
-(`test_input_fn`). To learn more about input functions, see the tutorial
-@{$input_fn$Building Input Functions with tf.estimator}.
-
-Then run the code. You should see output like the following:
-
-```none
-...
-INFO:tensorflow:loss = 4.86658, step = 4701
-INFO:tensorflow:loss = 4.86191, step = 4801
-INFO:tensorflow:loss = 4.85788, step = 4901
-...
-INFO:tensorflow:Saving evaluation summary for 5000 step: loss = 5.581
-Loss: 5.581
-```
-
-The loss score reported is the mean squared error returned from the `model_fn`
-when run on the `ABALONE_TEST` data set.
-
-To predict ages for the `ABALONE_PREDICT` data set, add the following to
-`main()`:
-
-```python
-# Print out predictions
-predict_input_fn = tf.estimator.inputs.numpy_input_fn(
- x={"x": prediction_set.data},
- num_epochs=1,
- shuffle=False)
-predictions = nn.predict(input_fn=predict_input_fn)
-for i, p in enumerate(predictions):
- print("Prediction %s: %s" % (i + 1, p["ages"]))
-```
-
-Here, the `predict()` function returns results in `predictions` as an iterable.
-The `for` loop enumerates and prints out the results. Rerun the code, and you
-should see output similar to the following:
-
-```python
-...
-Prediction 1: 4.92229
-Prediction 2: 10.3225
-Prediction 3: 7.384
-Prediction 4: 10.6264
-Prediction 5: 11.0862
-Prediction 6: 9.39239
-Prediction 7: 11.1289
-```
-
-## Additional Resources
-
-Congrats! You've successfully built a tf.estimator `Estimator` from scratch.
-For additional reference materials on building `Estimator`s, see the following
-sections of the API guides:
-
-* @{$python/contrib.layers$Layers}
-* @{tf.losses$Losses}
-* @{$python/contrib.layers#optimization$Optimization}
add support for your own shared or distributed filesystem.
* @{$new_data_formats$Custom Data Readers}, which details how to add support
for your own file and record formats.
- * @{$extend/estimators$Creating Estimators in tf.contrib.learn}, which explains how
- to write your own custom Estimator. For example, you could build your
- own Estimator to implement some variation on standard linear regression.
Python is currently the only language supported by TensorFlow's API stability
promises. However, TensorFlow also provides functionality in C++, Java, and Go,
adding_an_op.md
add_filesys.md
new_data_formats.md
-estimators.md
language_bindings.md
tool_developers/index.md
# Creating Custom Estimators
+
This document introduces custom Estimators. In particular, this document
demonstrates how to create a custom @{tf.estimator.Estimator$Estimator} that
mimics the behavior of the pre-made Estimator
```
If you are feeling impatient, feel free to compare and contrast
-[`custom_estimatr.py`](https://github.com/tensorflow/models/blob/master/samples/core/get_started/custom_estimator.py)
+[`custom_estimator.py`](https://github.com/tensorflow/models/blob/master/samples/core/get_started/custom_estimator.py)
with
-[`premade_estimatr.py`](https://github.com/tensorflow/models/blob/master/samples/core/get_started/premade_estimator.py).
+[`premade_estimator.py`](https://github.com/tensorflow/models/blob/master/samples/core/get_started/premade_estimator.py).
(which is in the same directory).
## Create feature columns
-As detailed in the @{$get_started/estimator$Premade Estimators} and
+As detailed in the @{$get_started/premade_estimators$Premade Estimators} and
@{$get_started/feature_columns$Feature Columns} chapters, you must define
your model's feature columns to specify how the model should use each feature.
Whether working with pre-made Estimators or custom Estimators, you define
In the simplest cases, @{tf.data.Dataset.from_tensor_slices} function takes an
array and returns a @{tf.data.Dataset} representing slices of the array. For
-example, an array containing the @{$mnist/beginners$mnist training data}
+example, an array containing the @{$tutorials/layers$mnist training data}
has a shape of `(60000, 28, 28)`. Passing this to `from_tensor_slices` returns
a `Dataset` object containing 60000 slices, each one a 28x28 image.
The result is a structure of @{$programmers_guide/tensors$TensorFlow tensors},
matching the layout of the items in the `Dataset`.
For an introduction to what these objects are and how to work with them,
-see @{$get_started/get_started}.
+see @{$programmers_guide/low_level_intro}.
``` python
print((features_result, labels_result))
+++ /dev/null
-# tf.estimator Quickstart
-
-TensorFlow’s high-level machine learning API (tf.estimator) makes it easy to
-configure, train, and evaluate a variety of machine learning models. In this
-tutorial, you’ll use tf.estimator to construct a
-[neural network](https://en.wikipedia.org/wiki/Artificial_neural_network)
-classifier and train it on the
-[Iris data set](https://en.wikipedia.org/wiki/Iris_flower_data_set) to
-predict flower species based on sepal/petal geometry. You'll write code to
-perform the following five steps:
-
-1. Load CSVs containing Iris training/test data into a TensorFlow `Dataset`
-2. Construct a @{tf.estimator.DNNClassifier$neural network classifier}
-3. Train the model using the training data
-4. Evaluate the accuracy of the model
-5. Classify new samples
-
-NOTE: Remember to @{$install$install TensorFlow on your machine}
-before getting started with this tutorial.
-
-## Complete Neural Network Source Code
-
-Here is the full code for the neural network classifier:
-
-```python
-from __future__ import absolute_import
-from __future__ import division
-from __future__ import print_function
-
-import os
-from six.moves.urllib.request import urlopen
-
-import numpy as np
-import tensorflow as tf
-
-# Data sets
-IRIS_TRAINING = "iris_training.csv"
-IRIS_TRAINING_URL = "http://download.tensorflow.org/data/iris_training.csv"
-
-IRIS_TEST = "iris_test.csv"
-IRIS_TEST_URL = "http://download.tensorflow.org/data/iris_test.csv"
-
-
-def main():
- # If the training and test sets aren't stored locally, download them.
- if not os.path.exists(IRIS_TRAINING):
- raw = urlopen(IRIS_TRAINING_URL).read()
- with open(IRIS_TRAINING, "wb") as f:
- f.write(raw)
-
- if not os.path.exists(IRIS_TEST):
- raw = urlopen(IRIS_TEST_URL).read()
- with open(IRIS_TEST, "wb") as f:
- f.write(raw)
-
- # Load datasets.
- training_set = tf.contrib.learn.datasets.base.load_csv_with_header(
- filename=IRIS_TRAINING,
- target_dtype=np.int,
- features_dtype=np.float32)
- test_set = tf.contrib.learn.datasets.base.load_csv_with_header(
- filename=IRIS_TEST,
- target_dtype=np.int,
- features_dtype=np.float32)
-
- # Specify that all features have real-value data
- feature_columns = [tf.feature_column.numeric_column("x", shape=[4])]
-
- # Build 3 layer DNN with 10, 20, 10 units respectively.
- classifier = tf.estimator.DNNClassifier(feature_columns=feature_columns,
- hidden_units=[10, 20, 10],
- n_classes=3,
- model_dir="/tmp/iris_model")
- # Define the training inputs
- train_input_fn = tf.estimator.inputs.numpy_input_fn(
- x={"x": np.array(training_set.data)},
- y=np.array(training_set.target),
- num_epochs=None,
- shuffle=True)
-
- # Train model.
- classifier.train(input_fn=train_input_fn, steps=2000)
-
- # Define the test inputs
- test_input_fn = tf.estimator.inputs.numpy_input_fn(
- x={"x": np.array(test_set.data)},
- y=np.array(test_set.target),
- num_epochs=1,
- shuffle=False)
-
- # Evaluate accuracy.
- accuracy_score = classifier.evaluate(input_fn=test_input_fn)["accuracy"]
-
- print("\nTest Accuracy: {0:f}\n".format(accuracy_score))
-
- # Classify two new flower samples.
- new_samples = np.array(
- [[6.4, 3.2, 4.5, 1.5],
- [5.8, 3.1, 5.0, 1.7]], dtype=np.float32)
- predict_input_fn = tf.estimator.inputs.numpy_input_fn(
- x={"x": new_samples},
- num_epochs=1,
- shuffle=False)
-
- predictions = list(classifier.predict(input_fn=predict_input_fn))
- predicted_classes = [p["classes"] for p in predictions]
-
- print(
- "New Samples, Class Predictions: {}\n"
- .format(predicted_classes))
-
-if __name__ == "__main__":
- main()
-```
-
-The following sections walk through the code in detail.
-
-## Load the Iris CSV data to TensorFlow
-
-The [Iris data set](https://en.wikipedia.org/wiki/Iris_flower_data_set) contains
-150 rows of data, comprising 50 samples from each of three related Iris species:
-*Iris setosa*, *Iris virginica*, and *Iris versicolor*.
-
-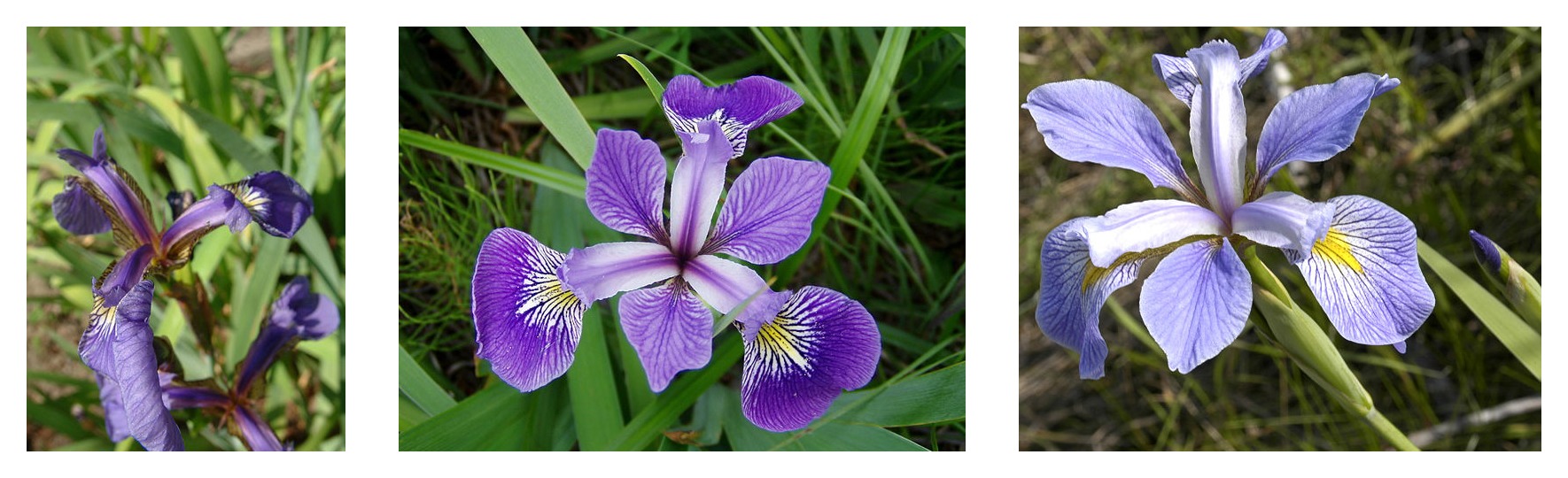 **From left to right,
-[*Iris setosa*](https://commons.wikimedia.org/w/index.php?curid=170298) (by
-[Radomil](https://commons.wikimedia.org/wiki/User:Radomil), CC BY-SA 3.0),
-[*Iris versicolor*](https://commons.wikimedia.org/w/index.php?curid=248095) (by
-[Dlanglois](https://commons.wikimedia.org/wiki/User:Dlanglois), CC BY-SA 3.0),
-and [*Iris virginica*](https://www.flickr.com/photos/33397993@N05/3352169862)
-(by [Frank Mayfield](https://www.flickr.com/photos/33397993@N05), CC BY-SA
-2.0).**
-
-Each row contains the following data for each flower sample:
-[sepal](https://en.wikipedia.org/wiki/Sepal) length, sepal width,
-[petal](https://en.wikipedia.org/wiki/Petal) length, petal width, and flower
-species. Flower species are represented as integers, with 0 denoting *Iris
-setosa*, 1 denoting *Iris versicolor*, and 2 denoting *Iris virginica*.
-
-Sepal Length | Sepal Width | Petal Length | Petal Width | Species
-:----------- | :---------- | :----------- | :---------- | :-------
-5.1 | 3.5 | 1.4 | 0.2 | 0
-4.9 | 3.0 | 1.4 | 0.2 | 0
-4.7 | 3.2 | 1.3 | 0.2 | 0
-… | … | … | … | …
-7.0 | 3.2 | 4.7 | 1.4 | 1
-6.4 | 3.2 | 4.5 | 1.5 | 1
-6.9 | 3.1 | 4.9 | 1.5 | 1
-… | … | … | … | …
-6.5 | 3.0 | 5.2 | 2.0 | 2
-6.2 | 3.4 | 5.4 | 2.3 | 2
-5.9 | 3.0 | 5.1 | 1.8 | 2
-
-For this tutorial, the Iris data has been randomized and split into two separate
-CSVs:
-
-* A training set of 120 samples
- ([iris_training.csv](http://download.tensorflow.org/data/iris_training.csv))
-* A test set of 30 samples
- ([iris_test.csv](http://download.tensorflow.org/data/iris_test.csv)).
-
-To get started, first import all the necessary modules, and define where to
-download and store the dataset:
-
-```python
-from __future__ import absolute_import
-from __future__ import division
-from __future__ import print_function
-
-import os
-from six.moves.urllib.request import urlopen
-
-import tensorflow as tf
-import numpy as np
-
-IRIS_TRAINING = "iris_training.csv"
-IRIS_TRAINING_URL = "http://download.tensorflow.org/data/iris_training.csv"
-
-IRIS_TEST = "iris_test.csv"
-IRIS_TEST_URL = "http://download.tensorflow.org/data/iris_test.csv"
-```
-
-Then, if the training and test sets aren't already stored locally, download
-them.
-
-```python
-if not os.path.exists(IRIS_TRAINING):
- raw = urlopen(IRIS_TRAINING_URL).read()
- with open(IRIS_TRAINING,'wb') as f:
- f.write(raw)
-
-if not os.path.exists(IRIS_TEST):
- raw = urlopen(IRIS_TEST_URL).read()
- with open(IRIS_TEST,'wb') as f:
- f.write(raw)
-```
-
-Next, load the training and test sets into `Dataset`s using the
-[`load_csv_with_header()`](https://www.tensorflow.org/code/tensorflow/contrib/learn/python/learn/datasets/base.py)
-method in `learn.datasets.base`. The `load_csv_with_header()` method takes three
-required arguments:
-
-* `filename`, which takes the filepath to the CSV file
-* `target_dtype`, which takes the
- [`numpy` datatype](http://docs.scipy.org/doc/numpy/user/basics.types.html)
- of the dataset's target value.
-* `features_dtype`, which takes the
- [`numpy` datatype](http://docs.scipy.org/doc/numpy/user/basics.types.html)
- of the dataset's feature values.
-
-
-Here, the target (the value you're training the model to predict) is flower
-species, which is an integer from 0–2, so the appropriate `numpy` datatype
-is `np.int`:
-
-```python
-# Load datasets.
-training_set = tf.contrib.learn.datasets.base.load_csv_with_header(
- filename=IRIS_TRAINING,
- target_dtype=np.int,
- features_dtype=np.float32)
-test_set = tf.contrib.learn.datasets.base.load_csv_with_header(
- filename=IRIS_TEST,
- target_dtype=np.int,
- features_dtype=np.float32)
-```
-
-`Dataset`s in tf.contrib.learn are
-[named tuples](https://docs.python.org/2/library/collections.html#collections.namedtuple);
-you can access feature data and target values via the `data` and `target`
-fields. Here, `training_set.data` and `training_set.target` contain the feature
-data and target values for the training set, respectively, and `test_set.data`
-and `test_set.target` contain feature data and target values for the test set.
-
-Later on, in
-["Fit the DNNClassifier to the Iris Training Data,"](#fit_the_dnnclassifier_to_the_iris_training_data)
-you'll use `training_set.data` and
-`training_set.target` to train your model, and in
-["Evaluate Model Accuracy,"](#evaluate_model_accuracy) you'll use `test_set.data` and
-`test_set.target`. But first, you'll construct your model in the next section.
-
-## Construct a Deep Neural Network Classifier
-
-tf.estimator offers a variety of predefined models, called `Estimator`s, which
-you can use "out of the box" to run training and evaluation operations on your
-data.
-Here, you'll configure a Deep Neural Network Classifier model to fit the Iris
-data. Using tf.estimator, you can instantiate your
-@{tf.estimator.DNNClassifier} with just a couple lines of code:
-
-```python
-# Specify that all features have real-value data
-feature_columns = [tf.feature_column.numeric_column("x", shape=[4])]
-
-# Build 3 layer DNN with 10, 20, 10 units respectively.
-classifier = tf.estimator.DNNClassifier(feature_columns=feature_columns,
- hidden_units=[10, 20, 10],
- n_classes=3,
- model_dir="/tmp/iris_model")
-```
-
-The code above first defines the model's feature columns, which specify the data
-type for the features in the data set. All the feature data is continuous, so
-`tf.feature_column.numeric_column` is the appropriate function to use to
-construct the feature columns. There are four features in the data set (sepal
-width, sepal height, petal width, and petal height), so accordingly `shape`
-must be set to `[4]` to hold all the data.
-
-Then, the code creates a `DNNClassifier` model using the following arguments:
-
-* `feature_columns=feature_columns`. The set of feature columns defined above.
-* `hidden_units=[10, 20, 10]`. Three
- [hidden layers](http://stats.stackexchange.com/questions/181/how-to-choose-the-number-of-hidden-layers-and-nodes-in-a-feedforward-neural-netw),
- containing 10, 20, and 10 neurons, respectively.
-* `n_classes=3`. Three target classes, representing the three Iris species.
-* `model_dir=/tmp/iris_model`. The directory in which TensorFlow will save
- checkpoint data and TensorBoard summaries during model training.
-
-## Describe the training input pipeline {#train-input}
-
-The `tf.estimator` API uses input functions, which create the TensorFlow
-operations that generate data for the model.
-We can use `tf.estimator.inputs.numpy_input_fn` to produce the input pipeline:
-
-```python
-# Define the training inputs
-train_input_fn = tf.estimator.inputs.numpy_input_fn(
- x={"x": np.array(training_set.data)},
- y=np.array(training_set.target),
- num_epochs=None,
- shuffle=True)
-```
-
-## Fit the DNNClassifier to the Iris Training Data {#fit-dnnclassifier}
-
-Now that you've configured your DNN `classifier` model, you can fit it to the
-Iris training data using the @{tf.estimator.Estimator.train$`train`} method.
-Pass `train_input_fn` as the `input_fn`, and the number of steps to train
-(here, 2000):
-
-```python
-# Train model.
-classifier.train(input_fn=train_input_fn, steps=2000)
-```
-
-The state of the model is preserved in the `classifier`, which means you can
-train iteratively if you like. For example, the above is equivalent to the
-following:
-
-```python
-classifier.train(input_fn=train_input_fn, steps=1000)
-classifier.train(input_fn=train_input_fn, steps=1000)
-```
-
-However, if you're looking to track the model while it trains, you'll likely
-want to instead use a TensorFlow @{tf.train.SessionRunHook$`SessionRunHook`}
-to perform logging operations.
-
-## Evaluate Model Accuracy {#evaluate-accuracy}
-
-You've trained your `DNNClassifier` model on the Iris training data; now, you
-can check its accuracy on the Iris test data using the
-@{tf.estimator.Estimator.evaluate$`evaluate`} method. Like `train`,
-`evaluate` takes an input function that builds its input pipeline. `evaluate`
-returns a `dict`s with the evaluation results. The following code passes the
-Iris test data—`test_set.data` and `test_set.target`—to `evaluate`
-and prints the `accuracy` from the results:
-
-```python
-# Define the test inputs
-test_input_fn = tf.estimator.inputs.numpy_input_fn(
- x={"x": np.array(test_set.data)},
- y=np.array(test_set.target),
- num_epochs=1,
- shuffle=False)
-
-# Evaluate accuracy.
-accuracy_score = classifier.evaluate(input_fn=test_input_fn)["accuracy"]
-
-print("\nTest Accuracy: {0:f}\n".format(accuracy_score))
-```
-
-Note: The `num_epochs=1` argument to `numpy_input_fn` is important here.
-`test_input_fn` will iterate over the data once, and then raise
-`OutOfRangeError`. This error signals the classifier to stop evaluating, so it
-will evaluate over the input once.
-
-When you run the full script, it will print something close to:
-
-```
-Test Accuracy: 0.966667
-```
-
-Your accuracy result may vary a bit, but should be higher than 90%. Not bad for
-a relatively small data set!
-
-## Classify New Samples
-
-Use the estimator's `predict()` method to classify new samples. For example, say
-you have these two new flower samples:
-
-Sepal Length | Sepal Width | Petal Length | Petal Width
-:----------- | :---------- | :----------- | :----------
-6.4 | 3.2 | 4.5 | 1.5
-5.8 | 3.1 | 5.0 | 1.7
-
-You can predict their species using the `predict()` method. `predict` returns a
-generator of dicts, which can easily be converted to a list. The following code
-retrieves and prints the class predictions:
-
-```python
-# Classify two new flower samples.
-new_samples = np.array(
- [[6.4, 3.2, 4.5, 1.5],
- [5.8, 3.1, 5.0, 1.7]], dtype=np.float32)
-predict_input_fn = tf.estimator.inputs.numpy_input_fn(
- x={"x": new_samples},
- num_epochs=1,
- shuffle=False)
-
-predictions = list(classifier.predict(input_fn=predict_input_fn))
-predicted_classes = [p["classes"] for p in predictions]
-
-print(
- "New Samples, Class Predictions: {}\n"
- .format(predicted_classes))
-```
-
-Your results should look as follows:
-
-```
-New Samples, Class Predictions: [1 2]
-```
-
-The model thus predicts that the first sample is *Iris versicolor*, and the
-second sample is *Iris virginica*.
-
-## Additional Resources
-
-* To learn more about using tf.estimator to create linear models, see
- @{$linear$Large-scale Linear Models with TensorFlow}.
-
-* To build your own Estimator using tf.estimator APIs, check out
- @{$extend/estimators$Creating Estimators}.
-
-* To experiment with neural network modeling and visualization in the browser,
- check out [Deep Playground](http://playground.tensorflow.org/).
-
-* For more advanced tutorials on neural networks, see
- @{$deep_cnn$Convolutional Neural Networks} and @{$recurrent$Recurrent Neural
- Networks}.
enabling you to transform a diverse range of raw data into formats that
Estimators can use, allowing easy experimentation.
-In @{$get_started/estimator$Premade Estimators}, we used the premade Estimator,
-@{tf.estimator.DNNClassifier$`DNNClassifier`} to train a model to predict
-different types of Iris flowers from four input features. That example created
-only numerical feature columns (of type @{tf.feature_column.numeric_column}).
-Although numerical feature columns model the lengths of petals and sepals
-effectively, real world data sets contain all kinds of features, many of which
-are non-numerical.
+In @{$get_started/premade_estimators$Premade Estimators}, we used the premade
+Estimator, @{tf.estimator.DNNClassifier$`DNNClassifier`} to train a model to
+predict different types of Iris flowers from four input features. That example
+created only numerical feature columns (of type
+@{tf.feature_column.numeric_column}). Although numerical feature columns model
+the lengths of petals and sepals effectively, real world data sets contain all
+kinds of features, many of which are non-numerical.
<div style="width:80%; margin:auto; margin-bottom:10px; margin-top:20px;">
<img style="width:100%" src="../images/feature_columns/feature_cloud.jpg">
+++ /dev/null
-# Getting Started With TensorFlow
-
-This guide gets you started programming in TensorFlow. Before using this guide,
-@{$install$install TensorFlow}. To get the most out of
-this guide, you should know the following:
-
-* How to program in Python.
-* At least a little bit about arrays.
-* Ideally, something about machine learning. However, if you know little or
- nothing about machine learning, then this is still the first guide you
- should read.
-
-TensorFlow provides multiple APIs. The lowest level API--TensorFlow Core--
-provides you with complete programming control. We recommend TensorFlow Core for
-machine learning researchers and others who require fine levels of control over
-their models. The higher level APIs are built on top of TensorFlow Core. These
-higher level APIs are typically easier to learn and use than TensorFlow Core. In
-addition, the higher level APIs make repetitive tasks easier and more consistent
-between different users. A high-level API like tf.estimator helps you manage
-data sets, estimators, training and inference.
-
-This guide begins with a tutorial on TensorFlow Core. Later, we
-demonstrate how to implement the same model in tf.estimator. Knowing
-TensorFlow Core principles will give you a great mental model of how things are
-working internally when you use the more compact higher level API.
-
-# Tensors
-
-The central unit of data in TensorFlow is the **tensor**. A tensor consists of a
-set of primitive values shaped into an array of any number of dimensions. A
-tensor's **rank** is its number of dimensions. Here are some examples of
-tensors:
-
-```python
-3 # a rank 0 tensor; a scalar with shape []
-[1., 2., 3.] # a rank 1 tensor; a vector with shape [3]
-[[1., 2., 3.], [4., 5., 6.]] # a rank 2 tensor; a matrix with shape [2, 3]
-[[[1., 2., 3.]], [[7., 8., 9.]]] # a rank 3 tensor with shape [2, 1, 3]
-```
-
-## TensorFlow Core tutorial
-
-### Importing TensorFlow
-
-The canonical import statement for TensorFlow programs is as follows:
-
-```python
-import tensorflow as tf
-```
-This gives Python access to all of TensorFlow's classes, methods, and symbols.
-Most of the documentation assumes you have already done this.
-
-### The Computational Graph
-
-You might think of TensorFlow Core programs as consisting of two discrete
-sections:
-
-1. Building the computational graph.
-2. Running the computational graph.
-
-A **computational graph** is a series of TensorFlow operations arranged into a
-graph of nodes.
-Let's build a simple computational graph. Each node takes zero
-or more tensors as inputs and produces a tensor as an output. One type of node
-is a constant. Like all TensorFlow constants, it takes no inputs, and it outputs
-a value it stores internally. We can create two floating point Tensors `node1`
-and `node2` as follows:
-
-```python
-node1 = tf.constant(3.0, dtype=tf.float32)
-node2 = tf.constant(4.0) # also tf.float32 implicitly
-print(node1, node2)
-```
-
-The final print statement produces
-
-```
-Tensor("Const:0", shape=(), dtype=float32) Tensor("Const_1:0", shape=(), dtype=float32)
-```
-
-Notice that printing the nodes does not output the values `3.0` and `4.0` as you
-might expect. Instead, they are nodes that, when evaluated, would produce 3.0
-and 4.0, respectively. To actually evaluate the nodes, we must run the
-computational graph within a **session**. A session encapsulates the control and
-state of the TensorFlow runtime.
-
-The following code creates a `Session` object and then invokes its `run` method
-to run enough of the computational graph to evaluate `node1` and `node2`. By
-running the computational graph in a session as follows:
-
-```python
-sess = tf.Session()
-print(sess.run([node1, node2]))
-```
-
-we see the expected values of 3.0 and 4.0:
-
-```
-[3.0, 4.0]
-```
-
-We can build more complicated computations by combining `Tensor` nodes with
-operations (Operations are also nodes). For example, we can add our two
-constant nodes and produce a new graph as follows:
-
-```python
-from __future__ import print_function
-node3 = tf.add(node1, node2)
-print("node3:", node3)
-print("sess.run(node3):", sess.run(node3))
-```
-
-The last two print statements produce
-
-```
-node3: Tensor("Add:0", shape=(), dtype=float32)
-sess.run(node3): 7.0
-```
-
-TensorFlow provides a utility called TensorBoard that can display a picture of
-the computational graph. Here is a screenshot showing how TensorBoard
-visualizes the graph:
-
-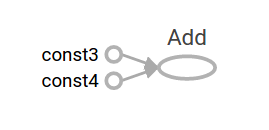
-
-As it stands, this graph is not especially interesting because it always
-produces a constant result. A graph can be parameterized to accept external
-inputs, known as **placeholders**. A **placeholder** is a promise to provide a
-value later.
-
-```python
-a = tf.placeholder(tf.float32)
-b = tf.placeholder(tf.float32)
-adder_node = a + b # + provides a shortcut for tf.add(a, b)
-```
-
-The preceding three lines are a bit like a function or a lambda in which we
-define two input parameters (a and b) and then an operation on them. We can
-evaluate this graph with multiple inputs by using the feed_dict argument to
-the [run method](https://www.tensorflow.org/api_docs/python/tf/Session#run)
-to feed concrete values to the placeholders:
-
-```python
-print(sess.run(adder_node, {a: 3, b: 4.5}))
-print(sess.run(adder_node, {a: [1, 3], b: [2, 4]}))
-```
-resulting in the output
-
-```
-7.5
-[ 3. 7.]
-```
-
-In TensorBoard, the graph looks like this:
-
-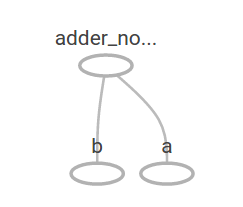
-
-We can make the computational graph more complex by adding another operation.
-For example,
-
-```python
-add_and_triple = adder_node * 3.
-print(sess.run(add_and_triple, {a: 3, b: 4.5}))
-```
-produces the output
-```
-22.5
-```
-
-The preceding computational graph would look as follows in TensorBoard:
-
-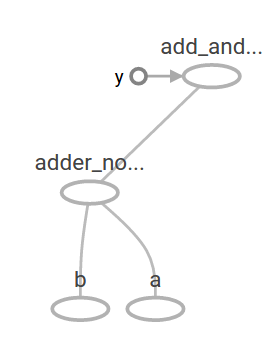
-
-In machine learning we will typically want a model that can take arbitrary
-inputs, such as the one above. To make the model trainable, we need to be able
-to modify the graph to get new outputs with the same input. **Variables** allow
-us to add trainable parameters to a graph. They are constructed with a type and
-initial value:
-
-
-```python
-W = tf.Variable([.3], dtype=tf.float32)
-b = tf.Variable([-.3], dtype=tf.float32)
-x = tf.placeholder(tf.float32)
-linear_model = W*x + b
-```
-
-Constants are initialized when you call `tf.constant`, and their value can never
-change. By contrast, variables are not initialized when you call `tf.Variable`.
-To initialize all the variables in a TensorFlow program, you must explicitly
-call a special operation as follows:
-
-```python
-init = tf.global_variables_initializer()
-sess.run(init)
-```
-It is important to realize `init` is a handle to the TensorFlow sub-graph that
-initializes all the global variables. Until we call `sess.run`, the variables
-are uninitialized.
-
-
-Since `x` is a placeholder, we can evaluate `linear_model` for several values of
-`x` simultaneously as follows:
-
-```python
-print(sess.run(linear_model, {x: [1, 2, 3, 4]}))
-```
-to produce the output
-```
-[ 0. 0.30000001 0.60000002 0.90000004]
-```
-
-We've created a model, but we don't know how good it is yet. To evaluate the
-model on training data, we need a `y` placeholder to provide the desired values,
-and we need to write a loss function.
-
-A loss function measures how far apart the
-current model is from the provided data. We'll use a standard loss model for
-linear regression, which sums the squares of the deltas between the current
-model and the provided data. `linear_model - y` creates a vector where each
-element is the corresponding example's error delta. We call `tf.square` to
-square that error. Then, we sum all the squared errors to create a single scalar
-that abstracts the error of all examples using `tf.reduce_sum`:
-
-```python
-y = tf.placeholder(tf.float32)
-squared_deltas = tf.square(linear_model - y)
-loss = tf.reduce_sum(squared_deltas)
-print(sess.run(loss, {x: [1, 2, 3, 4], y: [0, -1, -2, -3]}))
-```
-producing the loss value
-```
-23.66
-```
-
-We could improve this manually by reassigning the values of `W` and `b` to the
-perfect values of -1 and 1. A variable is initialized to the value provided to
-`tf.Variable` but can be changed using operations like `tf.assign`. For example,
-`W=-1` and `b=1` are the optimal parameters for our model. We can change `W` and
-`b` accordingly:
-
-```python
-fixW = tf.assign(W, [-1.])
-fixb = tf.assign(b, [1.])
-sess.run([fixW, fixb])
-print(sess.run(loss, {x: [1, 2, 3, 4], y: [0, -1, -2, -3]}))
-```
-The final print shows the loss now is zero.
-```
-0.0
-```
-
-We guessed the "perfect" values of `W` and `b`, but the whole point of machine
-learning is to find the correct model parameters automatically. We will show
-how to accomplish this in the next section.
-
-## tf.train API
-
-A complete discussion of machine learning is out of the scope of this tutorial.
-However, TensorFlow provides **optimizers** that slowly change each variable in
-order to minimize the loss function. The simplest optimizer is **gradient
-descent**. It modifies each variable according to the magnitude of the
-derivative of loss with respect to that variable. In general, computing symbolic
-derivatives manually is tedious and error-prone. Consequently, TensorFlow can
-automatically produce derivatives given only a description of the model using
-the function `tf.gradients`. For simplicity, optimizers typically do this
-for you. For example,
-
-```python
-optimizer = tf.train.GradientDescentOptimizer(0.01)
-train = optimizer.minimize(loss)
-```
-
-```python
-sess.run(init) # reset variables to incorrect defaults.
-for i in range(1000):
- sess.run(train, {x: [1, 2, 3, 4], y: [0, -1, -2, -3]})
-
-print(sess.run([W, b]))
-```
-results in the final model parameters:
-```
-[array([-0.9999969], dtype=float32), array([ 0.99999082], dtype=float32)]
-```
-
-Now we have done actual machine learning! Although this simple linear
-regression model does not require much TensorFlow core code, more complicated
-models and methods to feed data into your models necessitate more code. Thus,
-TensorFlow provides higher level abstractions for common patterns, structures,
-and functionality. We will learn how to use some of these abstractions in the
-next section.
-
-### Complete program
-
-The completed trainable linear regression model is shown here:
-
-```python
-import tensorflow as tf
-
-# Model parameters
-W = tf.Variable([.3], dtype=tf.float32)
-b = tf.Variable([-.3], dtype=tf.float32)
-# Model input and output
-x = tf.placeholder(tf.float32)
-linear_model = W*x + b
-y = tf.placeholder(tf.float32)
-
-# loss
-loss = tf.reduce_sum(tf.square(linear_model - y)) # sum of the squares
-# optimizer
-optimizer = tf.train.GradientDescentOptimizer(0.01)
-train = optimizer.minimize(loss)
-
-# training data
-x_train = [1, 2, 3, 4]
-y_train = [0, -1, -2, -3]
-# training loop
-init = tf.global_variables_initializer()
-sess = tf.Session()
-sess.run(init) # initialize variables with incorrect defaults.
-for i in range(1000):
- sess.run(train, {x: x_train, y: y_train})
-
-# evaluate training accuracy
-curr_W, curr_b, curr_loss = sess.run([W, b, loss], {x: x_train, y: y_train})
-print("W: %s b: %s loss: %s"%(curr_W, curr_b, curr_loss))
-```
-When run, it produces
-```
-W: [-0.9999969] b: [ 0.99999082] loss: 5.69997e-11
-```
-
-Notice that the loss is a very small number (very close to zero). If you run
-this program, your loss may not be exactly the same as the aforementioned loss
-because the model is initialized with pseudorandom values.
-
-This more complicated program can still be visualized in TensorBoard
-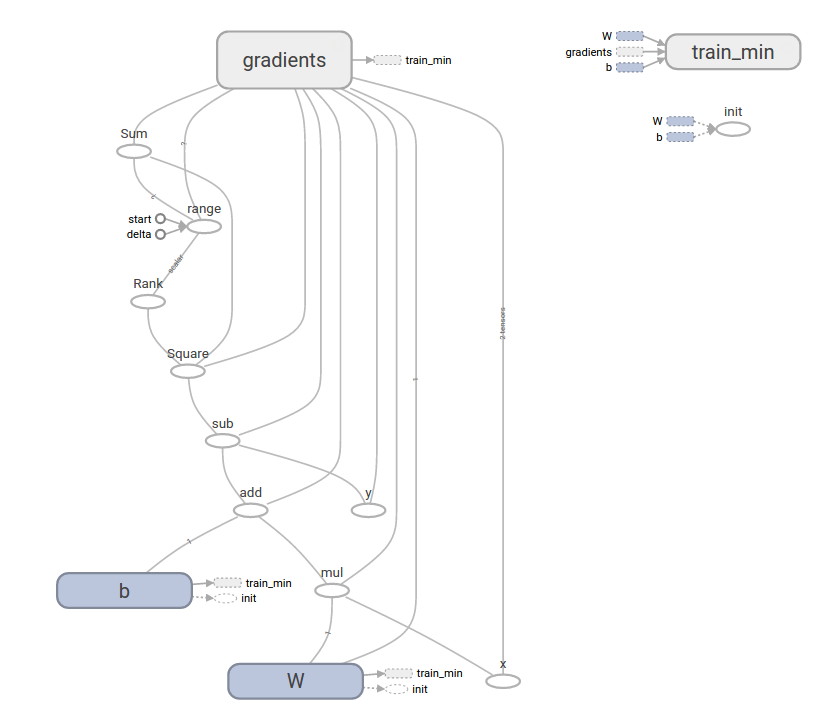
-
-## `tf.estimator`
-
-`tf.estimator` is a high-level TensorFlow library that simplifies the
-mechanics of machine learning, including the following:
-
-* running training loops
-* running evaluation loops
-* managing data sets
-
-tf.estimator defines many common models.
-
-### Basic usage
-
-Notice how much simpler the linear regression program becomes with
-`tf.estimator`:
-
-```python
-# NumPy is often used to load, manipulate and preprocess data.
-import numpy as np
-import tensorflow as tf
-
-# Declare list of features. We only have one numeric feature. There are many
-# other types of columns that are more complicated and useful.
-feature_columns = [tf.feature_column.numeric_column("x", shape=[1])]
-
-# An estimator is the front end to invoke training (fitting) and evaluation
-# (inference). There are many predefined types like linear regression,
-# linear classification, and many neural network classifiers and regressors.
-# The following code provides an estimator that does linear regression.
-estimator = tf.estimator.LinearRegressor(feature_columns=feature_columns)
-
-# TensorFlow provides many helper methods to read and set up data sets.
-# Here we use two data sets: one for training and one for evaluation
-# We have to tell the function how many batches
-# of data (num_epochs) we want and how big each batch should be.
-x_train = np.array([1., 2., 3., 4.])
-y_train = np.array([0., -1., -2., -3.])
-x_eval = np.array([2., 5., 8., 1.])
-y_eval = np.array([-1.01, -4.1, -7., 0.])
-input_fn = tf.estimator.inputs.numpy_input_fn(
- {"x": x_train}, y_train, batch_size=4, num_epochs=None, shuffle=True)
-train_input_fn = tf.estimator.inputs.numpy_input_fn(
- {"x": x_train}, y_train, batch_size=4, num_epochs=1000, shuffle=False)
-eval_input_fn = tf.estimator.inputs.numpy_input_fn(
- {"x": x_eval}, y_eval, batch_size=4, num_epochs=1000, shuffle=False)
-
-# We can invoke 1000 training steps by invoking the method and passing the
-# training data set.
-estimator.train(input_fn=input_fn, steps=1000)
-
-# Here we evaluate how well our model did.
-train_metrics = estimator.evaluate(input_fn=train_input_fn)
-eval_metrics = estimator.evaluate(input_fn=eval_input_fn)
-print("train metrics: %r"% train_metrics)
-print("eval metrics: %r"% eval_metrics)
-```
-When run, it produces something like
-```
-train metrics: {'average_loss': 1.4833182e-08, 'global_step': 1000, 'loss': 5.9332727e-08}
-eval metrics: {'average_loss': 0.0025353201, 'global_step': 1000, 'loss': 0.01014128}
-```
-Notice how our eval data has a higher loss, but it is still close to zero.
-That means we are learning properly.
-
-### A custom model
-
-`tf.estimator` does not lock you into its predefined models. Suppose we
-wanted to create a custom model that is not built into TensorFlow. We can still
-retain the high level abstraction of data set, feeding, training, etc. of
-`tf.estimator`. For illustration, we will show how to implement our own
-equivalent model to `LinearRegressor` using our knowledge of the lower level
-TensorFlow API.
-
-To define a custom model that works with `tf.estimator`, we need to use
-`tf.estimator.Estimator`. `tf.estimator.LinearRegressor` is actually
-a sub-class of `tf.estimator.Estimator`. Instead of sub-classing
-`Estimator`, we simply provide `Estimator` a function `model_fn` that tells
-`tf.estimator` how it can evaluate predictions, training steps, and
-loss. The code is as follows:
-
-```python
-import numpy as np
-import tensorflow as tf
-
-# Declare list of features, we only have one real-valued feature
-def model_fn(features, labels, mode):
- # Build a linear model and predict values
- W = tf.get_variable("W", [1], dtype=tf.float64)
- b = tf.get_variable("b", [1], dtype=tf.float64)
- y = W*features['x'] + b
- # Loss sub-graph
- loss = tf.reduce_sum(tf.square(y - labels))
- # Training sub-graph
- global_step = tf.train.get_global_step()
- optimizer = tf.train.GradientDescentOptimizer(0.01)
- train = tf.group(optimizer.minimize(loss),
- tf.assign_add(global_step, 1))
- # EstimatorSpec connects subgraphs we built to the
- # appropriate functionality.
- return tf.estimator.EstimatorSpec(
- mode=mode,
- predictions=y,
- loss=loss,
- train_op=train)
-
-estimator = tf.estimator.Estimator(model_fn=model_fn)
-# define our data sets
-x_train = np.array([1., 2., 3., 4.])
-y_train = np.array([0., -1., -2., -3.])
-x_eval = np.array([2., 5., 8., 1.])
-y_eval = np.array([-1.01, -4.1, -7., 0.])
-input_fn = tf.estimator.inputs.numpy_input_fn(
- {"x": x_train}, y_train, batch_size=4, num_epochs=None, shuffle=True)
-train_input_fn = tf.estimator.inputs.numpy_input_fn(
- {"x": x_train}, y_train, batch_size=4, num_epochs=1000, shuffle=False)
-eval_input_fn = tf.estimator.inputs.numpy_input_fn(
- {"x": x_eval}, y_eval, batch_size=4, num_epochs=1, shuffle=False)
-
-# train
-estimator.train(input_fn=input_fn, steps=1000)
-# Here we evaluate how well our model did.
-train_metrics = estimator.evaluate(input_fn=train_input_fn)
-eval_metrics = estimator.evaluate(input_fn=eval_input_fn)
-print("train metrics: %r"% train_metrics)
-print("eval metrics: %r"% eval_metrics)
-```
-When run, it produces
-```
-train metrics: {'loss': 1.227995e-11, 'global_step': 1000}
-eval metrics: {'loss': 0.01010036, 'global_step': 1000}
-```
-
-Notice how the contents of the custom `model_fn()` function are very similar
-to our manual model training loop from the lower level API.
-
-## Next steps
-
-Now you have a working knowledge of the basics of TensorFlow. We have several
-more tutorials that you can look at to learn more. If you are a beginner in
-machine learning see @{$beginners$MNIST for beginners},
-otherwise see @{$pros$Deep MNIST for experts}.
+++ /dev/null
-# TensorBoard: Graph Visualization
-
-TensorFlow computation graphs are powerful but complicated. The graph visualization can help you understand and debug them. Here's an example of the visualization at work.
-
-
-*Visualization of a TensorFlow graph.*
-
-To see your own graph, run TensorBoard pointing it to the log directory of the job, click on the graph tab on the top pane and select the appropriate run using the menu at the upper left corner. For in depth information on how to run TensorBoard and make sure you are logging all the necessary information, see @{$summaries_and_tensorboard$TensorBoard: Visualizing Learning}.
-
-## Name scoping and nodes
-
-Typical TensorFlow graphs can have many thousands of nodes--far too many to see
-easily all at once, or even to lay out using standard graph tools. To simplify,
-variable names can be scoped and the visualization uses this information to
-define a hierarchy on the nodes in the graph. By default, only the top of this
-hierarchy is shown. Here is an example that defines three operations under the
-`hidden` name scope using
-@{tf.name_scope}:
-
-```python
-import tensorflow as tf
-
-with tf.name_scope('hidden') as scope:
- a = tf.constant(5, name='alpha')
- W = tf.Variable(tf.random_uniform([1, 2], -1.0, 1.0), name='weights')
- b = tf.Variable(tf.zeros([1]), name='biases')
-```
-
-This results in the following three op names:
-
-* `hidden/alpha`
-* `hidden/weights`
-* `hidden/biases`
-
-By default, the visualization will collapse all three into a node labeled `hidden`.
-The extra detail isn't lost. You can double-click, or click
-on the orange `+` sign in the top right to expand the node, and then you'll see
-three subnodes for `alpha`, `weights` and `biases`.
-
-Here's a real-life example of a more complicated node in its initial and
-expanded states.
-
-<table width="100%;">
- <tr>
- <td style="width: 50%;">
- <img src="https://www.tensorflow.org/images/pool1_collapsed.png" alt="Unexpanded name scope" title="Unexpanded name scope" />
- </td>
- <td style="width: 50%;">
- <img src="https://www.tensorflow.org/images/pool1_expanded.png" alt="Expanded name scope" title="Expanded name scope" />
- </td>
- </tr>
- <tr>
- <td style="width: 50%;">
- Initial view of top-level name scope <code>pool_1</code>. Clicking on the orange <code>+</code> button on the top right or double-clicking on the node itself will expand it.
- </td>
- <td style="width: 50%;">
- Expanded view of <code>pool_1</code> name scope. Clicking on the orange <code>-</code> button on the top right or double-clicking on the node itself will collapse the name scope.
- </td>
- </tr>
-</table>
-
-Grouping nodes by name scopes is critical to making a legible graph. If you're
-building a model, name scopes give you control over the resulting visualization.
-**The better your name scopes, the better your visualization.**
-
-The figure above illustrates a second aspect of the visualization. TensorFlow
-graphs have two kinds of connections: data dependencies and control
-dependencies. Data dependencies show the flow of tensors between two ops and
-are shown as solid arrows, while control dependencies use dotted lines. In the
-expanded view (right side of the figure above) all the connections are data
-dependencies with the exception of the dotted line connecting `CheckNumerics`
-and `control_dependency`.
-
-There's a second trick to simplifying the layout. Most TensorFlow graphs have a
-few nodes with many connections to other nodes. For example, many nodes might
-have a control dependency on an initialization step. Drawing all edges between
-the `init` node and its dependencies would create a very cluttered view.
-
-To reduce clutter, the visualization separates out all high-degree nodes to an
-*auxiliary* area on the right and doesn't draw lines to represent their edges.
-Instead of lines, we draw small *node icons* to indicate the connections.
-Separating out the auxiliary nodes typically doesn't remove critical
-information since these nodes are usually related to bookkeeping functions.
-See [Interaction](#interaction) for how to move nodes between the main graph
-and the auxiliary area.
-
-<table width="100%;">
- <tr>
- <td style="width: 50%;">
- <img src="https://www.tensorflow.org/images/conv_1.png" alt="conv_1 is part of the main graph" title="conv_1 is part of the main graph" />
- </td>
- <td style="width: 50%;">
- <img src="https://www.tensorflow.org/images/save.png" alt="save is extracted as auxiliary node" title="save is extracted as auxiliary node" />
- </td>
- </tr>
- <tr>
- <td style="width: 50%;">
- Node <code>conv_1</code> is connected to <code>save</code>. Note the little <code>save</code> node icon on its right.
- </td>
- <td style="width: 50%;">
- <code>save</code> has a high degree, and will appear as an auxiliary node. The connection with <code>conv_1</code> is shown as a node icon on its left. To further reduce clutter, since <code>save</code> has a lot of connections, we show the first 5 and abbreviate the others as <code>... 12 more</code>.
- </td>
- </tr>
-</table>
-
-One last structural simplification is *series collapsing*. Sequential
-motifs--that is, nodes whose names differ by a number at the end and have
-isomorphic structures--are collapsed into a single *stack* of nodes, as shown
-below. For networks with long sequences, this greatly simplifies the view. As
-with hierarchical nodes, double-clicking expands the series. See
-[Interaction](#interaction) for how to disable/enable series collapsing for a
-specific set of nodes.
-
-<table width="100%;">
- <tr>
- <td style="width: 50%;">
- <img src="https://www.tensorflow.org/images/series.png" alt="Sequence of nodes" title="Sequence of nodes" />
- </td>
- <td style="width: 50%;">
- <img src="https://www.tensorflow.org/images/series_expanded.png" alt="Expanded sequence of nodes" title="Expanded sequence of nodes" />
- </td>
- </tr>
- <tr>
- <td style="width: 50%;">
- A collapsed view of a node sequence.
- </td>
- <td style="width: 50%;">
- A small piece of the expanded view, after double-click.
- </td>
- </tr>
-</table>
-
-Finally, as one last aid to legibility, the visualization uses special icons
-for constants and summary nodes. To summarize, here's a table of node symbols:
-
-Symbol | Meaning
---- | ---
- | *High-level* node representing a name scope. Double-click to expand a high-level node.
- | Sequence of numbered nodes that are not connected to each other.
- | Sequence of numbered nodes that are connected to each other.
- | An individual operation node.
- | A constant.
- | A summary node.
- | Edge showing the data flow between operations.
- | Edge showing the control dependency between operations.
- | A reference edge showing that the outgoing operation node can mutate the incoming tensor.
-
-## Interaction {#interaction}
-
-Navigate the graph by panning and zooming. Click and drag to pan, and use a
-scroll gesture to zoom. Double-click on a node, or click on its `+` button, to
-expand a name scope that represents a group of operations. To easily keep
-track of the current viewpoint when zooming and panning, there is a minimap in
-the bottom right corner.
-
-To close an open node, double-click it again or click its `-` button. You can
-also click once to select a node. It will turn a darker color, and details
-about it and the nodes it connects to will appear in the info card at upper
-right corner of the visualization.
-
-<table width="100%;">
- <tr>
- <td style="width: 50%;">
- <img src="https://www.tensorflow.org/images/infocard.png" alt="Info card of a name scope" title="Info card of a name scope" />
- </td>
- <td style="width: 50%;">
- <img src="https://www.tensorflow.org/images/infocard_op.png" alt="Info card of operation node" title="Info card of operation node" />
- </td>
- </tr>
- <tr>
- <td style="width: 50%;">
- Info card showing detailed information for the <code>conv2</code> name scope. The inputs and outputs are combined from the inputs and outputs of the operation nodes inside the name scope. For name scopes no attributes are shown.
- </td>
- <td style="width: 50%;">
- Info card showing detailed information for the <code>DecodeRaw</code> operation node. In addition to inputs and outputs, the card shows the device and the attributes associated with the current operation.
- </td>
- </tr>
-</table>
-
-TensorBoard provides several ways to change the visual layout of the graph. This
-doesn't change the graph's computational semantics, but it can bring some
-clarity to the network's structure. By right clicking on a node or pressing
-buttons on the bottom of that node's info card, you can make the following
-changes to its layout:
-
-* Nodes can be moved between the main graph and the auxiliary area.
-* A series of nodes can be ungrouped so that the nodes in the series do not
-appear grouped together. Ungrouped series can likewise be regrouped.
-
-Selection can also be helpful in understanding high-degree nodes. Select any
-high-degree node, and the corresponding node icons for its other connections
-will be selected as well. This makes it easy, for example, to see which nodes
-are being saved--and which aren't.
-
-Clicking on a node name in the info card will select it. If necessary, the
-viewpoint will automatically pan so that the node is visible.
-
-Finally, you can choose two color schemes for your graph, using the color menu
-above the legend. The default *Structure View* shows structure: when two
-high-level nodes have the same structure, they appear in the same color of the
-rainbow. Uniquely structured nodes are gray. There's a second view, which shows
-what device the different operations run on. Name scopes are colored
-proportionally to the fraction of devices for the operations inside them.
-
-The images below give an illustration for a piece of a real-life graph.
-
-<table width="100%;">
- <tr>
- <td style="width: 50%;">
- <img src="https://www.tensorflow.org/images/colorby_structure.png" alt="Color by structure" title="Color by structure" />
- </td>
- <td style="width: 50%;">
- <img src="https://www.tensorflow.org/images/colorby_device.png" alt="Color by device" title="Color by device" />
- </td>
- </tr>
- <tr>
- <td style="width: 50%;">
- Structure view: The gray nodes have unique structure. The orange <code>conv1</code> and <code>conv2</code> nodes have the same structure, and analogously for nodes with other colors.
- </td>
- <td style="width: 50%;">
- Device view: Name scopes are colored proportionally to the fraction of devices of the operation nodes inside them. Here, purple means GPU and the green is CPU.
- </td>
- </tr>
-</table>
-
-## Tensor shape information
-
-When the serialized `GraphDef` includes tensor shapes, the graph visualizer
-labels edges with tensor dimensions, and edge thickness reflects total tensor
-size. To include tensor shapes in the `GraphDef` pass the actual graph object
-(as in `sess.graph`) to the `FileWriter` when serializing the graph.
-The images below show the CIFAR-10 model with tensor shape information:
-<table width="100%;">
- <tr>
- <td style="width: 100%;">
- <img src="https://www.tensorflow.org/images/tensor_shapes.png" alt="CIFAR-10 model with tensor shape information" title="CIFAR-10 model with tensor shape information" />
- </td>
- </tr>
- <tr>
- <td style="width: 100%;">
- CIFAR-10 model with tensor shape information.
- </td>
- </tr>
-</table>
-
-## Runtime statistics
-
-Often it is useful to collect runtime metadata for a run, such as total memory
-usage, total compute time, and tensor shapes for nodes. The code example below
-is a snippet from the train and test section of a modification of the
-@{$beginners$simple MNIST tutorial},
-in which we have recorded summaries and runtime statistics. See the @{$summaries_and_tensorboard#serializing-the-data$Summaries Tutorial}
-for details on how to record summaries.
-Full source is [here](https://www.tensorflow.org/code/tensorflow/examples/tutorials/mnist/mnist_with_summaries.py).
-
-```python
- # Train the model, and also write summaries.
- # Every 10th step, measure test-set accuracy, and write test summaries
- # All other steps, run train_step on training data, & add training summaries
-
- def feed_dict(train):
- """Make a TensorFlow feed_dict: maps data onto Tensor placeholders."""
- if train or FLAGS.fake_data:
- xs, ys = mnist.train.next_batch(100, fake_data=FLAGS.fake_data)
- k = FLAGS.dropout
- else:
- xs, ys = mnist.test.images, mnist.test.labels
- k = 1.0
- return {x: xs, y_: ys, keep_prob: k}
-
- for i in range(FLAGS.max_steps):
- if i % 10 == 0: # Record summaries and test-set accuracy
- summary, acc = sess.run([merged, accuracy], feed_dict=feed_dict(False))
- test_writer.add_summary(summary, i)
- print('Accuracy at step %s: %s' % (i, acc))
- else: # Record train set summaries, and train
- if i % 100 == 99: # Record execution stats
- run_options = tf.RunOptions(trace_level=tf.RunOptions.FULL_TRACE)
- run_metadata = tf.RunMetadata()
- summary, _ = sess.run([merged, train_step],
- feed_dict=feed_dict(True),
- options=run_options,
- run_metadata=run_metadata)
- train_writer.add_run_metadata(run_metadata, 'step%d' % i)
- train_writer.add_summary(summary, i)
- print('Adding run metadata for', i)
- else: # Record a summary
- summary, _ = sess.run([merged, train_step], feed_dict=feed_dict(True))
- train_writer.add_summary(summary, i)
-```
-
-This code will emit runtime statistics for every 100th step starting at step99.
-
-When you launch tensorboard and go to the Graph tab, you will now see options
-under "Session runs" which correspond to the steps where run metadata was added.
-Selecting one of these runs will show you the snapshot of the network at that
-step, fading out unused nodes. In the controls on the left hand side, you will
-be able to color the nodes by total memory or total compute time. Additionally,
-clicking on a node will display the exact total memory, compute time, and
-tensor output sizes.
-
-
-<table width="100%;">
- <tr style="height: 380px">
- <td>
- <img src="https://www.tensorflow.org/images/colorby_compute_time.png" alt="Color by compute time" title="Color by compute time"/>
- </td>
- <td>
- <img src="https://www.tensorflow.org/images/run_metadata_graph.png" alt="Run metadata graph" title="Run metadata graph" />
- </td>
- <td>
- <img src="https://www.tensorflow.org/images/run_metadata_infocard.png" alt="Run metadata info card" title="Run metadata info card" />
- </td>
- </tr>
-</table>
# Getting Started
-For a brief overview of TensorFlow programming fundamentals, see the following
-guide:
-
- * @{$get_started/get_started$Getting Started with TensorFlow}
-
-MNIST has become the canonical dataset for trying out a new machine learning
-toolkit. We offer three guides that each demonstrate a different approach
-to training an MNIST model on TensorFlow:
-
- * @{$mnist/beginners$MNIST for ML Beginners}, which introduces MNIST through
- the high-level API.
- * @{$mnist/pros$Deep MNIST for Experts}, which is more-in depth than
- "MNIST for ML Beginners," and assumes some familiarity with machine
- learning concepts.
- * @{$mnist/mechanics$TensorFlow Mechanics 101}, which introduces MNIST through
- the low-level API.
-
-For developers new to TensorFlow, the high-level API is a good place to start.
-To learn about the high-level API, read the following guides:
-
- * @{$get_started/estimator$tf.estimator Quickstart}, which introduces this
- API.
- * @{$get_started/input_fn$Building Input Functions},
- which takes you into a somewhat more sophisticated use of this API.
-
-TensorBoard is a utility to visualize different aspects of machine learning.
-The following guides explain how to use TensorBoard:
-
- * @{$get_started/summaries_and_tensorboard$TensorBoard: Visualizing Learning},
- which gets you started.
- * @{$get_started/graph_viz$TensorBoard: Graph Visualization}, which explains
- how to visualize the computational graph. Graph visualization is typically
- more useful for programmers using the low-level API.
-
+TensorFlow is a tool for machine learning. While it contains a wide range of
+functionality, it is mainly designed for deep neural network models.
+
+The fastest way to build a fully-featured model trained on your data is to use
+TensorFlow's high-level API. In the following examples, we will use the
+high-level API on the classic [Iris dataset](https://en.wikipedia.org/wiki/Iris_flower_data_set).
+We will train a model that predicts what species a flower is based on its
+characteristics, and along the way get a quick introduction to the basic tasks
+in TensorFlow using Estimators.
+
+This tutorial is divided into the following parts:
+
+ * @{$get_started/premade_estimators}, which shows you
+ how to quickly setup prebuilt models to train on in-memory data.
+ * @{$get_started/checkpoints}, which shows you how to save training progress,
+ and resume where you left off.
+ * @{$get_started/feature_columns}, which shows how an
+ Estimator can handle a variety of input data types without changes to the
+ model.
+ * @{$get_started/datasets_quickstart}, which is a minimal introduction to
+ the TensorFlow's input pipelines.
+ * @{$get_started/custom_estimators}, which demonstrates how
+ to build and train models you design yourself.
+
+For more advanced users:
+
+ * The @{$low_level_intro$Low Level Introduction} demonstrates how to use
+ tensorflow outside of the Estimator framework, for debugging and
+ experimentation.
+ * The remainder of the @{$programmers_guide$Programmer's Guide} contains
+ in-depth guides to various major components of TensorFlow.
+ * The @{$tutorials$Tutorials} provide walkthroughs of a variety of
+ TensorFlow models.
+++ /dev/null
-# Building Input Functions with tf.estimator
-
-This tutorial introduces you to creating input functions in tf.estimator.
-You'll get an overview of how to construct an `input_fn` to preprocess and feed
-data into your models. Then, you'll implement an `input_fn` that feeds training,
-evaluation, and prediction data into a neural network regressor for predicting
-median house values.
-
-## Custom Input Pipelines with input_fn
-
-The `input_fn` is used to pass feature and target data to the `train`,
-`evaluate`, and `predict` methods of the `Estimator`.
-The user can do feature engineering or pre-processing inside the `input_fn`.
-Here's an example taken from the @{$get_started/estimator$tf.estimator Quickstart tutorial}:
-
-```python
-import numpy as np
-
-training_set = tf.contrib.learn.datasets.base.load_csv_with_header(
- filename=IRIS_TRAINING, target_dtype=np.int, features_dtype=np.float32)
-
-train_input_fn = tf.estimator.inputs.numpy_input_fn(
- x={"x": np.array(training_set.data)},
- y=np.array(training_set.target),
- num_epochs=None,
- shuffle=True)
-
-classifier.train(input_fn=train_input_fn, steps=2000)
-```
-
-### Anatomy of an input_fn
-
-The following code illustrates the basic skeleton for an input function:
-
-```python
-def my_input_fn():
-
- # Preprocess your data here...
-
- # ...then return 1) a mapping of feature columns to Tensors with
- # the corresponding feature data, and 2) a Tensor containing labels
- return feature_cols, labels
-```
-
-The body of the input function contains the specific logic for preprocessing
-your input data, such as scrubbing out bad examples or
-[feature scaling](https://en.wikipedia.org/wiki/Feature_scaling).
-
-Input functions must return the following two values containing the final
-feature and label data to be fed into your model (as shown in the above code
-skeleton):
-
-<dl>
- <dt><code>feature_cols</code></dt>
- <dd>A dict containing key/value pairs that map feature column
-names to <code>Tensor</code>s (or <code>SparseTensor</code>s) containing the corresponding feature
-data.</dd>
- <dt><code>labels</code></dt>
- <dd>A <code>Tensor</code> containing your label (target) values: the values your model aims to predict.</dd>
-</dl>
-
-### Converting Feature Data to Tensors
-
-If your feature/label data is a python array or stored in
-[_pandas_](http://pandas.pydata.org/) dataframes or
-[numpy](http://www.numpy.org/) arrays, you can use the following methods to
-construct `input_fn`:
-
-```python
-import numpy as np
-# numpy input_fn.
-my_input_fn = tf.estimator.inputs.numpy_input_fn(
- x={"x": np.array(x_data)},
- y=np.array(y_data),
- ...)
-```
-
-```python
-import pandas as pd
-# pandas input_fn.
-my_input_fn = tf.estimator.inputs.pandas_input_fn(
- x=pd.DataFrame({"x": x_data}),
- y=pd.Series(y_data),
- ...)
-```
-
-For [sparse, categorical data](https://en.wikipedia.org/wiki/Sparse_matrix)
-(data where the majority of values are 0), you'll instead want to populate a
-`SparseTensor`, which is instantiated with three arguments:
-
-<dl>
- <dt><code>dense_shape</code></dt>
- <dd>The shape of the tensor. Takes a list indicating the number of elements in each dimension. For example, <code>dense_shape=[3,6]</code> specifies a two-dimensional 3x6 tensor, <code>dense_shape=[2,3,4]</code> specifies a three-dimensional 2x3x4 tensor, and <code>dense_shape=[9]</code> specifies a one-dimensional tensor with 9 elements.</dd>
- <dt><code>indices</code></dt>
- <dd>The indices of the elements in your tensor that contain nonzero values. Takes a list of terms, where each term is itself a list containing the index of a nonzero element. (Elements are zero-indexed—i.e., [0,0] is the index value for the element in the first column of the first row in a two-dimensional tensor.) For example, <code>indices=[[1,3], [2,4]]</code> specifies that the elements with indexes of [1,3] and [2,4] have nonzero values.</dd>
- <dt><code>values</code></dt>
- <dd>A one-dimensional tensor of values. Term <code>i</code> in <code>values</code> corresponds to term <code>i</code> in <code>indices</code> and specifies its value. For example, given <code>indices=[[1,3], [2,4]]</code>, the parameter <code>values=[18, 3.6]</code> specifies that element [1,3] of the tensor has a value of 18, and element [2,4] of the tensor has a value of 3.6.</dd>
-</dl>
-
-The following code defines a two-dimensional `SparseTensor` with 3 rows and 5
-columns. The element with index [0,1] has a value of 6, and the element with
-index [2,4] has a value of 0.5 (all other values are 0):
-
-```python
-sparse_tensor = tf.SparseTensor(indices=[[0,1], [2,4]],
- values=[6, 0.5],
- dense_shape=[3, 5])
-```
-
-This corresponds to the following dense tensor:
-
-```none
-[[0, 6, 0, 0, 0]
- [0, 0, 0, 0, 0]
- [0, 0, 0, 0, 0.5]]
-```
-
-For more on `SparseTensor`, see @{tf.SparseTensor}.
-
-### Passing input_fn Data to Your Model
-
-To feed data to your model for training, you simply pass the input function
-you've created to your `train` operation as the value of the `input_fn`
-parameter, e.g.:
-
-```python
-classifier.train(input_fn=my_input_fn, steps=2000)
-```
-
-Note that the `input_fn` parameter must receive a function object (i.e.,
-`input_fn=my_input_fn`), not the return value of a function call
-(`input_fn=my_input_fn()`). This means that if you try to pass parameters to the
-`input_fn` in your `train` call, as in the following code, it will result in a
-`TypeError`:
-
-```python
-classifier.train(input_fn=my_input_fn(training_set), steps=2000)
-```
-
-However, if you'd like to be able to parameterize your input function, there are
-other methods for doing so. You can employ a wrapper function that takes no
-arguments as your `input_fn` and use it to invoke your input function
-with the desired parameters. For example:
-
-```python
-def my_input_fn(data_set):
- ...
-
-def my_input_fn_training_set():
- return my_input_fn(training_set)
-
-classifier.train(input_fn=my_input_fn_training_set, steps=2000)
-```
-
-Alternatively, you can use Python's [`functools.partial`](https://docs.python.org/2/library/functools.html#functools.partial)
-function to construct a new function object with all parameter values fixed:
-
-```python
-classifier.train(
- input_fn=functools.partial(my_input_fn, data_set=training_set),
- steps=2000)
-```
-
-A third option is to wrap your `input_fn` invocation in a
-[`lambda`](https://docs.python.org/3/tutorial/controlflow.html#lambda-expressions)
-and pass it to the `input_fn` parameter:
-
-```python
-classifier.train(input_fn=lambda: my_input_fn(training_set), steps=2000)
-```
-
-One big advantage of designing your input pipeline as shown above—to accept a
-parameter for data set—is that you can pass the same `input_fn` to `evaluate`
-and `predict` operations by just changing the data set argument, e.g.:
-
-```python
-classifier.evaluate(input_fn=lambda: my_input_fn(test_set), steps=2000)
-```
-
-This approach enhances code maintainability: no need to define multiple
-`input_fn` (e.g. `input_fn_train`, `input_fn_test`, `input_fn_predict`) for each
-type of operation.
-
-Finally, you can use the methods in `tf.estimator.inputs` to create `input_fn`
-from numpy or pandas data sets. The additional benefit is that you can use
-more arguments, such as `num_epochs` and `shuffle` to control how the `input_fn`
-iterates over the data:
-
-```python
-import pandas as pd
-
-def get_input_fn_from_pandas(data_set, num_epochs=None, shuffle=True):
- return tf.estimator.inputs.pandas_input_fn(
- x=pd.DataFrame(...),
- y=pd.Series(...),
- num_epochs=num_epochs,
- shuffle=shuffle)
-```
-
-```python
-import numpy as np
-
-def get_input_fn_from_numpy(data_set, num_epochs=None, shuffle=True):
- return tf.estimator.inputs.numpy_input_fn(
- x={...},
- y=np.array(...),
- num_epochs=num_epochs,
- shuffle=shuffle)
-```
-
-### A Neural Network Model for Boston House Values
-
-In the remainder of this tutorial, you'll write an input function for
-preprocessing a subset of Boston housing data pulled from the UCI Housing Data
-Set and use it to feed data to
-a neural network regressor for predicting median house values.
-
-The [Boston CSV data sets](#setup) you'll use to train your neural network
-contain the following
-[feature data](https://archive.ics.uci.edu/ml/machine-learning-databases/housing/housing.names)
-for Boston suburbs:
-
-Feature | Description
-------- | ---------------------------------------------------------------
-CRIM | Crime rate per capita
-ZN | Fraction of residential land zoned to permit 25,000+ sq ft lots
-INDUS | Fraction of land that is non-retail business
-NOX | Concentration of nitric oxides in parts per 10 million
-RM | Average Rooms per dwelling
-AGE | Fraction of owner-occupied residences built before 1940
-DIS | Distance to Boston-area employment centers
-TAX | Property tax rate per $10,000
-PTRATIO | Student-teacher ratio
-
-And the label your model will predict is MEDV, the median value of
-owner-occupied residences in thousands of dollars.
-
-## Setup {#setup}
-
-Download the following data sets:
-[boston_train.csv](http://download.tensorflow.org/data/boston_train.csv),
-[boston_test.csv](http://download.tensorflow.org/data/boston_test.csv), and
-[boston_predict.csv](http://download.tensorflow.org/data/boston_predict.csv).
-
-The following sections provide a step-by-step walkthrough of how to create an
-input function, feed these data sets into a neural network regressor, train and
-evaluate the model, and make house value predictions. The full, final code is [available
-here](https://www.tensorflow.org/code/tensorflow/examples/tutorials/input_fn/boston.py).
-
-### Importing the Housing Data
-
-To start, set up your imports (including `pandas` and `tensorflow`) and set logging verbosity to
-`INFO` for more detailed log output:
-
-```python
-from __future__ import absolute_import
-from __future__ import division
-from __future__ import print_function
-
-import itertools
-
-import pandas as pd
-import tensorflow as tf
-
-tf.logging.set_verbosity(tf.logging.INFO)
-```
-
-Define the column names for the data set in `COLUMNS`. To distinguish features
-from the label, also define `FEATURES` and `LABEL`. Then read the three CSVs
-([train](http://download.tensorflow.org/data/boston_train.csv),
-[test](http://download.tensorflow.org/data/boston_test.csv), and
-[predict](http://download.tensorflow.org/data/boston_predict.csv)) into _pandas_
-`DataFrame`s:
-
-```python
-COLUMNS = ["crim", "zn", "indus", "nox", "rm", "age",
- "dis", "tax", "ptratio", "medv"]
-FEATURES = ["crim", "zn", "indus", "nox", "rm",
- "age", "dis", "tax", "ptratio"]
-LABEL = "medv"
-
-training_set = pd.read_csv("boston_train.csv", skipinitialspace=True,
- skiprows=1, names=COLUMNS)
-test_set = pd.read_csv("boston_test.csv", skipinitialspace=True,
- skiprows=1, names=COLUMNS)
-prediction_set = pd.read_csv("boston_predict.csv", skipinitialspace=True,
- skiprows=1, names=COLUMNS)
-```
-
-### Defining FeatureColumns and Creating the Regressor
-
-Next, create a list of `FeatureColumn`s for the input data, which formally
-specify the set of features to use for training. Because all features in the
-housing data set contain continuous values, you can create their
-`FeatureColumn`s using the `tf.feature_column.numeric_column()` function:
-
-```python
-feature_cols = [tf.feature_column.numeric_column(k) for k in FEATURES]
-```
-
-NOTE: For a more in-depth overview of feature columns, see
-@{$linear#feature-columns-and-transformations$this introduction},
-and for an example that illustrates how to define `FeatureColumns` for
-categorical data, see the @{$wide$Linear Model Tutorial}.
-
-Now, instantiate a `DNNRegressor` for the neural network regression model.
-You'll need to provide two arguments here: `hidden_units`, a hyperparameter
-specifying the number of nodes in each hidden layer (here, two hidden layers
-with 10 nodes each), and `feature_columns`, containing the list of
-`FeatureColumns` you just defined:
-
-```python
-regressor = tf.estimator.DNNRegressor(feature_columns=feature_cols,
- hidden_units=[10, 10],
- model_dir="/tmp/boston_model")
-```
-
-### Building the input_fn
-
-To pass input data into the `regressor`, write a factory method that accepts a
-_pandas_ `Dataframe` and returns an `input_fn`:
-
-```python
-def get_input_fn(data_set, num_epochs=None, shuffle=True):
- return tf.estimator.inputs.pandas_input_fn(
- x=pd.DataFrame({k: data_set[k].values for k in FEATURES}),
- y = pd.Series(data_set[LABEL].values),
- num_epochs=num_epochs,
- shuffle=shuffle)
-```
-
-Note that the input data is passed into `input_fn` in the `data_set` argument,
-which means the function can process any of the `DataFrame`s you've imported:
-`training_set`, `test_set`, and `prediction_set`.
-
-Two additional arguments are provided:
-* `num_epochs`: controls the number of
- epochs to iterate over data. For training, set this to `None`, so the
- `input_fn` keeps returning data until the required number of train steps is
- reached. For evaluate and predict, set this to 1, so the `input_fn` will
- iterate over the data once and then raise `OutOfRangeError`. That error will
- signal the `Estimator` to stop evaluate or predict.
-* `shuffle`: Whether to shuffle the data. For evaluate and predict, set this to
- `False`, so the `input_fn` iterates over the data sequentially. For train,
- set this to `True`.
-
-### Training the Regressor
-
-To train the neural network regressor, run `train` with the `training_set`
-passed to the `input_fn` as follows:
-
-```python
-regressor.train(input_fn=get_input_fn(training_set), steps=5000)
-```
-
-You should see log output similar to the following, which reports training loss
-for every 100 steps:
-
-```none
-INFO:tensorflow:Step 1: loss = 483.179
-INFO:tensorflow:Step 101: loss = 81.2072
-INFO:tensorflow:Step 201: loss = 72.4354
-...
-INFO:tensorflow:Step 1801: loss = 33.4454
-INFO:tensorflow:Step 1901: loss = 32.3397
-INFO:tensorflow:Step 2001: loss = 32.0053
-INFO:tensorflow:Step 4801: loss = 27.2791
-INFO:tensorflow:Step 4901: loss = 27.2251
-INFO:tensorflow:Saving checkpoints for 5000 into /tmp/boston_model/model.ckpt.
-INFO:tensorflow:Loss for final step: 27.1674.
-```
-
-### Evaluating the Model
-
-Next, see how the trained model performs against the test data set. Run
-`evaluate`, and this time pass the `test_set` to the `input_fn`:
-
-```python
-ev = regressor.evaluate(
- input_fn=get_input_fn(test_set, num_epochs=1, shuffle=False))
-```
-
-Retrieve the loss from the `ev` results and print it to output:
-
-```python
-loss_score = ev["loss"]
-print("Loss: {0:f}".format(loss_score))
-```
-
-You should see results similar to the following:
-
-```none
-INFO:tensorflow:Eval steps [0,1) for training step 5000.
-INFO:tensorflow:Saving evaluation summary for 5000 step: loss = 11.9221
-Loss: 11.922098
-```
-
-### Making Predictions
-
-Finally, you can use the model to predict median house values for the
-`prediction_set`, which contains feature data but no labels for six examples:
-
-```python
-y = regressor.predict(
- input_fn=get_input_fn(prediction_set, num_epochs=1, shuffle=False))
-# .predict() returns an iterator of dicts; convert to a list and print
-# predictions
-predictions = list(p["predictions"] for p in itertools.islice(y, 6))
-print("Predictions: {}".format(str(predictions)))
-```
-
-Your results should contain six house-value predictions in thousands of dollars,
-e.g:
-
-```none
-Predictions: [ 33.30348587 17.04452896 22.56370163 34.74345398 14.55953979
- 19.58005714]
-```
-
-## Additional Resources
-
-This tutorial focused on creating an `input_fn` for a neural network regressor.
-To learn more about using `input_fn`s for other types of models, check out the
-following resources:
-
-* @{$linear$Large-scale Linear Models with TensorFlow}: This
- introduction to linear models in TensorFlow provides a high-level overview
- of feature columns and techniques for transforming input data.
-
-* @{$wide$TensorFlow Linear Model Tutorial}: This tutorial covers
- creating `FeatureColumn`s and an `input_fn` for a linear classification
- model that predicts income range based on census data.
-
-* @{$wide_and_deep$TensorFlow Wide & Deep Learning Tutorial}: Building on
- the @{$wide$Linear Model Tutorial}, this tutorial covers
- `FeatureColumn` and `input_fn` creation for a "wide and deep" model that
- combines a linear model and a neural network using
- `DNNLinearCombinedClassifier`.
index.md
-get_started.md
-mnist/beginners.md
-mnist/pros.md
-mnist/mechanics.md
-estimator.md
-input_fn.md
-summaries_and_tensorboard.md
-graph_viz.md
-tensorboard_histograms.md
+premade_estimators.md
+checkpoints.md
+feature_columns.md
+datasets_quickstart.md
+custom_estimators.md
+++ /dev/null
-# MNIST For ML Beginners
-
-*This tutorial is intended for readers who are new to both machine learning and
-TensorFlow. If you already know what MNIST is, and what softmax (multinomial
-logistic) regression is, you might prefer this
-@{$pros$faster paced tutorial}. Be sure to
-@{$install$install TensorFlow} before starting either
-tutorial.*
-
-When one learns how to program, there's a tradition that the first thing you do
-is print "Hello World." Just like programming has Hello World, machine learning
-has MNIST.
-
-MNIST is a simple computer vision dataset. It consists of images of handwritten
-digits like these:
-
-<div style="width:40%; margin:auto; margin-bottom:10px; margin-top:20px;">
-<img style="width:100%" src="https://www.tensorflow.org/images/MNIST.png">
-</div>
-
-It also includes labels for each image, telling us which digit it is. For
-example, the labels for the above images are 5, 0, 4, and 1.
-
-In this tutorial, we're going to train a model to look at images and predict
-what digits they are. Our goal isn't to train a really elaborate model that
-achieves state-of-the-art performance -- although we'll give you code to do that
-later! -- but rather to dip a toe into using TensorFlow. As such, we're going
-to start with a very simple model, called a Softmax Regression.
-
-The actual code for this tutorial is very short, and all the interesting
-stuff happens in just three lines. However, it is very
-important to understand the ideas behind it: both how TensorFlow works and the
-core machine learning concepts. Because of this, we are going to very carefully
-work through the code.
-
-## About this tutorial
-
-This tutorial is an explanation, line by line, of what is happening in the
-[mnist_softmax.py](https://www.tensorflow.org/code/tensorflow/examples/tutorials/mnist/mnist_softmax.py) code.
-
-You can use this tutorial in a few different ways, including:
-
-- Copy and paste each code snippet, line by line, into a Python environment as
- you read through the explanations of each line.
-
-- Run the entire `mnist_softmax.py` Python file either before or after reading
- through the explanations, and use this tutorial to understand the lines of
- code that aren't clear to you.
-
-What we will accomplish in this tutorial:
-
-- Learn about the MNIST data and softmax regressions
-
-- Create a function that is a model for recognizing digits, based on looking at
- every pixel in the image
-
-- Use TensorFlow to train the model to recognize digits by having it "look" at
- thousands of examples (and run our first TensorFlow session to do so)
-
-- Check the model's accuracy with our test data
-
-## The MNIST Data
-
-The MNIST data is hosted on
-[Yann LeCun's website](http://yann.lecun.com/exdb/mnist/). If you are copying and
-pasting in the code from this tutorial, start here with these two lines of code
-which will download and read in the data automatically:
-
-```python
-from tensorflow.examples.tutorials.mnist import input_data
-mnist = input_data.read_data_sets("MNIST_data/", one_hot=True)
-```
-
-The MNIST data is split into three parts: 55,000 data points of training
-data (`mnist.train`), 10,000 points of test data (`mnist.test`), and 5,000
-points of validation data (`mnist.validation`). This split is very important:
-it's essential in machine learning that we have separate data which we don't
-learn from so that we can make sure that what we've learned actually
-generalizes!
-
-As mentioned earlier, every MNIST data point has two parts: an image of a
-handwritten digit and a corresponding label. We'll call the images "x"
-and the labels "y". Both the training set and test set contain images and their
-corresponding labels; for example the training images are `mnist.train.images`
-and the training labels are `mnist.train.labels`.
-
-Each image is 28 pixels by 28 pixels. We can interpret this as a big array of
-numbers:
-
-<div style="width:50%; margin:auto; margin-bottom:10px; margin-top:20px;">
-<img style="width:100%" src="https://www.tensorflow.org/images/MNIST-Matrix.png">
-</div>
-
-We can flatten this array into a vector of 28x28 = 784 numbers. It doesn't
-matter how we flatten the array, as long as we're consistent between images.
-From this perspective, the MNIST images are just a bunch of points in a
-784-dimensional vector space, with a
-[very rich structure](https://colah.github.io/posts/2014-10-Visualizing-MNIST/)
-(warning: computationally intensive visualizations).
-
-Flattening the data throws away information about the 2D structure of the image.
-Isn't that bad? Well, the best computer vision methods do exploit this
-structure, and we will in later tutorials. But the simple method we will be
-using here, a softmax regression (defined below), won't.
-
-The result is that `mnist.train.images` is a tensor (an n-dimensional array)
-with a shape of `[55000, 784]`. The first dimension is an index into the list
-of images and the second dimension is the index for each pixel in each image.
-Each entry in the tensor is a pixel intensity between 0 and 1, for a particular
-pixel in a particular image.
-
-<div style="width:40%; margin:auto; margin-bottom:10px; margin-top:20px;">
-<img style="width:100%" src="https://www.tensorflow.org/images/mnist-train-xs.png">
-</div>
-
-Each image in MNIST has a corresponding label, a number between 0 and 9
-representing the digit drawn in the image.
-
-For the purposes of this tutorial, we're going to want our labels as "one-hot
-vectors". A one-hot vector is a vector which is 0 in most dimensions, and 1 in a
-single dimension. In this case, the \\(n\\)th digit will be represented as a
-vector which is 1 in the \\(n\\)th dimension. For example, 3 would be
-\\([0,0,0,1,0,0,0,0,0,0]\\). Consequently, `mnist.train.labels` is a
-`[55000, 10]` array of floats.
-
-<div style="width:40%; margin:auto; margin-bottom:10px; margin-top:20px;">
-<img style="width:100%" src="https://www.tensorflow.org/images/mnist-train-ys.png">
-</div>
-
-We're now ready to actually make our model!
-
-## Softmax Regressions
-
-We know that every image in MNIST is of a handwritten digit between zero and
-nine. So there are only ten possible things that a given image can be. We want
-to be able to look at an image and give the probabilities for it being each
-digit. For example, our model might look at a picture of a nine and be 80% sure
-it's a nine, but give a 5% chance to it being an eight (because of the top loop)
-and a bit of probability to all the others because it isn't 100% sure.
-
-This is a classic case where a softmax regression is a natural, simple model.
-If you want to assign probabilities to an object being one of several different
-things, softmax is the thing to do, because softmax gives us a list of values
-between 0 and 1 that add up to 1. Even later on, when we train more sophisticated
-models, the final step will be a layer of softmax.
-
-A softmax regression has two steps: first we add up the evidence of our input
-being in certain classes, and then we convert that evidence into probabilities.
-
-To tally up the evidence that a given image is in a particular class, we do a
-weighted sum of the pixel intensities. The weight is negative if that pixel
-having a high intensity is evidence against the image being in that class, and
-positive if it is evidence in favor.
-
-The following diagram shows the weights one model learned for each of these
-classes. Red represents negative weights, while blue represents positive
-weights.
-
-<div style="width:40%; margin:auto; margin-bottom:10px; margin-top:20px;">
-<img style="width:100%" src="https://www.tensorflow.org/images/softmax-weights.png">
-</div>
-
-We also add some extra evidence called a bias. Basically, we want to be able
-to say that some things are more likely independent of the input. The result is
-that the evidence for a class \\(i\\) given an input \\(x\\) is:
-
-$$\text{evidence}_i = \sum_j W_{i,~ j} x_j + b_i$$
-
-where \\(W_i\\) is the weights and \\(b_i\\) is the bias for class \\(i\\),
-and \\(j\\) is an index for summing over the pixels in our input image \\(x\\).
-We then convert the evidence tallies into our predicted probabilities
-\\(y\\) using the "softmax" function:
-
-$$y = \text{softmax}(\text{evidence})$$
-
-Here softmax is serving as an "activation" or "link" function, shaping
-the output of our linear function into the form we want -- in this case, a
-probability distribution over 10 cases.
-You can think of it as converting tallies
-of evidence into probabilities of our input being in each class.
-It's defined as:
-
-$$\text{softmax}(evidence) = \text{normalize}(\exp(evidence))$$
-
-If you expand that equation out, you get:
-
-$$\text{softmax}(evidence)_i = \frac{\exp(evidence_i)}{\sum_j \exp(evidence_j)}$$
-
-But it's often more helpful to think of softmax the first way: exponentiating
-its inputs and then normalizing them. The exponentiation means that one more
-unit of evidence increases the weight given to any hypothesis multiplicatively.
-And conversely, having one less unit of evidence means that a hypothesis gets a
-fraction of its earlier weight. No hypothesis ever has zero or negative
-weight. Softmax then normalizes these weights, so that they add up to one,
-forming a valid probability distribution. (To get more intuition about the
-softmax function, check out the
-[section](http://neuralnetworksanddeeplearning.com/chap3.html#softmax) on it in
-Michael Nielsen's book, complete with an interactive visualization.)
-
-You can picture our softmax regression as looking something like the following,
-although with a lot more \\(x\\)s. For each output, we compute a weighted sum of
-the \\(x\\)s, add a bias, and then apply softmax.
-
-<div style="width:55%; margin:auto; margin-bottom:10px; margin-top:20px;">
-<img style="width:100%" src="https://www.tensorflow.org/images/softmax-regression-scalargraph.png">
-</div>
-
-If we write that out as equations, we get:
-
-<div style="width:52%; margin-left:25%; margin-bottom:10px; margin-top:20px;">
-<img style="width:100%" src="https://www.tensorflow.org/images/softmax-regression-scalarequation.png"
- alt="[y1, y2, y3] = softmax(W11*x1 + W12*x2 + W13*x3 + b1, W21*x1 + W22*x2 + W23*x3 + b2, W31*x1 + W32*x2 + W33*x3 + b3)">
-</div>
-
-We can "vectorize" this procedure, turning it into a matrix multiplication
-and vector addition. This is helpful for computational efficiency. (It's also
-a useful way to think.)
-
-<div style="width:50%; margin:auto; margin-bottom:10px; margin-top:20px;">
-<img style="width:100%" src="https://www.tensorflow.org/images/softmax-regression-vectorequation.png"
- alt="[y1, y2, y3] = softmax([[W11, W12, W13], [W21, W22, W23], [W31, W32, W33]]*[x1, x2, x3] + [b1, b2, b3])">
-</div>
-
-More compactly, we can just write:
-
-$$y = \text{softmax}(Wx + b)$$
-
-Now let's turn that into something that TensorFlow can use.
-
-## Implementing the Regression
-
-
-To do efficient numerical computing in Python, we typically use libraries like
-[NumPy](http://www.numpy.org) that do expensive operations such as matrix
-multiplication outside Python, using highly efficient code implemented in
-another language. Unfortunately, there can still be a lot of overhead from
-switching back to Python every operation. This overhead is especially bad if you
-want to run computations on GPUs or in a distributed manner, where there can be
-a high cost to transferring data.
-
-TensorFlow also does its heavy lifting outside Python, but it takes things a
-step further to avoid this overhead. Instead of running a single expensive
-operation independently from Python, TensorFlow lets us describe a graph of
-interacting operations that run entirely outside Python. (Approaches like this
-can be seen in a few machine learning libraries.)
-
-To use TensorFlow, first we need to import it.
-
-```python
-import tensorflow as tf
-```
-
-We describe these interacting operations by manipulating symbolic variables.
-Let's create one:
-
-```python
-x = tf.placeholder(tf.float32, [None, 784])
-```
-
-`x` isn't a specific value. It's a `placeholder`, a value that we'll input when
-we ask TensorFlow to run a computation. We want to be able to input any number
-of MNIST images, each flattened into a 784-dimensional vector. We represent
-this as a 2-D tensor of floating-point numbers, with a shape `[None, 784]`.
-(Here `None` means that a dimension can be of any length.)
-
-We also need the weights and biases for our model. We could imagine treating
-these like additional inputs, but TensorFlow has an even better way to handle
-it: `Variable`. A `Variable` is a modifiable tensor that lives in TensorFlow's
-graph of interacting operations. It can be used and even modified by the
-computation. For machine learning applications, one generally has the model
-parameters be `Variable`s.
-
-```python
-W = tf.Variable(tf.zeros([784, 10]))
-b = tf.Variable(tf.zeros([10]))
-```
-
-We create these `Variable`s by giving `tf.Variable` the initial value of the
-`Variable`: in this case, we initialize both `W` and `b` as tensors full of
-zeros. Since we are going to learn `W` and `b`, it doesn't matter very much
-what they initially are.
-
-Notice that `W` has a shape of [784, 10] because we want to multiply the
-784-dimensional image vectors by it to produce 10-dimensional vectors of
-evidence for the difference classes. `b` has a shape of [10] so we can add it
-to the output.
-
-We can now implement our model. It only takes one line to define it!
-
-```python
-y = tf.nn.softmax(tf.matmul(x, W) + b)
-```
-
-First, we multiply `x` by `W` with the expression `tf.matmul(x, W)`. This is
-flipped from when we multiplied them in our equation, where we had \\(Wx\\), as
-a small trick to deal with `x` being a 2D tensor with multiple inputs. We then
-add `b`, and finally apply `tf.nn.softmax`.
-
-That's it. It only took us one line to define our model, after a couple short
-lines of setup. That isn't because TensorFlow is designed to make a softmax
-regression particularly easy: it's just a very flexible way to describe many
-kinds of numerical computations, from machine learning models to physics
-simulations. And once defined, our model can be run on different devices:
-your computer's CPU, GPUs, and even phones!
-
-
-## Training
-
-In order to train our model, we need to define what it means for the model to be
-good. Well, actually, in machine learning we typically define what it means for
-a model to be bad. We call this the cost, or the loss, and it represents how far
-off our model is from our desired outcome. We try to minimize that error, and
-the smaller the error margin, the better our model is.
-
-One very common, very nice function to determine the loss of a model is called
-"cross-entropy." Cross-entropy arises from thinking about information
-compressing codes in information theory but it winds up being an important idea
-in lots of areas, from gambling to machine learning. It's defined as:
-
-$$H_{y'}(y) = -\sum_i y'_i \log(y_i)$$
-
-Where \\(y\\) is our predicted probability distribution, and \\(y'\\) is the true
-distribution (the one-hot vector with the digit labels). In some rough sense, the
-cross-entropy is measuring how inefficient our predictions are for describing
-the truth. Going into more detail about cross-entropy is beyond the scope of
-this tutorial, but it's well worth
-[understanding](https://colah.github.io/posts/2015-09-Visual-Information).
-
-To implement cross-entropy we need to first add a new placeholder to input the
-correct answers:
-
-```python
-y_ = tf.placeholder(tf.float32, [None, 10])
-```
-
-Then we can implement the cross-entropy function, \\(-\sum y'\log(y)\\):
-
-```python
-cross_entropy = tf.reduce_mean(-tf.reduce_sum(y_ * tf.log(y), reduction_indices=[1]))
-```
-
-First, `tf.log` computes the logarithm of each element of `y`. Next, we multiply
-each element of `y_` with the corresponding element of `tf.log(y)`. Then
-`tf.reduce_sum` adds the elements in the second dimension of y, due to the
-`reduction_indices=[1]` parameter. Finally, `tf.reduce_mean` computes the mean
-over all the examples in the batch.
-
-Note that in the source code, we don't use this formulation, because it is
-numerically unstable. Instead, we apply
-`tf.losses.sparse_softmax_cross_entropy` on the unnormalized logits (e.g., we
-call `sparse_softmax_cross_entropy` on the output of `tf.matmul(x, W) + b`),
-because this more numerically stable function internally computes the softmax
-activation.
-
-Now that we know what we want our model to do, it's very easy to have TensorFlow
-train it to do so. Because TensorFlow knows the entire graph of your
-computations, it can automatically use the
-[backpropagation algorithm](https://colah.github.io/posts/2015-08-Backprop) to
-efficiently determine how your variables affect the loss you ask it to
-minimize. Then it can apply your choice of optimization algorithm to modify the
-variables and reduce the loss.
-
-```python
-train_step = tf.train.GradientDescentOptimizer(0.5).minimize(cross_entropy)
-```
-
-In this case, we ask TensorFlow to minimize `cross_entropy` using the
-[gradient descent algorithm](https://en.wikipedia.org/wiki/Gradient_descent)
-with a learning rate of 0.5. Gradient descent is a simple procedure, where
-TensorFlow simply shifts each variable a little bit in the direction that
-reduces the cost. But TensorFlow also provides
-@{$python/train#Optimizers$many other optimization algorithms}:
-using one is as simple as tweaking one line.
-
-What TensorFlow actually does here, behind the scenes, is to add new operations
-to your graph which implement backpropagation and gradient descent. Then it
-gives you back a single operation which, when run, does a step of gradient
-descent training, slightly tweaking your variables to reduce the loss.
-
-
-We can now launch the model in an `InteractiveSession`:
-
-```python
-sess = tf.InteractiveSession()
-```
-
-We first have to create an operation to initialize the variables we created:
-
-```python
-tf.global_variables_initializer().run()
-```
-
-
-Let's train -- we'll run the training step 1000 times!
-
-```python
-for _ in range(1000):
- batch_xs, batch_ys = mnist.train.next_batch(100)
- sess.run(train_step, feed_dict={x: batch_xs, y_: batch_ys})
-```
-
-Each step of the loop, we get a "batch" of one hundred random data points from
-our training set. We run `train_step` feeding in the batches data to replace
-the `placeholder`s.
-
-Using small batches of random data is called stochastic training -- in this
-case, stochastic gradient descent. Ideally, we'd like to use all our data for
-every step of training because that would give us a better sense of what we
-should be doing, but that's expensive. So, instead, we use a different subset
-every time. Doing this is cheap and has much of the same benefit.
-
-
-
-## Evaluating Our Model
-
-How well does our model do?
-
-Well, first let's figure out where we predicted the correct label. `tf.argmax`
-is an extremely useful function which gives you the index of the highest entry
-in a tensor along some axis. For example, `tf.argmax(y,1)` is the label our
-model thinks is most likely for each input, while `tf.argmax(y_,1)` is the
-correct label. We can use `tf.equal` to check if our prediction matches the
-truth.
-
-```python
-correct_prediction = tf.equal(tf.argmax(y,1), tf.argmax(y_,1))
-```
-
-That gives us a list of booleans. To determine what fraction are correct, we
-cast to floating point numbers and then take the mean. For example,
-`[True, False, True, True]` would become `[1,0,1,1]` which would become `0.75`.
-
-```python
-accuracy = tf.reduce_mean(tf.cast(correct_prediction, tf.float32))
-```
-
-Finally, we ask for our accuracy on our test data.
-
-```python
-print(sess.run(accuracy, feed_dict={x: mnist.test.images, y_: mnist.test.labels}))
-```
-
-This should be about 92%.
-
-Is that good? Well, not really. In fact, it's pretty bad. This is because we're
-using a very simple model. With some small changes, we can get to 97%. The best
-models can get to over 99.7% accuracy! (For more information, have a look at
-this
-[list of results](https://rodrigob.github.io/are_we_there_yet/build/classification_datasets_results).)
-
-What matters is that we learned from this model. Still, if you're feeling a bit
-down about these results, check out
-@{$pros$the next tutorial} where we do a lot
-better, and learn how to build more sophisticated models using TensorFlow!
+++ /dev/null
-# TensorFlow Mechanics 101
-
-Code: [tensorflow/examples/tutorials/mnist/](https://www.tensorflow.org/code/tensorflow/examples/tutorials/mnist/)
-
-The goal of this tutorial is to show how to use TensorFlow to train and
-evaluate a simple feed-forward neural network for handwritten digit
-classification using the (classic) MNIST data set. The intended audience for
-this tutorial is experienced machine learning users interested in using
-TensorFlow.
-
-These tutorials are not intended for teaching Machine Learning in general.
-
-Please ensure you have followed the instructions to
-@{$install$install TensorFlow}.
-
-## Tutorial Files
-
-This tutorial references the following files:
-
-File | Purpose
---- | ---
-[`mnist.py`](https://www.tensorflow.org/code/tensorflow/examples/tutorials/mnist/mnist.py) | The code to build a fully-connected MNIST model.
-[`fully_connected_feed.py`](https://www.tensorflow.org/code/tensorflow/examples/tutorials/mnist/fully_connected_feed.py) | The main code to train the built MNIST model against the downloaded dataset using a feed dictionary.
-
-Simply run the `fully_connected_feed.py` file directly to start training:
-
-```bash
-python fully_connected_feed.py
-```
-
-## Prepare the Data
-
-MNIST is a classic problem in machine learning. The problem is to look at
-greyscale 28x28 pixel images of handwritten digits and determine which digit
-the image represents, for all the digits from zero to nine.
-
-
-
-For more information, refer to [Yann LeCun's MNIST page](http://yann.lecun.com/exdb/mnist/)
-or [Chris Olah's visualizations of MNIST](http://colah.github.io/posts/2014-10-Visualizing-MNIST/).
-
-### Download
-
-At the top of the `run_training()` method, the `input_data.read_data_sets()`
-function will ensure that the correct data has been downloaded to your local
-training folder and then unpack that data to return a dictionary of `DataSet`
-instances.
-
-```python
-data_sets = input_data.read_data_sets(FLAGS.input_data_dir, FLAGS.fake_data)
-```
-
-**NOTE**: The `fake_data` flag is used for unit-testing purposes and may be
-safely ignored by the reader.
-
-Dataset | Purpose
---- | ---
-`data_sets.train` | 55000 images and labels, for primary training.
-`data_sets.validation` | 5000 images and labels, for iterative validation of training accuracy.
-`data_sets.test` | 10000 images and labels, for final testing of trained accuracy.
-
-### Inputs and Placeholders
-
-The `placeholder_inputs()` function creates two @{tf.placeholder}
-ops that define the shape of the inputs, including the `batch_size`, to the
-rest of the graph and into which the actual training examples will be fed.
-
-```python
-images_placeholder = tf.placeholder(tf.float32, shape=(batch_size,
- mnist.IMAGE_PIXELS))
-labels_placeholder = tf.placeholder(tf.int32, shape=(batch_size))
-```
-
-Further down, in the training loop, the full image and label datasets are
-sliced to fit the `batch_size` for each step, matched with these placeholder
-ops, and then passed into the `sess.run()` function using the `feed_dict`
-parameter.
-
-## Build the Graph
-
-After creating placeholders for the data, the graph is built from the
-`mnist.py` file according to a 3-stage pattern: `inference()`, `loss()`, and
-`training()`.
-
-1. `inference()` - Builds the graph as far as required for running
-the network forward to make predictions.
-1. `loss()` - Adds to the inference graph the ops required to generate
-loss.
-1. `training()` - Adds to the loss graph the ops required to compute
-and apply gradients.
-
-<div style="width:95%; margin:auto; margin-bottom:10px; margin-top:20px;">
- <img style="width:100%" src="https://www.tensorflow.org/images/mnist_subgraph.png">
-</div>
-
-### Inference
-
-The `inference()` function builds the graph as far as needed to
-return the tensor that would contain the output predictions.
-
-It takes the images placeholder as input and builds on top
-of it a pair of fully connected layers with [ReLU](https://en.wikipedia.org/wiki/Rectifier_(neural_networks)) activation followed by a ten
-node linear layer specifying the output logits.
-
-Each layer is created beneath a unique @{tf.name_scope}
-that acts as a prefix to the items created within that scope.
-
-```python
-with tf.name_scope('hidden1'):
-```
-
-Within the defined scope, the weights and biases to be used by each of these
-layers are generated into @{tf.Variable}
-instances, with their desired shapes:
-
-```python
-weights = tf.Variable(
- tf.truncated_normal([IMAGE_PIXELS, hidden1_units],
- stddev=1.0 / math.sqrt(float(IMAGE_PIXELS))),
- name='weights')
-biases = tf.Variable(tf.zeros([hidden1_units]),
- name='biases')
-```
-
-When, for instance, these are created under the `hidden1` scope, the unique
-name given to the weights variable would be "`hidden1/weights`".
-
-Each variable is given initializer ops as part of their construction.
-
-In this most common case, the weights are initialized with the
-@{tf.truncated_normal}
-and given their shape of a 2-D tensor with
-the first dim representing the number of units in the layer from which the
-weights connect and the second dim representing the number of
-units in the layer to which the weights connect. For the first layer, named
-`hidden1`, the dimensions are `[IMAGE_PIXELS, hidden1_units]` because the
-weights are connecting the image inputs to the hidden1 layer. The
-`tf.truncated_normal` initializer generates a random distribution with a given
-mean and standard deviation.
-
-Then the biases are initialized with @{tf.zeros}
-to ensure they start with all zero values, and their shape is simply the number
-of units in the layer to which they connect.
-
-The graph's three primary ops -- two @{tf.nn.relu}
-ops wrapping @{tf.matmul}
-for the hidden layers and one extra `tf.matmul` for the logits -- are then
-created, each in turn, with separate `tf.Variable` instances connected to each
-of the input placeholders or the output tensors of the previous layer.
-
-```python
-hidden1 = tf.nn.relu(tf.matmul(images, weights) + biases)
-```
-
-```python
-hidden2 = tf.nn.relu(tf.matmul(hidden1, weights) + biases)
-```
-
-```python
-logits = tf.matmul(hidden2, weights) + biases
-```
-
-Finally, the `logits` tensor that will contain the output is returned.
-
-### Loss
-
-The `loss()` function further builds the graph by adding the required loss
-ops.
-
-First, the values from the `labels_placeholder` are converted to 64-bit
-integers. Then, a @{tf.losses.sparse_softmax_cross_entropy} op is used to
-calculate the batch's average cross entropy, of the `inference()` result,
-compared to the labels.
-
-```python
-labels = tf.to_int64(labels)
-cross_entropy = tf.losses.sparse_softmax_cross_entropy(
- labels=labels, logits=logits)
-```
-
-And the tensor that will then contain the loss value is returned.
-
-> Note: Cross-entropy is an idea from information theory that allows us
-> to describe how bad it is to believe the predictions of the neural network,
-> given what is actually true. For more information, read the blog post Visual
-> Information Theory (http://colah.github.io/posts/2015-09-Visual-Information/)
-
-### Training
-
-The `training()` function adds the operations needed to minimize the loss via
-[Gradient Descent](https://en.wikipedia.org/wiki/Gradient_descent).
-
-Firstly, it takes the loss tensor from the `loss()` function and hands it to a
-@{tf.summary.scalar},
-an op for generating summary values into the events file when used with a
-@{tf.summary.FileWriter} (see below). In this case, it will emit the snapshot value of
-the loss every time the summaries are written out.
-
-```python
-tf.summary.scalar('loss', loss)
-```
-
-Next, we instantiate a @{tf.train.GradientDescentOptimizer}
-responsible for applying gradients with the requested learning rate.
-
-```python
-optimizer = tf.train.GradientDescentOptimizer(learning_rate)
-```
-
-We then generate a single variable to contain a counter for the global
-training step and the @{tf.train.Optimizer.minimize}
-op is used to both update the trainable weights in the system and increment the
-global step. This op is, by convention, known as the `train_op` and is what must
-be run by a TensorFlow session in order to induce one full step of training
-(see below).
-
-```python
-global_step = tf.Variable(0, name='global_step', trainable=False)
-train_op = optimizer.minimize(loss, global_step=global_step)
-```
-
-## Train the Model
-
-Once the graph is built, it can be iteratively trained and evaluated in a loop
-controlled by the user code in `fully_connected_feed.py`.
-
-### The Graph
-
-At the top of the `run_training()` function is a python `with` command that
-indicates all of the built ops are to be associated with the default
-global @{tf.Graph}
-instance.
-
-```python
-with tf.Graph().as_default():
-```
-
-A `tf.Graph` is a collection of ops that may be executed together as a group.
-Most TensorFlow uses will only need to rely on the single default graph.
-
-More complicated uses with multiple graphs are possible, but beyond the scope of
-this simple tutorial.
-
-### The Session
-
-Once all of the build preparation has been completed and all of the necessary
-ops generated, a @{tf.Session}
-is created for running the graph.
-
-```python
-sess = tf.Session()
-```
-
-Alternately, a `Session` may be generated into a `with` block for scoping:
-
-```python
-with tf.Session() as sess:
-```
-
-The empty parameter to session indicates that this code will attach to
-(or create if not yet created) the default local session.
-
-Immediately after creating the session, all of the `tf.Variable`
-instances are initialized by calling @{tf.Session.run}
-on their initialization op.
-
-```python
-init = tf.global_variables_initializer()
-sess.run(init)
-```
-
-The @{tf.Session.run}
-method will run the complete subset of the graph that
-corresponds to the op(s) passed as parameters. In this first call, the `init`
-op is a @{tf.group}
-that contains only the initializers for the variables. None of the rest of the
-graph is run here; that happens in the training loop below.
-
-### Train Loop
-
-After initializing the variables with the session, training may begin.
-
-The user code controls the training per step, and the simplest loop that
-can do useful training is:
-
-```python
-for step in xrange(FLAGS.max_steps):
- sess.run(train_op)
-```
-
-However, this tutorial is slightly more complicated in that it must also slice
-up the input data for each step to match the previously generated placeholders.
-
-#### Feed the Graph
-
-For each step, the code will generate a feed dictionary that will contain the
-set of examples on which to train for the step, keyed by the placeholder
-ops they represent.
-
-In the `fill_feed_dict()` function, the given `DataSet` is queried for its next
-`batch_size` set of images and labels, and tensors matching the placeholders are
-filled containing the next images and labels.
-
-```python
-images_feed, labels_feed = data_set.next_batch(FLAGS.batch_size,
- FLAGS.fake_data)
-```
-
-A python dictionary object is then generated with the placeholders as keys and
-the representative feed tensors as values.
-
-```python
-feed_dict = {
- images_placeholder: images_feed,
- labels_placeholder: labels_feed,
-}
-```
-
-This is passed into the `sess.run()` function's `feed_dict` parameter to provide
-the input examples for this step of training.
-
-#### Check the Status
-
-The code specifies two values to fetch in its run call: `[train_op, loss]`.
-
-```python
-for step in xrange(FLAGS.max_steps):
- feed_dict = fill_feed_dict(data_sets.train,
- images_placeholder,
- labels_placeholder)
- _, loss_value = sess.run([train_op, loss],
- feed_dict=feed_dict)
-```
-
-Because there are two values to fetch, `sess.run()` returns a tuple with two
-items. Each `Tensor` in the list of values to fetch corresponds to a numpy
-array in the returned tuple, filled with the value of that tensor during this
-step of training. Since `train_op` is an `Operation` with no output value, the
-corresponding element in the returned tuple is `None` and, thus,
-discarded. However, the value of the `loss` tensor may become NaN if the model
-diverges during training, so we capture this value for logging.
-
-Assuming that the training runs fine without NaNs, the training loop also
-prints a simple status text every 100 steps to let the user know the state of
-training.
-
-```python
-if step % 100 == 0:
- print('Step %d: loss = %.2f (%.3f sec)' % (step, loss_value, duration))
-```
-
-#### Visualize the Status
-
-In order to emit the events files used by @{$summaries_and_tensorboard$TensorBoard},
-all of the summaries (in this case, only one) are collected into a single Tensor
-during the graph building phase.
-
-```python
-summary = tf.summary.merge_all()
-```
-
-And then after the session is created, a @{tf.summary.FileWriter}
-may be instantiated to write the events files, which
-contain both the graph itself and the values of the summaries.
-
-```python
-summary_writer = tf.summary.FileWriter(FLAGS.log_dir, sess.graph)
-```
-
-Lastly, the events file will be updated with new summary values every time the
-`summary` is evaluated and the output passed to the writer's `add_summary()`
-function.
-
-```python
-summary_str = sess.run(summary, feed_dict=feed_dict)
-summary_writer.add_summary(summary_str, step)
-```
-
-When the events files are written, TensorBoard may be run against the training
-folder to display the values from the summaries.
-
-
-
-**NOTE**: For more info about how to build and run Tensorboard, please see the accompanying tutorial @{$summaries_and_tensorboard$Tensorboard: Visualizing Learning}.
-
-#### Save a Checkpoint
-
-In order to emit a checkpoint file that may be used to later restore a model
-for further training or evaluation, we instantiate a
-@{tf.train.Saver}.
-
-```python
-saver = tf.train.Saver()
-```
-
-In the training loop, the @{tf.train.Saver.save}
-method will periodically be called to write a checkpoint file to the training
-directory with the current values of all the trainable variables.
-
-```python
-saver.save(sess, checkpoint_file, global_step=step)
-```
-
-At some later point in the future, training might be resumed by using the
-@{tf.train.Saver.restore}
-method to reload the model parameters.
-
-```python
-saver.restore(sess, checkpoint_file)
-```
-
-## Evaluate the Model
-
-Every thousand steps, the code will attempt to evaluate the model against both
-the training and test datasets. The `do_eval()` function is called thrice, for
-the training, validation, and test datasets.
-
-```python
-print('Training Data Eval:')
-do_eval(sess,
- eval_correct,
- images_placeholder,
- labels_placeholder,
- data_sets.train)
-print('Validation Data Eval:')
-do_eval(sess,
- eval_correct,
- images_placeholder,
- labels_placeholder,
- data_sets.validation)
-print('Test Data Eval:')
-do_eval(sess,
- eval_correct,
- images_placeholder,
- labels_placeholder,
- data_sets.test)
-```
-
-> Note that more complicated usage would usually sequester the `data_sets.test`
-> to only be checked after significant amounts of hyperparameter tuning. For
-> the sake of a simple little MNIST problem, however, we evaluate against all of
-> the data.
-
-### Build the Eval Graph
-
-Before entering the training loop, the Eval op should have been built
-by calling the `evaluation()` function from `mnist.py` with the same
-logits/labels parameters as the `loss()` function.
-
-```python
-eval_correct = mnist.evaluation(logits, labels_placeholder)
-```
-
-The `evaluation()` function simply generates a @{tf.nn.in_top_k}
-op that can automatically score each model output as correct if the true label
-can be found in the K most-likely predictions. In this case, we set the value
-of K to 1 to only consider a prediction correct if it is for the true label.
-
-```python
-eval_correct = tf.nn.in_top_k(logits, labels, 1)
-```
-
-### Eval Output
-
-One can then create a loop for filling a `feed_dict` and calling `sess.run()`
-against the `eval_correct` op to evaluate the model on the given dataset.
-
-```python
-for step in xrange(steps_per_epoch):
- feed_dict = fill_feed_dict(data_set,
- images_placeholder,
- labels_placeholder)
- true_count += sess.run(eval_correct, feed_dict=feed_dict)
-```
-
-The `true_count` variable simply accumulates all of the predictions that the
-`in_top_k` op has determined to be correct. From there, the precision may be
-calculated from simply dividing by the total number of examples.
-
-```python
-precision = true_count / num_examples
-print(' Num examples: %d Num correct: %d Precision @ 1: %0.04f' %
- (num_examples, true_count, precision))
-```
+++ /dev/null
-# Deep MNIST for Experts
-
-TensorFlow is a powerful library for doing large-scale numerical computation.
-One of the tasks at which it excels is implementing and training deep neural
-networks. In this tutorial we will learn the basic building blocks of a
-TensorFlow model while constructing a deep convolutional MNIST classifier.
-
-*This introduction assumes familiarity with neural networks and the MNIST
-dataset. If you don't have
-a background with them, check out the
-@{$beginners$introduction for beginners}. Be sure to
-@{$install$install TensorFlow} before starting.*
-
-
-## About this tutorial
-
-The first part of this tutorial explains what is happening in the
-[mnist_softmax.py](https://www.tensorflow.org/code/tensorflow/examples/tutorials/mnist/mnist_softmax.py)
-code, which is a basic implementation of a Tensorflow model. The second part
-shows some ways to improve the accuracy.
-
-You can copy and paste each code snippet from this tutorial into a Python
-environment to follow along, or you can download the fully implemented deep net
-from [mnist_deep.py](https://www.tensorflow.org/code/tensorflow/examples/tutorials/mnist/mnist_deep.py)
-.
-
-What we will accomplish in this tutorial:
-
-- Create a softmax regression function that is a model for recognizing MNIST
- digits, based on looking at every pixel in the image
-
-- Use Tensorflow to train the model to recognize digits by having it "look" at
- thousands of examples (and run our first Tensorflow session to do so)
-
-- Check the model's accuracy with our test data
-
-- Build, train, and test a multilayer convolutional neural network to improve
- the results
-
-## Setup
-
-Before we create our model, we will first load the MNIST dataset, and start a
-TensorFlow session.
-
-### Load MNIST Data
-
-If you are copying and pasting in the code from this tutorial, start here with
-these two lines of code which will download and read in the data automatically:
-
-```python
-from tensorflow.examples.tutorials.mnist import input_data
-mnist = input_data.read_data_sets('MNIST_data')
-```
-
-Here `mnist` is a lightweight class which stores the training, validation, and
-testing sets as NumPy arrays. It also provides a function for iterating through
-data minibatches, which we will use below.
-
-### Start TensorFlow InteractiveSession
-
-TensorFlow relies on a highly efficient C++ backend to do its computation. The
-connection to this backend is called a session. The common usage for TensorFlow
-programs is to first create a graph and then launch it in a session.
-
-Here we instead use the convenient `InteractiveSession` class, which makes
-TensorFlow more flexible about how you structure your code. It allows you to
-interleave operations which build a
-@{$get_started/get_started#the_computational_graph$computation graph}
-with ones that run the graph. This is particularly convenient when working in
-interactive contexts like IPython. If you are not using an
-`InteractiveSession`, then you should build the entire computation graph before
-starting a session and
-@{$get_started/get_started#the_computational_graph$launching the graph}.
-
-```python
-import tensorflow as tf
-sess = tf.InteractiveSession()
-```
-
-#### Computation Graph
-
-To do efficient numerical computing in Python, we typically use libraries like
-[NumPy](http://www.numpy.org/) that do expensive operations such as matrix
-multiplication outside Python, using highly efficient code implemented in
-another language. Unfortunately, there can still be a lot of overhead from
-switching back to Python every operation. This overhead is especially bad if you
-want to run computations on GPUs or in a distributed manner, where there can be
-a high cost to transferring data.
-
-TensorFlow also does its heavy lifting outside Python, but it takes things a
-step further to avoid this overhead. Instead of running a single expensive
-operation independently from Python, TensorFlow lets us describe a graph of
-interacting operations that run entirely outside Python. This approach is
-similar to that used in Theano or Torch.
-
-The role of the Python code is therefore to build this external computation
-graph, and to dictate which parts of the computation graph should be run. See
-the @{$get_started/get_started#the_computational_graph$Computation Graph}
-section of @{$get_started/get_started} for more detail.
-
-## Build a Softmax Regression Model
-
-In this section we will build a softmax regression model with a single linear
-layer. In the next section, we will extend this to the case of softmax
-regression with a multilayer convolutional network.
-
-### Placeholders
-
-We start building the computation graph by creating nodes for the
-input images and target output classes.
-
-```python
-x = tf.placeholder(tf.float32, shape=[None, 784])
-y_ = tf.placeholder(tf.float32, shape=[None, 10])
-```
-
-Here `x` and `y_` aren't specific values. Rather, they are each a `placeholder`
--- a value that we'll input when we ask TensorFlow to run a computation.
-
-The input images `x` will consist of a 2d tensor of floating point numbers.
-Here we assign it a `shape` of `[None, 784]`, where `784` is the dimensionality
-of a single flattened 28 by 28 pixel MNIST image, and `None` indicates that the
-first dimension, corresponding to the batch size, can be of any size. The
-target output classes `y_` will also consist of a 2d tensor, where each row is a
-one-hot 10-dimensional vector indicating which digit class (zero through nine)
-the corresponding MNIST image belongs to.
-
-The `shape` argument to `placeholder` is optional, but it allows TensorFlow
-to automatically catch bugs stemming from inconsistent tensor shapes.
-
-### Variables
-
-We now define the weights `W` and biases `b` for our model. We could imagine
-treating these like additional inputs, but TensorFlow has an even better way to
-handle them: `Variable`. A `Variable` is a value that lives in TensorFlow's
-computation graph. It can be used and even modified by the computation. In
-machine learning applications, one generally has the model parameters be
-`Variable`s.
-
-```python
-W = tf.Variable(tf.zeros([784,10]))
-b = tf.Variable(tf.zeros([10]))
-```
-
-We pass the initial value for each parameter in the call to `tf.Variable`. In
-this case, we initialize both `W` and `b` as tensors full of zeros. `W` is a
-784x10 matrix (because we have 784 input features and 10 outputs) and `b` is a
-10-dimensional vector (because we have 10 classes).
-
-Before `Variable`s can be used within a session, they must be initialized using
-that session. This step takes the initial values (in this case tensors full of
-zeros) that have already been specified, and assigns them to each
-`Variable`. This can be done for all `Variables` at once:
-
-```python
-sess.run(tf.global_variables_initializer())
-```
-
-### Predicted Class and Loss Function
-
-We can now implement our regression model. It only takes one line! We multiply
-the vectorized input images `x` by the weight matrix `W`, add the bias `b`.
-
-```python
-y = tf.matmul(x,W) + b
-```
-
-We can specify a loss function just as easily. Loss indicates how bad the
-model's prediction was on a single example; we try to minimize that while
-training across all the examples. Here, our loss function is the cross-entropy
-between the target and the softmax activation function applied to the model's
-prediction. As in the beginners tutorial, we use the stable formulation:
-
-```python
-cross_entropy = tf.losses.sparse_softmax_cross_entropy(labels=y_, logits=y))
-```
-
-Note that `tf.nn.softmax_cross_entropy_with_logits` internally applies the
-softmax on the model's unnormalized model prediction and sums across all
-classes, and `tf.reduce_mean` takes the average over these sums.
-
-## Train the Model
-
-Now that we have defined our model and training loss function, it is
-straightforward to train using TensorFlow. Because TensorFlow knows the entire
-computation graph, it can use automatic differentiation to find the gradients of
-the loss with respect to each of the variables. TensorFlow has a variety of
-@{$python/train#optimizers$built-in optimization algorithms}.
-For this example, we will use steepest gradient descent, with a step length of
-0.5, to descend the cross entropy.
-
-```python
-train_step = tf.train.GradientDescentOptimizer(0.5).minimize(cross_entropy)
-```
-
-What TensorFlow actually did in that single line was to add new operations to
-the computation graph. These operations included ones to compute gradients,
-compute parameter update steps, and apply update steps to the parameters.
-
-The returned operation `train_step`, when run, will apply the gradient descent
-updates to the parameters. Training the model can therefore be accomplished by
-repeatedly running `train_step`.
-
-```python
-for _ in range(1000):
- batch = mnist.train.next_batch(100)
- train_step.run(feed_dict={x: batch[0], y_: batch[1]})
-```
-
-We load 100 training examples in each training iteration. We then run the
-`train_step` operation, using `feed_dict` to replace the `placeholder` tensors
-`x` and `y_` with the training examples. Note that you can replace any tensor
-in your computation graph using `feed_dict` -- it's not restricted to just
-`placeholder`s.
-
-### Evaluate the Model
-
-How well did our model do?
-
-First we'll figure out where we predicted the correct label. `tf.argmax` is an
-extremely useful function which gives you the index of the highest entry in a
-tensor along some axis. For example, `tf.argmax(y,1)` is the label our model
-thinks is most likely for each input, while `tf.argmax(y_,1)` is the true
-label. We can use `tf.equal` to check if our prediction matches the truth.
-
-```python
-correct_prediction = tf.equal(tf.argmax(y,1), tf.argmax(y_,1))
-```
-
-That gives us a list of booleans. To determine what fraction are correct, we
-cast to floating point numbers and then take the mean. For example,
-`[True, False, True, True]` would become `[1,0,1,1]` which would become `0.75`.
-
-```python
-accuracy = tf.reduce_mean(tf.cast(correct_prediction, tf.float32))
-```
-
-Finally, we can evaluate our accuracy on the test data. This should be about
-92% correct.
-
-```python
-print(accuracy.eval(feed_dict={x: mnist.test.images, y_: mnist.test.labels}))
-```
-
-## Build a Multilayer Convolutional Network
-
-Getting 92% accuracy on MNIST is bad. It's almost embarrassingly bad. In this
-section, we'll fix that, jumping from a very simple model to something
-moderately sophisticated: a small convolutional neural network. This will get us
-to around 99.2% accuracy -- not state of the art, but respectable.
-
-Here is a diagram, created with TensorBoard, of the model we will build:
-
-<div style="width:40%; margin:auto; margin-bottom:10px; margin-top:20px;">
-<img src="https://www.tensorflow.org/images/mnist_deep.png">
-</div>
-
-### Weight Initialization
-
-To create this model, we're going to need to create a lot of weights and biases.
-One should generally initialize weights with a small amount of noise for
-symmetry breaking, and to prevent 0 gradients. Since we're using
-[ReLU](https://en.wikipedia.org/wiki/Rectifier_(neural_networks)) neurons, it is
-also good practice to initialize them with a slightly positive initial bias to
-avoid "dead neurons". Instead of doing this repeatedly while we build the model,
-let's create two handy functions to do it for us.
-
-```python
-def weight_variable(shape):
- initial = tf.truncated_normal(shape, stddev=0.1)
- return tf.Variable(initial)
-
-def bias_variable(shape):
- initial = tf.constant(0.1, shape=shape)
- return tf.Variable(initial)
-```
-
-### Convolution and Pooling
-
-TensorFlow also gives us a lot of flexibility in convolution and pooling
-operations. How do we handle the boundaries? What is our stride size?
-In this example, we're always going to choose the vanilla version.
-Our convolutions uses a stride of one and are zero padded so that the
-output is the same size as the input. Our pooling is plain old max pooling
-over 2x2 blocks. To keep our code cleaner, let's also abstract those operations
-into functions.
-
-```python
-def conv2d(x, W):
- return tf.nn.conv2d(x, W, strides=[1, 1, 1, 1], padding='SAME')
-
-def max_pool_2x2(x):
- return tf.nn.max_pool(x, ksize=[1, 2, 2, 1],
- strides=[1, 2, 2, 1], padding='SAME')
-```
-
-### First Convolutional Layer
-
-We can now implement our first layer. It will consist of convolution, followed
-by max pooling. The convolution will compute 32 features for each 5x5 patch.
-Its weight tensor will have a shape of `[5, 5, 1, 32]`. The first two
-dimensions are the patch size, the next is the number of input channels, and
-the last is the number of output channels. We will also have a bias vector with
-a component for each output channel.
-
-```python
-W_conv1 = weight_variable([5, 5, 1, 32])
-b_conv1 = bias_variable([32])
-```
-
-To apply the layer, we first reshape `x` to a 4d tensor, with the second and
-third dimensions corresponding to image width and height, and the final
-dimension corresponding to the number of color channels.
-
-```python
-x_image = tf.reshape(x, [-1, 28, 28, 1])
-```
-
-We then convolve `x_image` with the weight tensor, add the
-bias, apply the ReLU function, and finally max pool. The `max_pool_2x2` method will
-reduce the image size to 14x14.
-
-```python
-h_conv1 = tf.nn.relu(conv2d(x_image, W_conv1) + b_conv1)
-h_pool1 = max_pool_2x2(h_conv1)
-```
-
-### Second Convolutional Layer
-
-In order to build a deep network, we stack several layers of this type. The
-second layer will have 64 features for each 5x5 patch.
-
-```python
-W_conv2 = weight_variable([5, 5, 32, 64])
-b_conv2 = bias_variable([64])
-
-h_conv2 = tf.nn.relu(conv2d(h_pool1, W_conv2) + b_conv2)
-h_pool2 = max_pool_2x2(h_conv2)
-```
-
-### Densely Connected Layer
-
-Now that the image size has been reduced to 7x7, we add a fully-connected layer
-with 1024 neurons to allow processing on the entire image. We reshape the tensor
-from the pooling layer into a batch of vectors,
-multiply by a weight matrix, add a bias, and apply a ReLU.
-
-```python
-W_fc1 = weight_variable([7 * 7 * 64, 1024])
-b_fc1 = bias_variable([1024])
-
-h_pool2_flat = tf.reshape(h_pool2, [-1, 7*7*64])
-h_fc1 = tf.nn.relu(tf.matmul(h_pool2_flat, W_fc1) + b_fc1)
-```
-
-#### Dropout
-
-To reduce overfitting, we will apply [dropout](
-https://www.cs.toronto.edu/~hinton/absps/JMLRdropout.pdf) before the readout layer.
-We create a `placeholder` for the probability that a neuron's output is kept
-during dropout. This allows us to turn dropout on during training, and turn it
-off during testing.
-TensorFlow's `tf.nn.dropout` op automatically handles scaling neuron outputs in
-addition to masking them, so dropout just works without any additional
-scaling.<sup id="a1">[1](#f1)</sup>
-
-```python
-keep_prob = tf.placeholder(tf.float32)
-h_fc1_drop = tf.nn.dropout(h_fc1, keep_prob)
-```
-
-### Readout Layer
-
-Finally, we add a layer, just like for the one layer softmax regression
-above.
-
-```python
-W_fc2 = weight_variable([1024, 10])
-b_fc2 = bias_variable([10])
-
-y_conv = tf.matmul(h_fc1_drop, W_fc2) + b_fc2
-```
-
-### Train and Evaluate the Model
-
-How well does this model do? To train and evaluate it we will use code that is
-nearly identical to that for the simple one layer SoftMax network above.
-
-The differences are that:
-
-- We will replace the steepest gradient descent optimizer with the more
- sophisticated ADAM optimizer.
-
-- We will include the additional parameter `keep_prob` in `feed_dict` to control
- the dropout rate.
-
-- We will add logging to every 100th iteration in the training process.
-
-We will also use tf.Session rather than tf.InteractiveSession. This better
-separates the process of creating the graph (model specification) and the
-process of evaluating the graph (model fitting). It generally makes for cleaner
-code. The tf.Session is created within a [`with` block](https://docs.python.org/3/whatsnew/2.6.html#pep-343-the-with-statement)
-so that it is automatically destroyed once the block is exited.
-
-Feel free to run this code. Be aware that it does 20,000 training iterations
-and may take a while (possibly up to half an hour), depending on your processor.
-
-```python
-cross_entropy = tf.reduce_mean(
- tf.nn.softmax_cross_entropy_with_logits(labels=y_, logits=y_conv))
-train_step = tf.train.AdamOptimizer(1e-4).minimize(cross_entropy)
-correct_prediction = tf.equal(tf.argmax(y_conv, 1), tf.argmax(y_, 1))
-accuracy = tf.reduce_mean(tf.cast(correct_prediction, tf.float32))
-
-with tf.Session() as sess:
- sess.run(tf.global_variables_initializer())
- for i in range(20000):
- batch = mnist.train.next_batch(50)
- if i % 100 == 0:
- train_accuracy = accuracy.eval(feed_dict={
- x: batch[0], y_: batch[1], keep_prob: 1.0})
- print('step %d, training accuracy %g' % (i, train_accuracy))
- train_step.run(feed_dict={x: batch[0], y_: batch[1], keep_prob: 0.5})
-
- print('test accuracy %g' % accuracy.eval(feed_dict={
- x: mnist.test.images, y_: mnist.test.labels, keep_prob: 1.0}))
-```
-
-The final test set accuracy after running this code should be approximately 99.2%.
-
-We have learned how to quickly and easily build, train, and evaluate a
-fairly sophisticated deep learning model using TensorFlow.
-
-<b id="f1">1</b>: For this small convolutional network, performance is actually nearly identical with and without dropout. Dropout is often very effective at reducing overfitting, but it is most useful when training very large neural networks. [↩](#a1)
+++ /dev/null
-# TensorBoard: Visualizing Learning
-
-The computations you'll use TensorFlow for - like training a massive
-deep neural network - can be complex and confusing. To make it easier to
-understand, debug, and optimize TensorFlow programs, we've included a suite of
-visualization tools called TensorBoard. You can use TensorBoard to visualize
-your TensorFlow graph, plot quantitative metrics about the execution of your
-graph, and show additional data like images that pass through it. When
-TensorBoard is fully configured, it looks like this:
-
-
-
-<div class="video-wrapper">
- <iframe class="devsite-embedded-youtube-video" data-video-id="eBbEDRsCmv4"
- data-autohide="1" data-showinfo="0" frameborder="0" allowfullscreen>
- </iframe>
-</div>
-
-This tutorial is intended to get you started with simple TensorBoard usage.
-There are other resources available as well! The [TensorBoard's GitHub](https://github.com/tensorflow/tensorboard)
-has a lot more information on TensorBoard usage, including tips & tricks, and
-debugging information.
-
-## Serializing the data
-
-TensorBoard operates by reading TensorFlow events files, which contain summary
-data that you can generate when running TensorFlow. Here's the general
-lifecycle for summary data within TensorBoard.
-
-First, create the TensorFlow graph that you'd like to collect summary
-data from, and decide which nodes you would like to annotate with
-@{$python/summary$summary operations}.
-
-For example, suppose you are training a convolutional neural network for
-recognizing MNIST digits. You'd like to record how the learning rate
-varies over time, and how the objective function is changing. Collect these by
-attaching @{tf.summary.scalar} ops
-to the nodes that output the learning rate and loss respectively. Then, give
-each `scalar_summary` a meaningful `tag`, like `'learning rate'` or `'loss
-function'`.
-
-Perhaps you'd also like to visualize the distributions of activations coming
-off a particular layer, or the distribution of gradients or weights. Collect
-this data by attaching
-@{tf.summary.histogram} ops to
-the gradient outputs and to the variable that holds your weights, respectively.
-
-For details on all of the summary operations available, check out the docs on
-@{$python/summary$summary operations}.
-
-Operations in TensorFlow don't do anything until you run them, or an op that
-depends on their output. And the summary nodes that we've just created are
-peripheral to your graph: none of the ops you are currently running depend on
-them. So, to generate summaries, we need to run all of these summary nodes.
-Managing them by hand would be tedious, so use
-@{tf.summary.merge_all}
-to combine them into a single op that generates all the summary data.
-
-Then, you can just run the merged summary op, which will generate a serialized
-`Summary` protobuf object with all of your summary data at a given step.
-Finally, to write this summary data to disk, pass the summary protobuf to a
-@{tf.summary.FileWriter}.
-
-The `FileWriter` takes a logdir in its constructor - this logdir is quite
-important, it's the directory where all of the events will be written out.
-Also, the `FileWriter` can optionally take a `Graph` in its constructor.
-If it receives a `Graph` object, then TensorBoard will visualize your graph
-along with tensor shape information. This will give you a much better sense of
-what flows through the graph: see
-@{$graph_viz#tensor-shape-information$Tensor shape information}.
-
-Now that you've modified your graph and have a `FileWriter`, you're ready to
-start running your network! If you want, you could run the merged summary op
-every single step, and record a ton of training data. That's likely to be more
-data than you need, though. Instead, consider running the merged summary op
-every `n` steps.
-
-The code example below is a modification of the
-@{$beginners$simple MNIST tutorial},
-in which we have added some summary ops, and run them every ten steps. If you
-run this and then launch `tensorboard --logdir=/tmp/tensorflow/mnist`, you'll be able
-to visualize statistics, such as how the weights or accuracy varied during
-training. The code below is an excerpt; full source is
-[here](https://www.tensorflow.org/code/tensorflow/examples/tutorials/mnist/mnist_with_summaries.py).
-
-```python
-def variable_summaries(var):
- """Attach a lot of summaries to a Tensor (for TensorBoard visualization)."""
- with tf.name_scope('summaries'):
- mean = tf.reduce_mean(var)
- tf.summary.scalar('mean', mean)
- with tf.name_scope('stddev'):
- stddev = tf.sqrt(tf.reduce_mean(tf.square(var - mean)))
- tf.summary.scalar('stddev', stddev)
- tf.summary.scalar('max', tf.reduce_max(var))
- tf.summary.scalar('min', tf.reduce_min(var))
- tf.summary.histogram('histogram', var)
-
-def nn_layer(input_tensor, input_dim, output_dim, layer_name, act=tf.nn.relu):
- """Reusable code for making a simple neural net layer.
-
- It does a matrix multiply, bias add, and then uses relu to nonlinearize.
- It also sets up name scoping so that the resultant graph is easy to read,
- and adds a number of summary ops.
- """
- # Adding a name scope ensures logical grouping of the layers in the graph.
- with tf.name_scope(layer_name):
- # This Variable will hold the state of the weights for the layer
- with tf.name_scope('weights'):
- weights = weight_variable([input_dim, output_dim])
- variable_summaries(weights)
- with tf.name_scope('biases'):
- biases = bias_variable([output_dim])
- variable_summaries(biases)
- with tf.name_scope('Wx_plus_b'):
- preactivate = tf.matmul(input_tensor, weights) + biases
- tf.summary.histogram('pre_activations', preactivate)
- activations = act(preactivate, name='activation')
- tf.summary.histogram('activations', activations)
- return activations
-
-hidden1 = nn_layer(x, 784, 500, 'layer1')
-
-with tf.name_scope('dropout'):
- keep_prob = tf.placeholder(tf.float32)
- tf.summary.scalar('dropout_keep_probability', keep_prob)
- dropped = tf.nn.dropout(hidden1, keep_prob)
-
-# Do not apply softmax activation yet, see below.
-y = nn_layer(dropped, 500, 10, 'layer2', act=tf.identity)
-
-with tf.name_scope('cross_entropy'):
- # The raw formulation of cross-entropy,
- #
- # tf.reduce_mean(-tf.reduce_sum(y_ * tf.log(tf.softmax(y)),
- # reduction_indices=[1]))
- #
- # can be numerically unstable.
- #
- # So here we use tf.losses.sparse_softmax_cross_entropy on the
- # raw logit outputs of the nn_layer above.
- with tf.name_scope('total'):
- cross_entropy = tf.losses.sparse_softmax_cross_entropy(labels=y_, logits=y)
-tf.summary.scalar('cross_entropy', cross_entropy)
-
-with tf.name_scope('train'):
- train_step = tf.train.AdamOptimizer(FLAGS.learning_rate).minimize(
- cross_entropy)
-
-with tf.name_scope('accuracy'):
- with tf.name_scope('correct_prediction'):
- correct_prediction = tf.equal(tf.argmax(y, 1), tf.argmax(y_, 1))
- with tf.name_scope('accuracy'):
- accuracy = tf.reduce_mean(tf.cast(correct_prediction, tf.float32))
-tf.summary.scalar('accuracy', accuracy)
-
-# Merge all the summaries and write them out to /tmp/mnist_logs (by default)
-merged = tf.summary.merge_all()
-train_writer = tf.summary.FileWriter(FLAGS.summaries_dir + '/train',
- sess.graph)
-test_writer = tf.summary.FileWriter(FLAGS.summaries_dir + '/test')
-tf.global_variables_initializer().run()
-```
-
-After we've initialized the `FileWriters`, we have to add summaries to the
-`FileWriters` as we train and test the model.
-
-```python
-# Train the model, and also write summaries.
-# Every 10th step, measure test-set accuracy, and write test summaries
-# All other steps, run train_step on training data, & add training summaries
-
-def feed_dict(train):
- """Make a TensorFlow feed_dict: maps data onto Tensor placeholders."""
- if train or FLAGS.fake_data:
- xs, ys = mnist.train.next_batch(100, fake_data=FLAGS.fake_data)
- k = FLAGS.dropout
- else:
- xs, ys = mnist.test.images, mnist.test.labels
- k = 1.0
- return {x: xs, y_: ys, keep_prob: k}
-
-for i in range(FLAGS.max_steps):
- if i % 10 == 0: # Record summaries and test-set accuracy
- summary, acc = sess.run([merged, accuracy], feed_dict=feed_dict(False))
- test_writer.add_summary(summary, i)
- print('Accuracy at step %s: %s' % (i, acc))
- else: # Record train set summaries, and train
- summary, _ = sess.run([merged, train_step], feed_dict=feed_dict(True))
- train_writer.add_summary(summary, i)
-```
-
-You're now all set to visualize this data using TensorBoard.
-
-
-## Launching TensorBoard
-
-To run TensorBoard, use the following command (alternatively `python -m
-tensorboard.main`)
-
-```bash
-tensorboard --logdir=path/to/log-directory
-```
-
-where `logdir` points to the directory where the `FileWriter` serialized its
-data. If this `logdir` directory contains subdirectories which contain
-serialized data from separate runs, then TensorBoard will visualize the data
-from all of those runs. Once TensorBoard is running, navigate your web browser
-to `localhost:6006` to view the TensorBoard.
-
-When looking at TensorBoard, you will see the navigation tabs in the top right
-corner. Each tab represents a set of serialized data that can be visualized.
-
-For in depth information on how to use the *graph* tab to visualize your graph,
-see @{$graph_viz$TensorBoard: Graph Visualization}.
-
-For more usage information on TensorBoard in general, see the [TensorBoard's GitHub](https://github.com/tensorflow/tensorboard).
+++ /dev/null
-# TensorBoard Histogram Dashboard
-
-The TensorBoard Histogram Dashboard displays how the distribution of some
-`Tensor` in your TensorFlow graph has changed over time. It does this by showing
-many histograms visualizations of your tensor at different points in time.
-
-## A Basic Example
-
-Let's start with a simple case: a normally-distributed variable, where the mean
-shifts over time.
-TensorFlow has an op
-[`tf.random_normal`](https://www.tensorflow.org/api_docs/python/tf/random_normal)
-which is perfect for this purpose. As is usually the case with TensorBoard, we
-will ingest data using a summary op; in this case,
-['tf.summary.histogram'](https://www.tensorflow.org/api_docs/python/tf/summary/histogram).
-For a primer on how summaries work, please see the general
-[TensorBoard tutorial](https://www.tensorflow.org/get_started/summaries_and_tensorboard).
-
-Here is a code snippet that will generate some histogram summaries containing
-normally distributed data, where the mean of the distribution increases over
-time.
-
-```python
-import tensorflow as tf
-
-k = tf.placeholder(tf.float32)
-
-# Make a normal distribution, with a shifting mean
-mean_moving_normal = tf.random_normal(shape=[1000], mean=(5*k), stddev=1)
-# Record that distribution into a histogram summary
-tf.summary.histogram("normal/moving_mean", mean_moving_normal)
-
-# Setup a session and summary writer
-sess = tf.Session()
-writer = tf.summary.FileWriter("/tmp/histogram_example")
-
-summaries = tf.summary.merge_all()
-
-# Setup a loop and write the summaries to disk
-N = 400
-for step in range(N):
- k_val = step/float(N)
- summ = sess.run(summaries, feed_dict={k: k_val})
- writer.add_summary(summ, global_step=step)
-```
-
-Once that code runs, we can load the data into TensorBoard via the command line:
-
-
-```sh
-tensorboard --logdir=/tmp/histogram_example
-```
-
-Once TensorBoard is running, load it in Chrome or Firefox and navigate to the
-Histogram Dashboard. Then we can see a histogram visualization for our normally
-distributed data.
-
-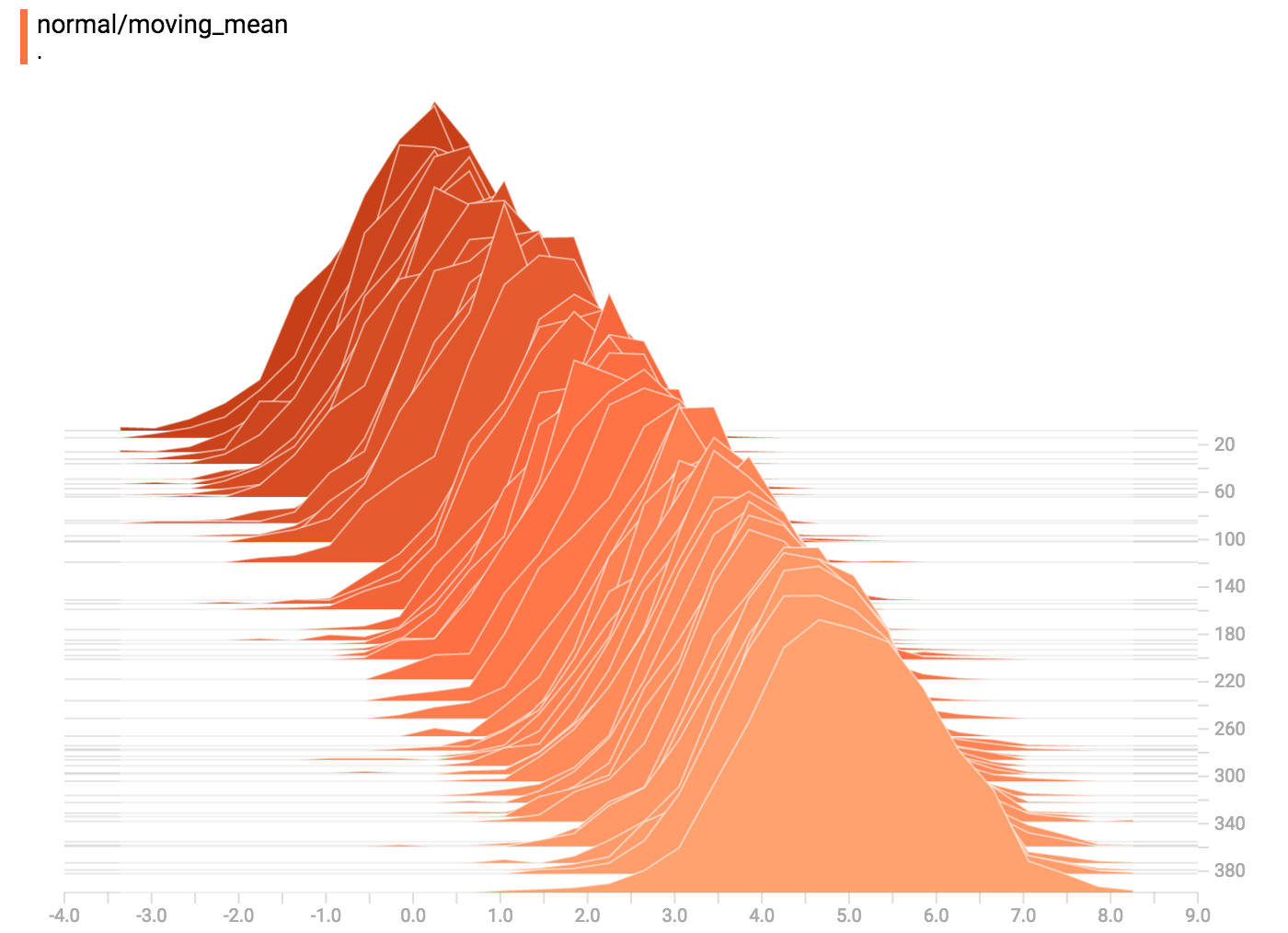
-
-`tf.summary.histogram` takes an arbitrarily sized and shaped Tensor, and
-compresses it into a histogram data structure consisting of many bins with
-widths and counts. For example, let's say we want to organize the numbers
-`[0.5, 1.1, 1.3, 2.2, 2.9, 2.99]` into bins. We could make three bins:
-* a bin
-containing everything from 0 to 1 (it would contain one element, 0.5),
-* a bin
-containing everything from 1-2 (it would contain two elements, 1.1 and 1.3),
-* a bin containing everything from 2-3 (it would contain three elements: 2.2,
-2.9 and 2.99).
-
-TensorFlow uses a similar approach to create bins, but unlike in our example, it
-doesn't create integer bins. For large, sparse datasets, that might result in
-many thousands of bins.
-Instead, [the bins are exponentially distributed, with many bins close to 0 and
-comparatively few bins for very large numbers.](https://github.com/tensorflow/tensorflow/blob/c8b59c046895fa5b6d79f73e0b5817330fcfbfc1/tensorflow/core/lib/histogram/histogram.cc#L28)
-However, visualizing exponentially-distributed bins is tricky; if height is used
-to encode count, then wider bins take more space, even if they have the same
-number of elements. Conversely, encoding count in the area makes height
-comparisons impossible. Instead, the histograms [resample the data](https://github.com/tensorflow/tensorflow/blob/17c47804b86e340203d451125a721310033710f1/tensorflow/tensorboard/components/tf_backend/backend.ts#L400)
-into uniform bins. This can lead to unfortunate artifacts in some cases.
-
-Each slice in the histogram visualizer displays a single histogram.
-The slices are organized by step;
-older slices (e.g. step 0) are further "back" and darker, while newer slices
-(e.g. step 400) are close to the foreground, and lighter in color.
-The y-axis on the right shows the step number.
-
-You can mouse over the histogram to see tooltips with some more detailed
-information. For example, in the following image we can see that the histogram
-at timestep 176 has a bin centered at 2.25 with 177 elements in that bin.
-
-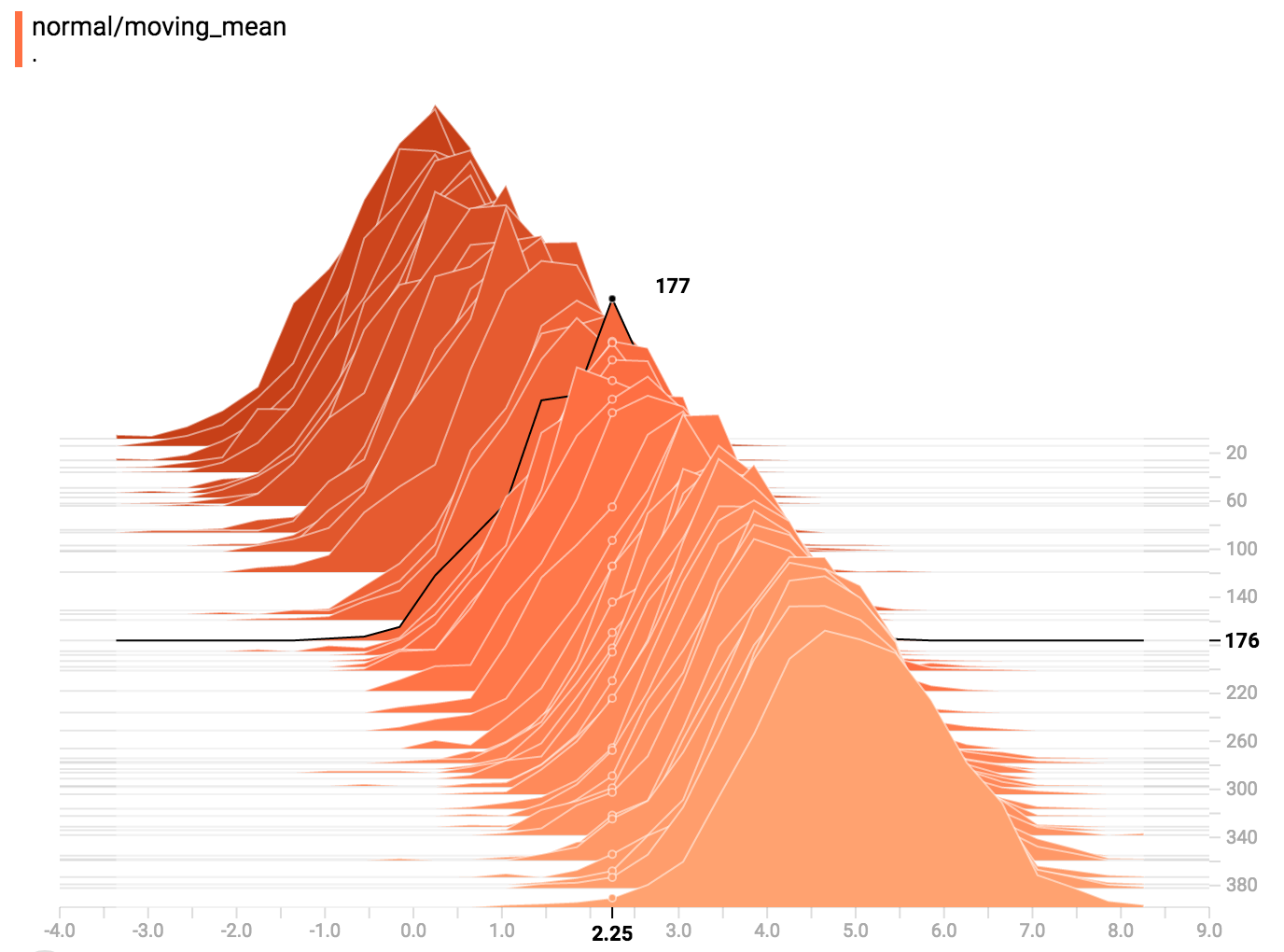
-
-Also, you may note that the histogram slices are not always evenly spaced in
-step count or time. This is because TensorBoard uses
-[reservoir sampling](https://en.wikipedia.org/wiki/Reservoir_sampling) to keep a
-subset of all the histograms, to save on memory. Reservoir sampling guarantees
-that every sample has an equal likelihood of being included, but because it is
-a randomized algorithm, the samples chosen don't occur at even steps.
-
-## Overlay Mode
-
-There is a control on the left of the dashboard that allows you to toggle the
-histogram mode from "offset" to "overlay":
-
-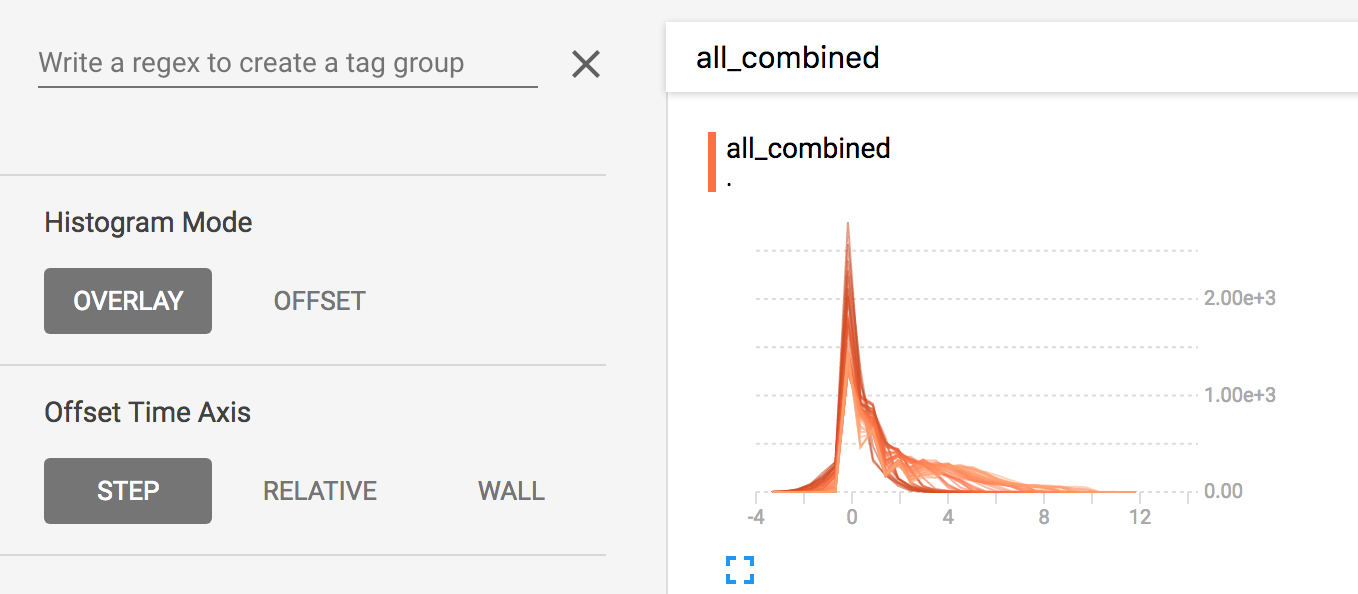
-
-In "offset" mode, the visualization rotates 45 degrees, so that the individual
-histogram slices are no longer spread out in time, but instead are all plotted
-on the same y-axis.
-
-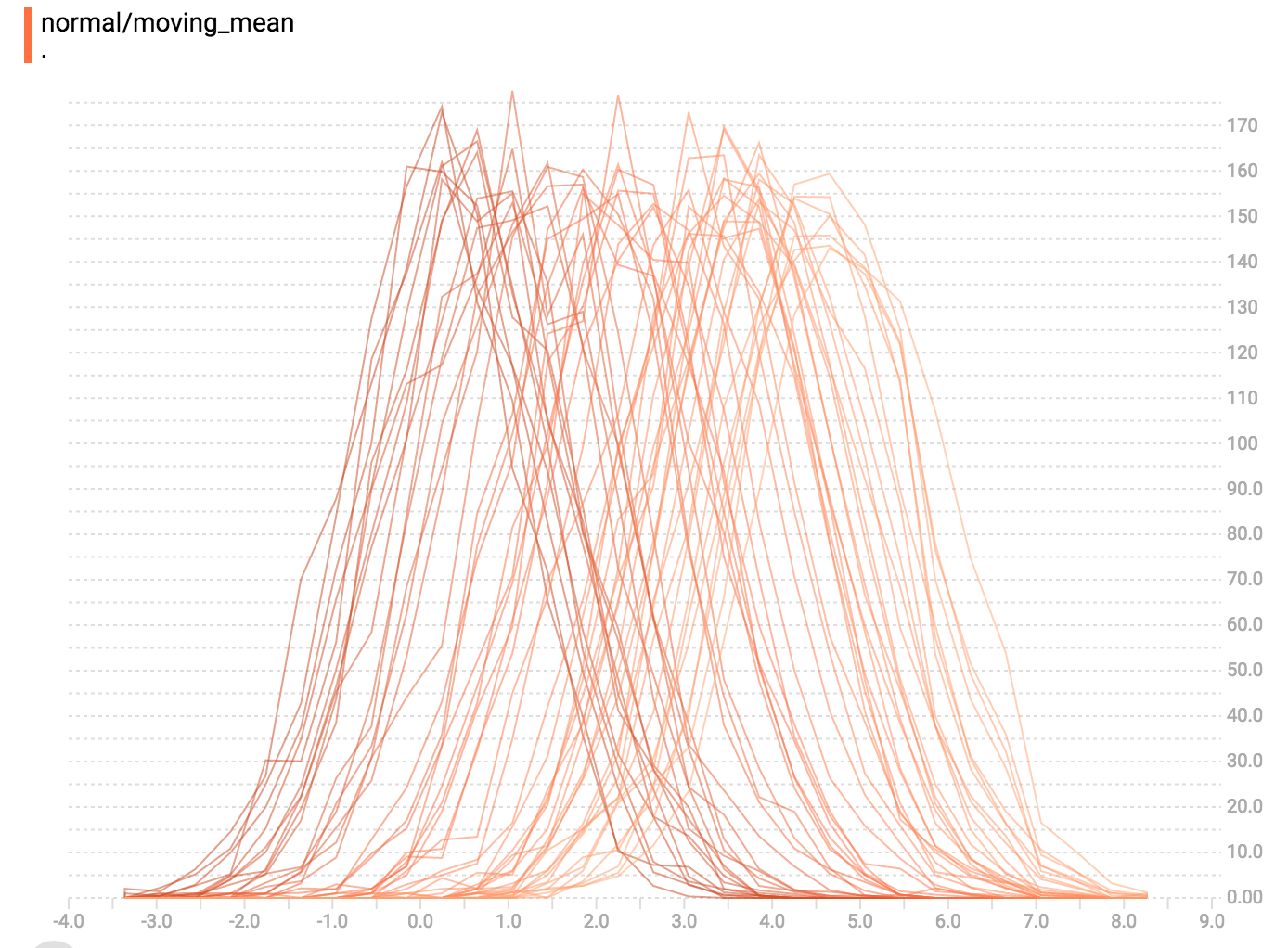
-Now, each slice is a separate line on the chart, and the y-axis shows the item
-count within each bucket. Darker lines are older, earlier steps, and lighter
-lines are more recent, later steps. Once again, you can mouse over the chart to
-see some additional information.
-
-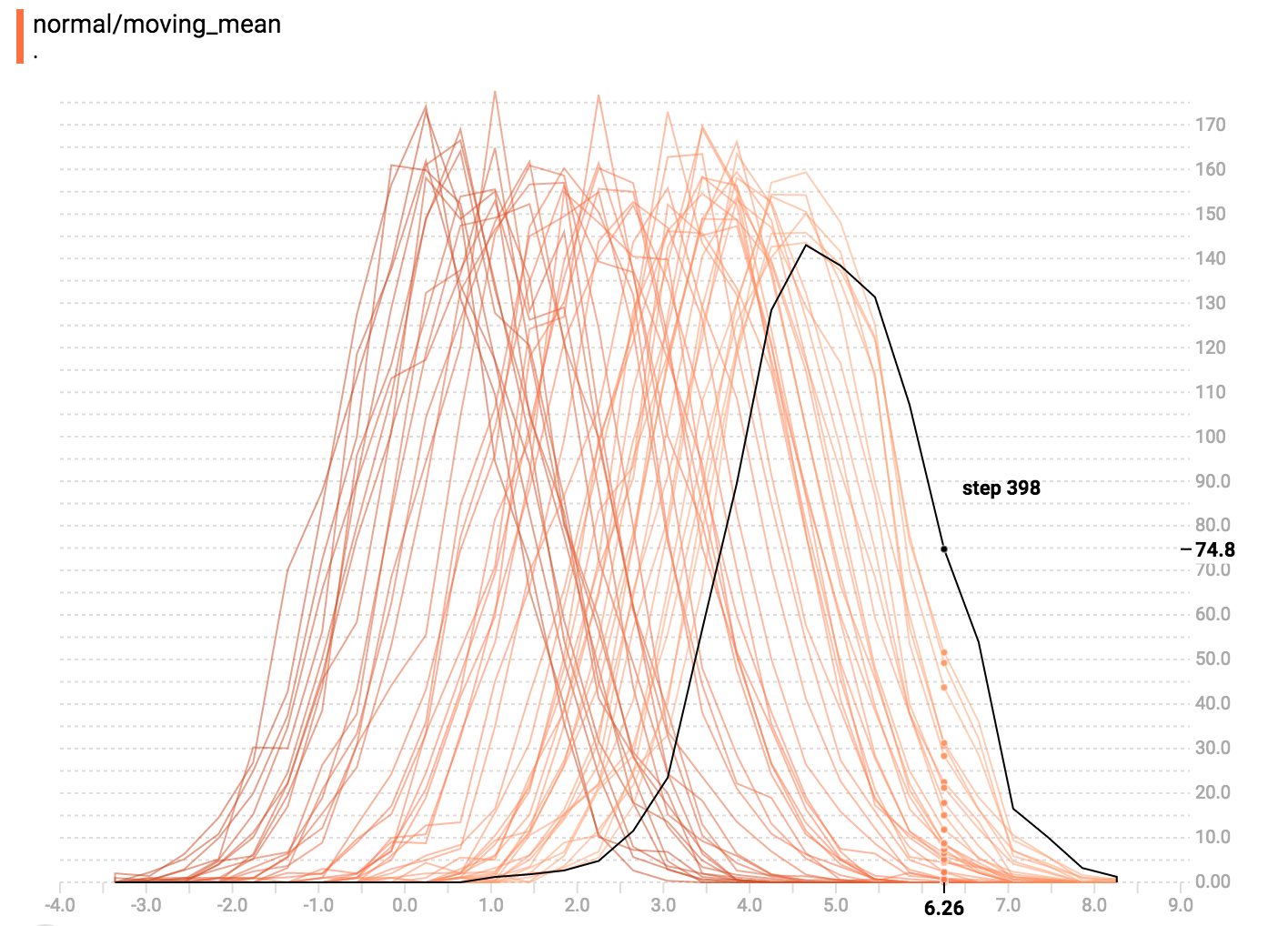
-
-In general, the overlay visualization is useful if you want to directly compare
-the counts of different histograms.
-
-## Multimodal Distributions
-
-The Histogram Dashboard is great for visualizing multimodal
-distributions. Let's construct a simple bimodal distribution by concatenating
-the outputs from two different normal distributions. The code will look like
-this:
-
-```python
-import tensorflow as tf
-
-k = tf.placeholder(tf.float32)
-
-# Make a normal distribution, with a shifting mean
-mean_moving_normal = tf.random_normal(shape=[1000], mean=(5*k), stddev=1)
-# Record that distribution into a histogram summary
-tf.summary.histogram("normal/moving_mean", mean_moving_normal)
-
-# Make a normal distribution with shrinking variance
-variance_shrinking_normal = tf.random_normal(shape=[1000], mean=0, stddev=1-(k))
-# Record that distribution too
-tf.summary.histogram("normal/shrinking_variance", variance_shrinking_normal)
-
-# Let's combine both of those distributions into one dataset
-normal_combined = tf.concat([mean_moving_normal, variance_shrinking_normal], 0)
-# We add another histogram summary to record the combined distribution
-tf.summary.histogram("normal/bimodal", normal_combined)
-
-summaries = tf.summary.merge_all()
-
-# Setup a session and summary writer
-sess = tf.Session()
-writer = tf.summary.FileWriter("/tmp/histogram_example")
-
-# Setup a loop and write the summaries to disk
-N = 400
-for step in range(N):
- k_val = step/float(N)
- summ = sess.run(summaries, feed_dict={k: k_val})
- writer.add_summary(summ, global_step=step)
-```
-
-You already remember our "moving mean" normal distribution from the example
-above. Now we also have a "shrinking variance" distribution. Side-by-side, they
-look like this:
-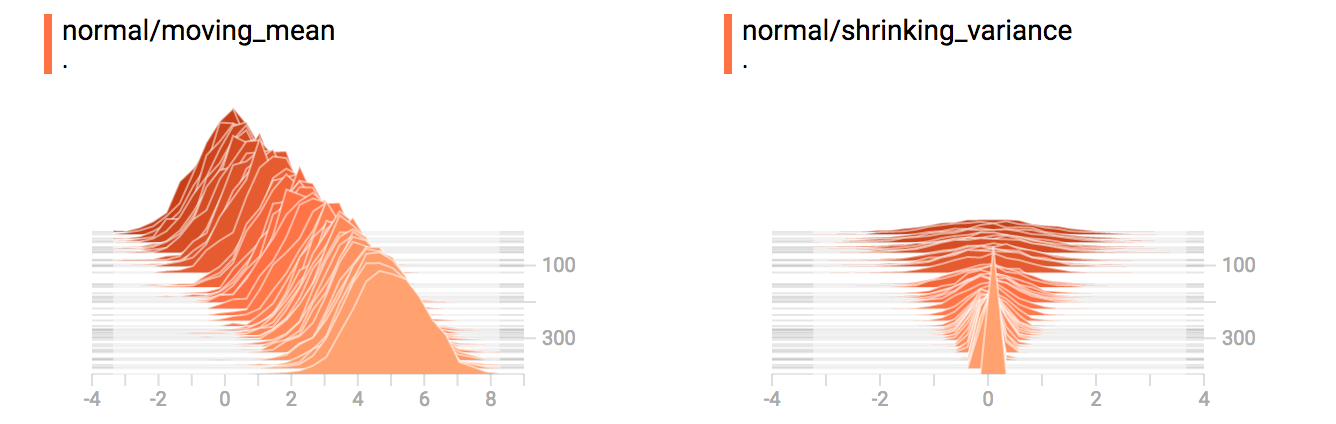
-
-When we concatenate them, we get a chart that clearly reveals the divergent,
-bimodal structure:
-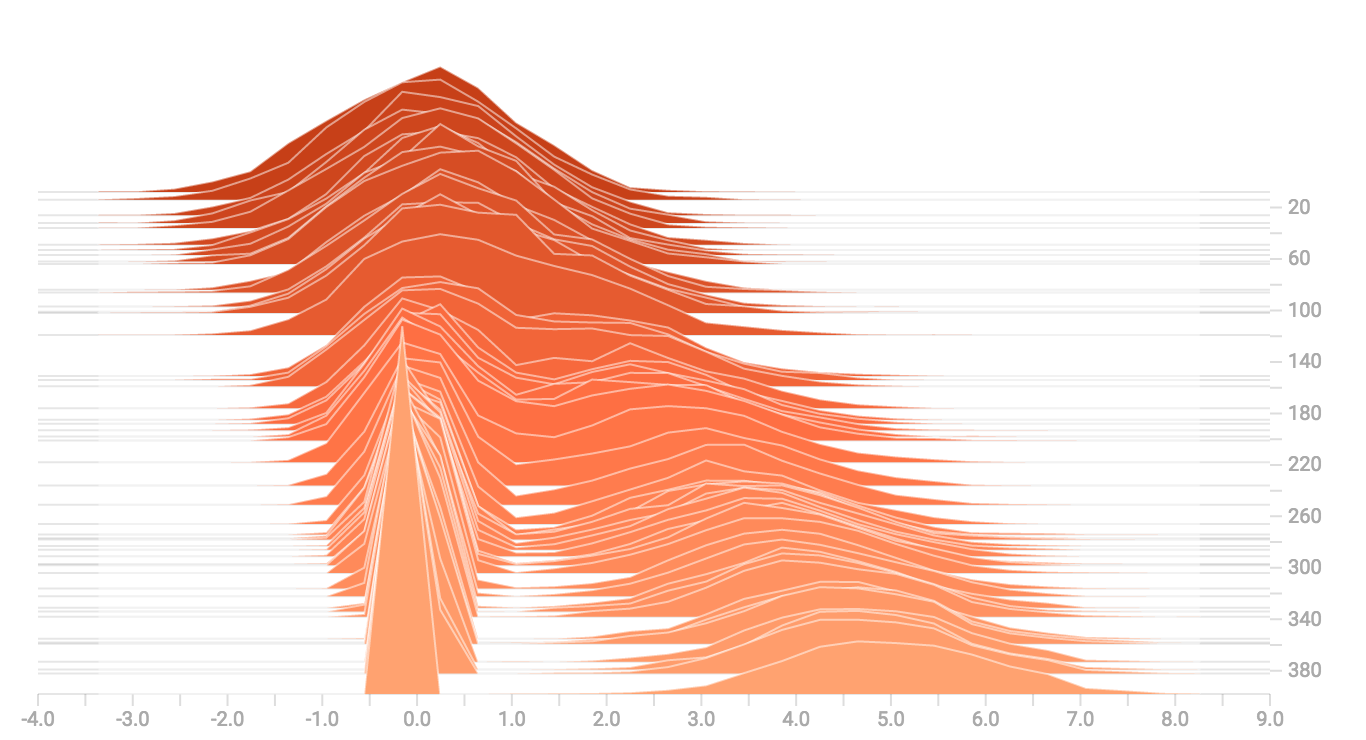
-
-## Some more distributions
-
-Just for fun, let's generate and visualize a few more distributions, and then
-combine them all into one chart. Here's the code we'll use:
-
-```python
-import tensorflow as tf
-
-k = tf.placeholder(tf.float32)
-
-# Make a normal distribution, with a shifting mean
-mean_moving_normal = tf.random_normal(shape=[1000], mean=(5*k), stddev=1)
-# Record that distribution into a histogram summary
-tf.summary.histogram("normal/moving_mean", mean_moving_normal)
-
-# Make a normal distribution with shrinking variance
-variance_shrinking_normal = tf.random_normal(shape=[1000], mean=0, stddev=1-(k))
-# Record that distribution too
-tf.summary.histogram("normal/shrinking_variance", variance_shrinking_normal)
-
-# Let's combine both of those distributions into one dataset
-normal_combined = tf.concat([mean_moving_normal, variance_shrinking_normal], 0)
-# We add another histogram summary to record the combined distribution
-tf.summary.histogram("normal/bimodal", normal_combined)
-
-# Add a gamma distribution
-gamma = tf.random_gamma(shape=[1000], alpha=k)
-tf.summary.histogram("gamma", gamma)
-
-# And a poisson distribution
-poisson = tf.random_poisson(shape=[1000], lam=k)
-tf.summary.histogram("poisson", poisson)
-
-# And a uniform distribution
-uniform = tf.random_uniform(shape=[1000], maxval=k*10)
-tf.summary.histogram("uniform", uniform)
-
-# Finally, combine everything together!
-all_distributions = [mean_moving_normal, variance_shrinking_normal,
- gamma, poisson, uniform]
-all_combined = tf.concat(all_distributions, 0)
-tf.summary.histogram("all_combined", all_combined)
-
-summaries = tf.summary.merge_all()
-
-# Setup a session and summary writer
-sess = tf.Session()
-writer = tf.summary.FileWriter("/tmp/histogram_example")
-
-# Setup a loop and write the summaries to disk
-N = 400
-for step in range(N):
- k_val = step/float(N)
- summ = sess.run(summaries, feed_dict={k: k_val})
- writer.add_summary(summ, global_step=step)
-```
-### Gamma Distribution
-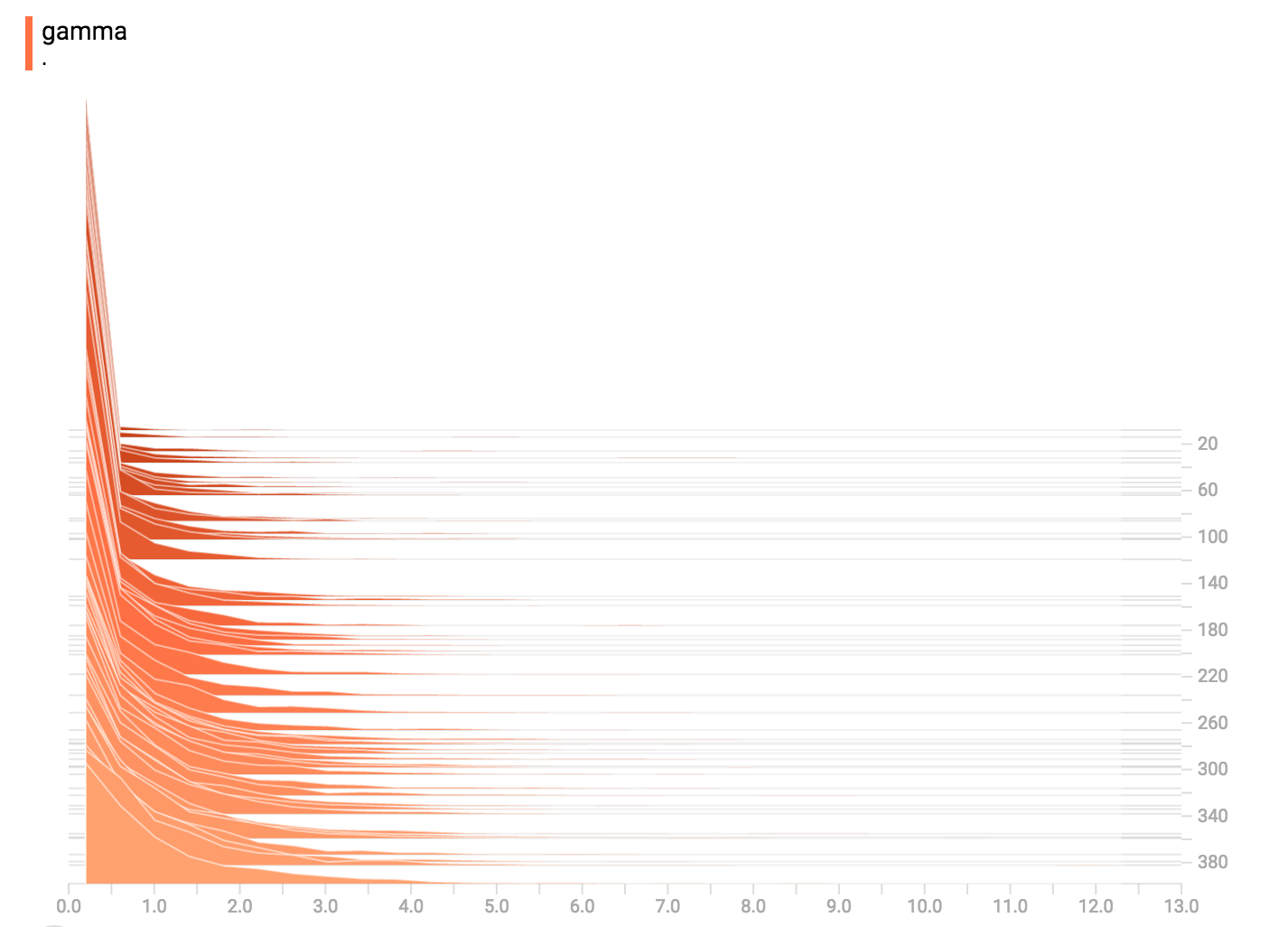
-
-### Uniform Distribution
-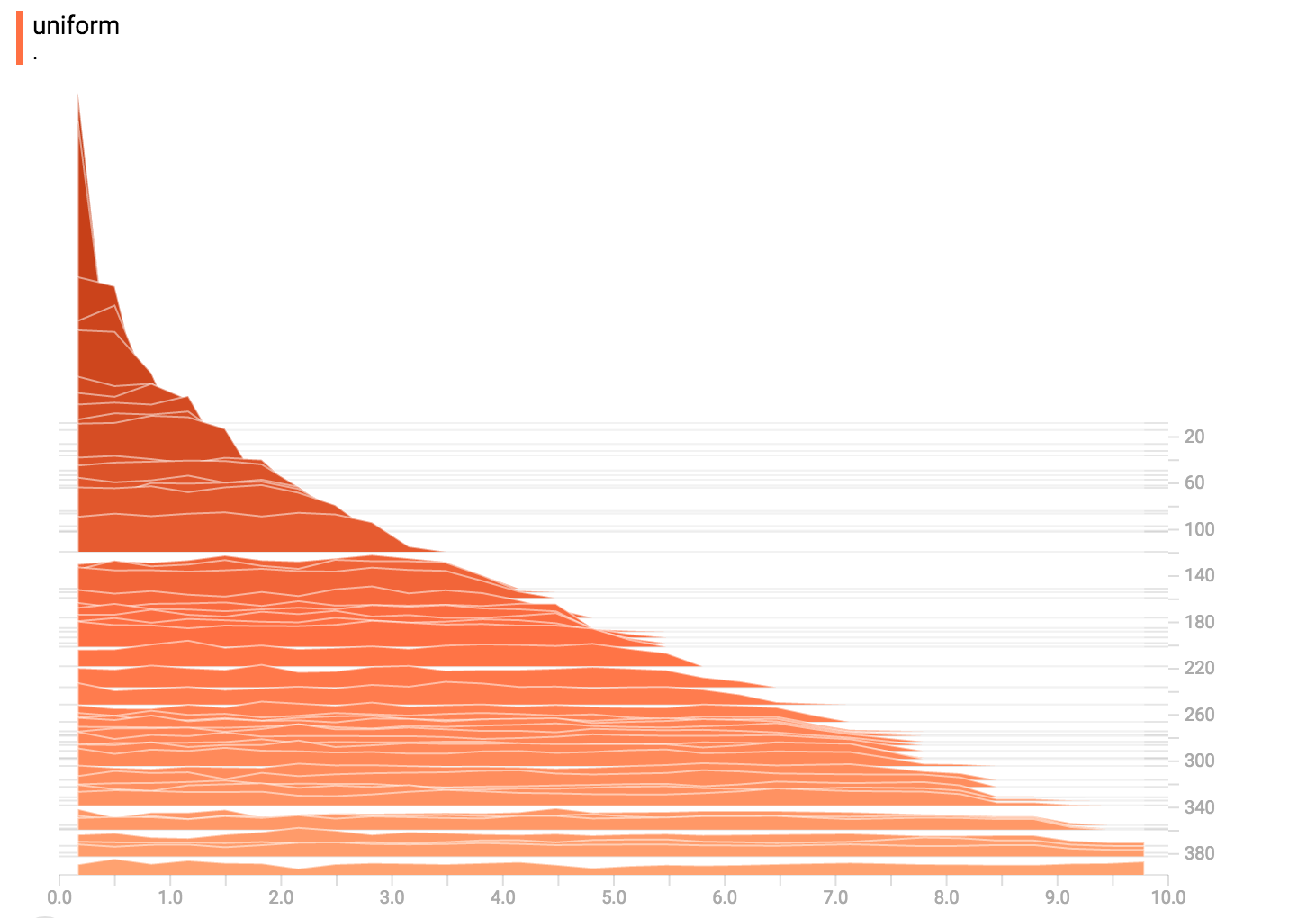
-
-### Poisson Distribution
-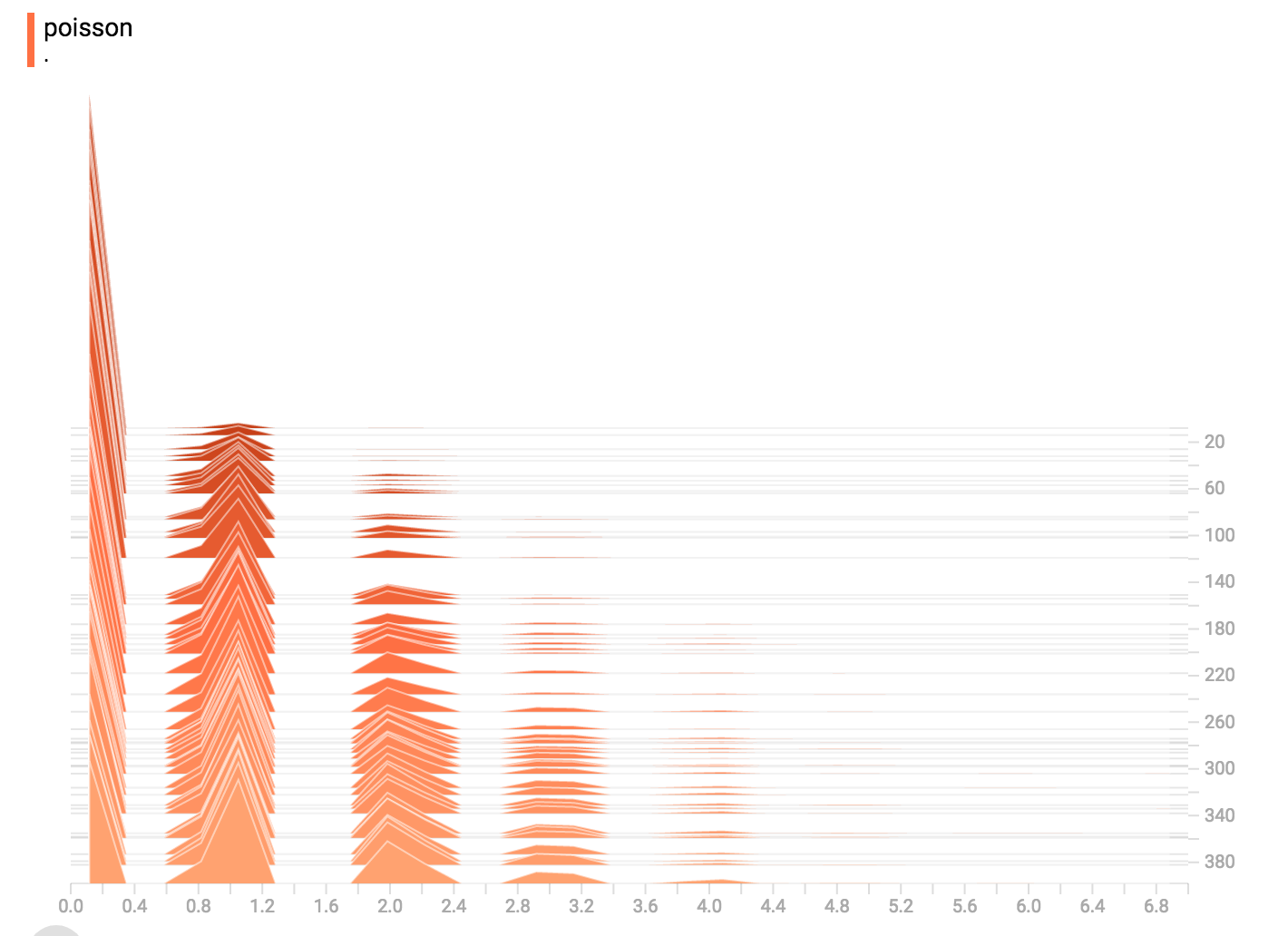
-The poisson distribution is defined over the integers. So, all of the values
-being generated are perfect integers. The histogram compression moves the data
-into floating-point bins, causing the visualization to show little
-bumps over the integer values rather than perfect spikes.
-
-### All Together Now
-Finally, we can concatenate all of the data into one funny-looking curve.
-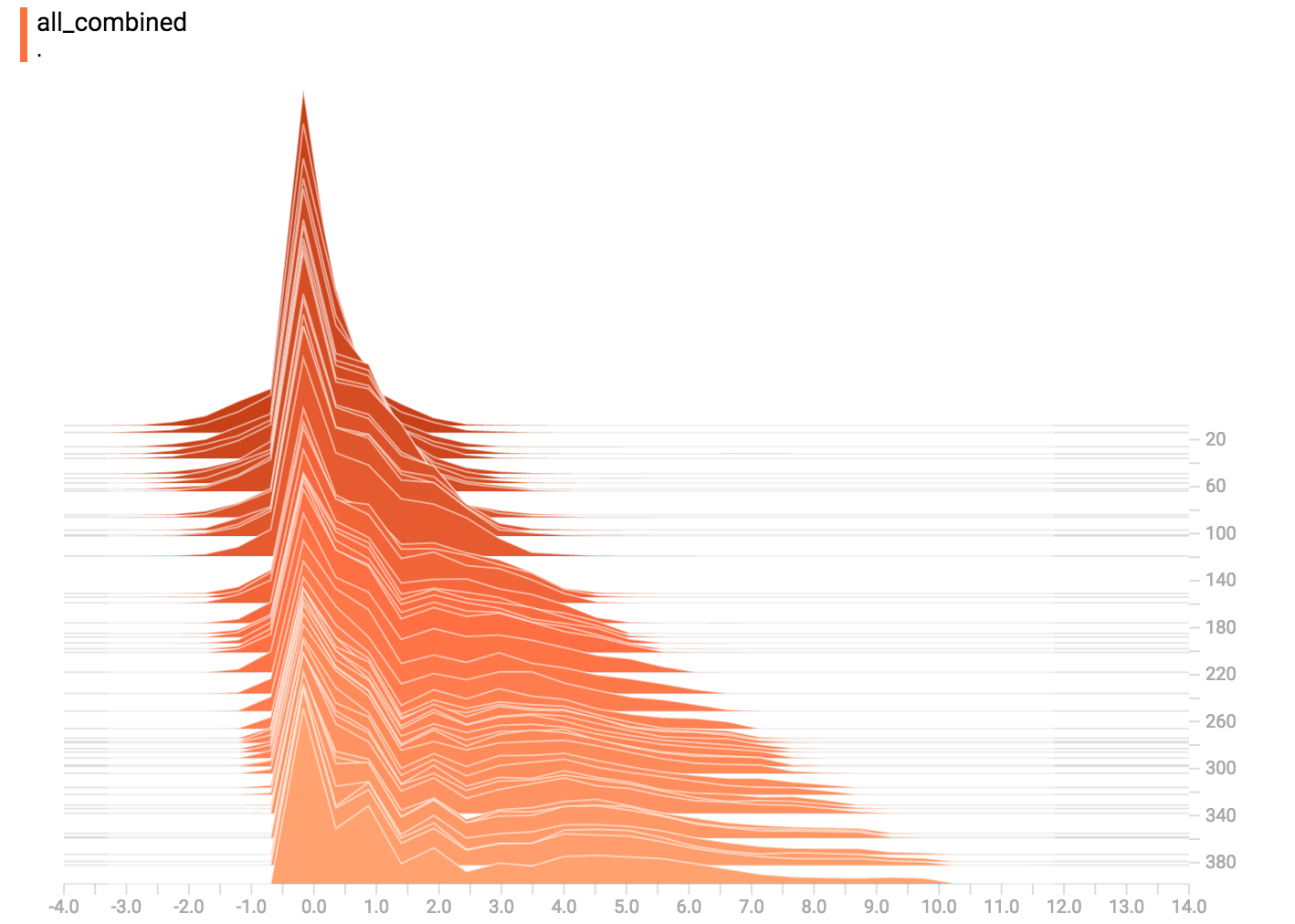
-
<pre>Hello, TensorFlow!</pre>
-If you are new to TensorFlow, see @{$get_started/get_started$Getting Started with TensorFlow}.
+If you are new to TensorFlow, see @{$get_started/premade_estimators$Getting Started with TensorFlow}.
If the system outputs an error message instead of a greeting, see [Common
installation problems](#common_installation_problems).
<pre>Hello, TensorFlow!</pre>
If you are new to TensorFlow, see
-@{$get_started/get_started$Getting Started with TensorFlow}.
+@{$get_started/premade_estimators$Getting Started with TensorFlow}.
If the system outputs an error message instead of a greeting, see
[Common installation problems](#common_installation_problems).
+index.md
+
+### Python
install_linux.md
install_mac.md
install_windows.md
install_sources.md
>>>
migration.md
->>>
+
+### Other Languages
install_java.md
install_go.md
install_c.md
+
+
datasets_performance.md
performance_models.md
benchmarks.md
-quantization.md
->>>
+
+### XLA
xla/index.md
xla/broadcasting.md
xla/developing_new_backend.md
xla/operation_semantics.md
xla/shapes.md
xla/tfcompile.md
+
+### Quantization
+quantization.md
# Importing Data
-The `tf.data` API enables you to build complex input pipelines from
+The @{tf.data} API enables you to build complex input pipelines from
simple, reusable pieces. For example, the pipeline for an image model might
aggregate data from files in a distributed file system, apply random
perturbations to each image, and merge randomly selected images into a batch
This document introduces the concept of embeddings, gives a simple example of
how to train an embedding in TensorFlow, and explains how to view embeddings
-with the TensorBoard Embedding Projector. The first two parts target newcomers
-to machine learning or TensorFlow, and the Embedding Projector how-to is for
-users at all levels.
+with the TensorBoard Embedding Projector
+([live example](http://projector.tensorflow.org)). The first two parts target
+newcomers to machine learning or TensorFlow, and the Embedding Projector how-to
+is for users at all levels.
[TOC]
evaluation, and prediction. When you are using a pre-made Estimator,
someone else has already implemented the model function. When relying
on a custom Estimator, you must write the model function yourself. A
-@{$extend/estimators$companion document}
+@{$get_started/custom_estimators$companion document}
explains how to write the model function.
```
Note that the names of feature columns and labels of a keras estimator come from
the corresponding compiled keras model. For example, the input key names for
-@{$get_started/input_fn} in above `est_inception_v3` estimator can be obtained
-from `keras_inception_v3.input_names`, and similarly, the predicted output
-names can be obtained from `keras_inception_v3.output_names`.
+`train_input_fn` above can be obtained from `keras_inception_v3.input_names`,
+and similarly, the predicted output names can be obtained from
+`keras_inception_v3.output_names`.
For more details, please refer to the documentation for
@{tf.keras.estimator.model_to_estimator}.
numpy arrays (and some other types), which will be used as the values of those
tensors in the execution of a step.
-Often, you have certain tensors, such as inputs, that will always be fed. The
-@{tf.placeholder} op allows you
-to define tensors that *must* be fed, and optionally allows you to constrain
-their shape as well. See the
-@{$beginners$beginners' MNIST tutorial} for an
-example of how placeholders and feeding can be used to provide the training data
-for a neural network.
-
#### What is the difference between `Session.run()` and `Tensor.eval()`?
If `t` is a @{tf.Tensor} object,
--- /dev/null
+# TensorBoard: Graph Visualization
+
+TensorFlow computation graphs are powerful but complicated. The graph visualization can help you understand and debug them. Here's an example of the visualization at work.
+
+
+*Visualization of a TensorFlow graph.*
+
+To see your own graph, run TensorBoard pointing it to the log directory of the job, click on the graph tab on the top pane and select the appropriate run using the menu at the upper left corner. For in depth information on how to run TensorBoard and make sure you are logging all the necessary information, see @{$summaries_and_tensorboard$TensorBoard: Visualizing Learning}.
+
+## Name scoping and nodes
+
+Typical TensorFlow graphs can have many thousands of nodes--far too many to see
+easily all at once, or even to lay out using standard graph tools. To simplify,
+variable names can be scoped and the visualization uses this information to
+define a hierarchy on the nodes in the graph. By default, only the top of this
+hierarchy is shown. Here is an example that defines three operations under the
+`hidden` name scope using
+@{tf.name_scope}:
+
+```python
+import tensorflow as tf
+
+with tf.name_scope('hidden') as scope:
+ a = tf.constant(5, name='alpha')
+ W = tf.Variable(tf.random_uniform([1, 2], -1.0, 1.0), name='weights')
+ b = tf.Variable(tf.zeros([1]), name='biases')
+```
+
+This results in the following three op names:
+
+* `hidden/alpha`
+* `hidden/weights`
+* `hidden/biases`
+
+By default, the visualization will collapse all three into a node labeled `hidden`.
+The extra detail isn't lost. You can double-click, or click
+on the orange `+` sign in the top right to expand the node, and then you'll see
+three subnodes for `alpha`, `weights` and `biases`.
+
+Here's a real-life example of a more complicated node in its initial and
+expanded states.
+
+<table width="100%;">
+ <tr>
+ <td style="width: 50%;">
+ <img src="https://www.tensorflow.org/images/pool1_collapsed.png" alt="Unexpanded name scope" title="Unexpanded name scope" />
+ </td>
+ <td style="width: 50%;">
+ <img src="https://www.tensorflow.org/images/pool1_expanded.png" alt="Expanded name scope" title="Expanded name scope" />
+ </td>
+ </tr>
+ <tr>
+ <td style="width: 50%;">
+ Initial view of top-level name scope <code>pool_1</code>. Clicking on the orange <code>+</code> button on the top right or double-clicking on the node itself will expand it.
+ </td>
+ <td style="width: 50%;">
+ Expanded view of <code>pool_1</code> name scope. Clicking on the orange <code>-</code> button on the top right or double-clicking on the node itself will collapse the name scope.
+ </td>
+ </tr>
+</table>
+
+Grouping nodes by name scopes is critical to making a legible graph. If you're
+building a model, name scopes give you control over the resulting visualization.
+**The better your name scopes, the better your visualization.**
+
+The figure above illustrates a second aspect of the visualization. TensorFlow
+graphs have two kinds of connections: data dependencies and control
+dependencies. Data dependencies show the flow of tensors between two ops and
+are shown as solid arrows, while control dependencies use dotted lines. In the
+expanded view (right side of the figure above) all the connections are data
+dependencies with the exception of the dotted line connecting `CheckNumerics`
+and `control_dependency`.
+
+There's a second trick to simplifying the layout. Most TensorFlow graphs have a
+few nodes with many connections to other nodes. For example, many nodes might
+have a control dependency on an initialization step. Drawing all edges between
+the `init` node and its dependencies would create a very cluttered view.
+
+To reduce clutter, the visualization separates out all high-degree nodes to an
+*auxiliary* area on the right and doesn't draw lines to represent their edges.
+Instead of lines, we draw small *node icons* to indicate the connections.
+Separating out the auxiliary nodes typically doesn't remove critical
+information since these nodes are usually related to bookkeeping functions.
+See [Interaction](#interaction) for how to move nodes between the main graph
+and the auxiliary area.
+
+<table width="100%;">
+ <tr>
+ <td style="width: 50%;">
+ <img src="https://www.tensorflow.org/images/conv_1.png" alt="conv_1 is part of the main graph" title="conv_1 is part of the main graph" />
+ </td>
+ <td style="width: 50%;">
+ <img src="https://www.tensorflow.org/images/save.png" alt="save is extracted as auxiliary node" title="save is extracted as auxiliary node" />
+ </td>
+ </tr>
+ <tr>
+ <td style="width: 50%;">
+ Node <code>conv_1</code> is connected to <code>save</code>. Note the little <code>save</code> node icon on its right.
+ </td>
+ <td style="width: 50%;">
+ <code>save</code> has a high degree, and will appear as an auxiliary node. The connection with <code>conv_1</code> is shown as a node icon on its left. To further reduce clutter, since <code>save</code> has a lot of connections, we show the first 5 and abbreviate the others as <code>... 12 more</code>.
+ </td>
+ </tr>
+</table>
+
+One last structural simplification is *series collapsing*. Sequential
+motifs--that is, nodes whose names differ by a number at the end and have
+isomorphic structures--are collapsed into a single *stack* of nodes, as shown
+below. For networks with long sequences, this greatly simplifies the view. As
+with hierarchical nodes, double-clicking expands the series. See
+[Interaction](#interaction) for how to disable/enable series collapsing for a
+specific set of nodes.
+
+<table width="100%;">
+ <tr>
+ <td style="width: 50%;">
+ <img src="https://www.tensorflow.org/images/series.png" alt="Sequence of nodes" title="Sequence of nodes" />
+ </td>
+ <td style="width: 50%;">
+ <img src="https://www.tensorflow.org/images/series_expanded.png" alt="Expanded sequence of nodes" title="Expanded sequence of nodes" />
+ </td>
+ </tr>
+ <tr>
+ <td style="width: 50%;">
+ A collapsed view of a node sequence.
+ </td>
+ <td style="width: 50%;">
+ A small piece of the expanded view, after double-click.
+ </td>
+ </tr>
+</table>
+
+Finally, as one last aid to legibility, the visualization uses special icons
+for constants and summary nodes. To summarize, here's a table of node symbols:
+
+Symbol | Meaning
+--- | ---
+ | *High-level* node representing a name scope. Double-click to expand a high-level node.
+ | Sequence of numbered nodes that are not connected to each other.
+ | Sequence of numbered nodes that are connected to each other.
+ | An individual operation node.
+ | A constant.
+ | A summary node.
+ | Edge showing the data flow between operations.
+ | Edge showing the control dependency between operations.
+ | A reference edge showing that the outgoing operation node can mutate the incoming tensor.
+
+## Interaction {#interaction}
+
+Navigate the graph by panning and zooming. Click and drag to pan, and use a
+scroll gesture to zoom. Double-click on a node, or click on its `+` button, to
+expand a name scope that represents a group of operations. To easily keep
+track of the current viewpoint when zooming and panning, there is a minimap in
+the bottom right corner.
+
+To close an open node, double-click it again or click its `-` button. You can
+also click once to select a node. It will turn a darker color, and details
+about it and the nodes it connects to will appear in the info card at upper
+right corner of the visualization.
+
+<table width="100%;">
+ <tr>
+ <td style="width: 50%;">
+ <img src="https://www.tensorflow.org/images/infocard.png" alt="Info card of a name scope" title="Info card of a name scope" />
+ </td>
+ <td style="width: 50%;">
+ <img src="https://www.tensorflow.org/images/infocard_op.png" alt="Info card of operation node" title="Info card of operation node" />
+ </td>
+ </tr>
+ <tr>
+ <td style="width: 50%;">
+ Info card showing detailed information for the <code>conv2</code> name scope. The inputs and outputs are combined from the inputs and outputs of the operation nodes inside the name scope. For name scopes no attributes are shown.
+ </td>
+ <td style="width: 50%;">
+ Info card showing detailed information for the <code>DecodeRaw</code> operation node. In addition to inputs and outputs, the card shows the device and the attributes associated with the current operation.
+ </td>
+ </tr>
+</table>
+
+TensorBoard provides several ways to change the visual layout of the graph. This
+doesn't change the graph's computational semantics, but it can bring some
+clarity to the network's structure. By right clicking on a node or pressing
+buttons on the bottom of that node's info card, you can make the following
+changes to its layout:
+
+* Nodes can be moved between the main graph and the auxiliary area.
+* A series of nodes can be ungrouped so that the nodes in the series do not
+appear grouped together. Ungrouped series can likewise be regrouped.
+
+Selection can also be helpful in understanding high-degree nodes. Select any
+high-degree node, and the corresponding node icons for its other connections
+will be selected as well. This makes it easy, for example, to see which nodes
+are being saved--and which aren't.
+
+Clicking on a node name in the info card will select it. If necessary, the
+viewpoint will automatically pan so that the node is visible.
+
+Finally, you can choose two color schemes for your graph, using the color menu
+above the legend. The default *Structure View* shows structure: when two
+high-level nodes have the same structure, they appear in the same color of the
+rainbow. Uniquely structured nodes are gray. There's a second view, which shows
+what device the different operations run on. Name scopes are colored
+proportionally to the fraction of devices for the operations inside them.
+
+The images below give an illustration for a piece of a real-life graph.
+
+<table width="100%;">
+ <tr>
+ <td style="width: 50%;">
+ <img src="https://www.tensorflow.org/images/colorby_structure.png" alt="Color by structure" title="Color by structure" />
+ </td>
+ <td style="width: 50%;">
+ <img src="https://www.tensorflow.org/images/colorby_device.png" alt="Color by device" title="Color by device" />
+ </td>
+ </tr>
+ <tr>
+ <td style="width: 50%;">
+ Structure view: The gray nodes have unique structure. The orange <code>conv1</code> and <code>conv2</code> nodes have the same structure, and analogously for nodes with other colors.
+ </td>
+ <td style="width: 50%;">
+ Device view: Name scopes are colored proportionally to the fraction of devices of the operation nodes inside them. Here, purple means GPU and the green is CPU.
+ </td>
+ </tr>
+</table>
+
+## Tensor shape information
+
+When the serialized `GraphDef` includes tensor shapes, the graph visualizer
+labels edges with tensor dimensions, and edge thickness reflects total tensor
+size. To include tensor shapes in the `GraphDef` pass the actual graph object
+(as in `sess.graph`) to the `FileWriter` when serializing the graph.
+The images below show the CIFAR-10 model with tensor shape information:
+<table width="100%;">
+ <tr>
+ <td style="width: 100%;">
+ <img src="https://www.tensorflow.org/images/tensor_shapes.png" alt="CIFAR-10 model with tensor shape information" title="CIFAR-10 model with tensor shape information" />
+ </td>
+ </tr>
+ <tr>
+ <td style="width: 100%;">
+ CIFAR-10 model with tensor shape information.
+ </td>
+ </tr>
+</table>
+
+## Runtime statistics
+
+Often it is useful to collect runtime metadata for a run, such as total memory
+usage, total compute time, and tensor shapes for nodes. The code example below
+is a snippet from the train and test section of a modification of the
+@{$layers$simple MNIST tutorial}, in which we have recorded summaries and
+runtime statistics. See the
+@{$summaries_and_tensorboard#serializing-the-data$Summaries Tutorial}
+for details on how to record summaries.
+Full source is [here](https://www.tensorflow.org/code/tensorflow/examples/tutorials/mnist/mnist_with_summaries.py).
+
+```python
+ # Train the model, and also write summaries.
+ # Every 10th step, measure test-set accuracy, and write test summaries
+ # All other steps, run train_step on training data, & add training summaries
+
+ def feed_dict(train):
+ """Make a TensorFlow feed_dict: maps data onto Tensor placeholders."""
+ if train or FLAGS.fake_data:
+ xs, ys = mnist.train.next_batch(100, fake_data=FLAGS.fake_data)
+ k = FLAGS.dropout
+ else:
+ xs, ys = mnist.test.images, mnist.test.labels
+ k = 1.0
+ return {x: xs, y_: ys, keep_prob: k}
+
+ for i in range(FLAGS.max_steps):
+ if i % 10 == 0: # Record summaries and test-set accuracy
+ summary, acc = sess.run([merged, accuracy], feed_dict=feed_dict(False))
+ test_writer.add_summary(summary, i)
+ print('Accuracy at step %s: %s' % (i, acc))
+ else: # Record train set summaries, and train
+ if i % 100 == 99: # Record execution stats
+ run_options = tf.RunOptions(trace_level=tf.RunOptions.FULL_TRACE)
+ run_metadata = tf.RunMetadata()
+ summary, _ = sess.run([merged, train_step],
+ feed_dict=feed_dict(True),
+ options=run_options,
+ run_metadata=run_metadata)
+ train_writer.add_run_metadata(run_metadata, 'step%d' % i)
+ train_writer.add_summary(summary, i)
+ print('Adding run metadata for', i)
+ else: # Record a summary
+ summary, _ = sess.run([merged, train_step], feed_dict=feed_dict(True))
+ train_writer.add_summary(summary, i)
+```
+
+This code will emit runtime statistics for every 100th step starting at step99.
+
+When you launch tensorboard and go to the Graph tab, you will now see options
+under "Session runs" which correspond to the steps where run metadata was added.
+Selecting one of these runs will show you the snapshot of the network at that
+step, fading out unused nodes. In the controls on the left hand side, you will
+be able to color the nodes by total memory or total compute time. Additionally,
+clicking on a node will display the exact total memory, compute time, and
+tensor output sizes.
+
+
+<table width="100%;">
+ <tr style="height: 380px">
+ <td>
+ <img src="https://www.tensorflow.org/images/colorby_compute_time.png" alt="Color by compute time" title="Color by compute time"/>
+ </td>
+ <td>
+ <img src="https://www.tensorflow.org/images/run_metadata_graph.png" alt="Run metadata graph" title="Run metadata graph" />
+ </td>
+ <td>
+ <img src="https://www.tensorflow.org/images/run_metadata_infocard.png" alt="Run metadata info card" title="Run metadata info card" />
+ </td>
+ </tr>
+</table>
# Programmer's Guide
-The documents in this unit dive into the details of writing TensorFlow
-code. For TensorFlow 1.3, we revised this document extensively.
-The units are now as follows:
+The documents in this unit dive into the details of how TensorFlow
+works. The units are as follows:
- * @{$programmers_guide/estimators$Estimators}, which introduces a high-level
+## High Level APIs
+
+ * @{$programmers_guide/estimators}, which introduces a high-level
TensorFlow API that greatly simplifies ML programming.
- * @{$programmers_guide/tensors$Tensors}, which explains how to create,
+ * @{$programmers_guide/datasets}, which explains how to
+ set up data pipelines to read data sets into your TensorFlow program.
+
+## Low Level APIs
+
+ * @{$programmers_guide/low_level_intro}, which introduces the
+ basics of how you can to use TensorFlow outside of the high Level APIs.
+ * @{$programmers_guide/tensors}, which explains how to create,
manipulate, and access Tensors--the fundamental object in TensorFlow.
- * @{$programmers_guide/variables$Variables}, which details how
+ * @{$programmers_guide/variables}, which details how
to represent shared, persistent state in your program.
- * @{$programmers_guide/graphs$Graphs and Sessions}, which explains:
+ * @{$programmers_guide/graphs}, which explains:
* dataflow graphs, which are TensorFlow's representation of computations
as dependencies between operations.
* sessions, which are TensorFlow's mechanism for running dataflow graphs
such as Estimators or Keras, the high-level API creates and manages
graphs and sessions for you, but understanding graphs and sessions
can still be helpful.
- * @{$programmers_guide/saved_model$Saving and Restoring}, which
+ * @{$programmers_guide/saved_model}, which
explains how to save and restore variables and models.
- * @{$programmers_guide/datasets$Input Pipelines}, which explains how to
- set up data pipelines to read data sets into your TensorFlow program.
- * @{$programmers_guide/embedding$Embeddings}, which introduces the concept
+ * @{$using_gpu} explains how TensorFlow assigns operations to
+ devices and how you can change the arrangement manually.
+
+
+## ML Concepts
+
+ * @{$programmers_guide/embedding}, which introduces the concept
of embeddings, provides a simple example of training an embedding in
TensorFlow, and explains how to view embeddings with the TensorBoard
Embedding Projector.
- * @{$programmers_guide/debugger$Debugging TensorFlow Programs}, which
+
+## Debugging
+
+ * @{$programmers_guide/debugger}, which
explains how to use the TensorFlow debugger (tfdbg).
- * @{$programmers_guide/version_compat$TensorFlow Version Compatibility},
+
+## TensorBoard
+
+TensorBoard is a utility to visualize different aspects of machine learning.
+The following guides explain how to use TensorBoard:
+
+ * @{$programmers_guide/summaries_and_tensorboard},
+ which introduces TensorBoard.
+ * @{$programmers_guide/graph_viz}, which
+ explains how to visualize the computational graph.
+ * @{$programmers_guide/tensorboard_histograms} which demonstrates the how to
+ use TensorBoard's histogram dashboard.
+
+
+## Misc
+
+ * @{$programmers_guide/version_compat},
which explains backward compatibility guarantees and non-guarantees.
- * @{$programmers_guide/faq$FAQ}, which contains frequently asked
- questions about TensorFlow. (We have not revised this document for v1.3,
- except to remove some obsolete information.)
+ * @{$programmers_guide/faq}, which contains frequently asked
+ questions about TensorFlow.
index.md
+
+### High Level APIs
estimators.md
+datasets.md
+
+### Low Level APIs
+low_level_intro.md
tensors.md
variables.md
graphs.md
saved_model.md
-datasets.md
+using_gpu.md
+
+### ML Concepts
embedding.md
+
+### Debugging
debugger.md
-supervisor.md
+
+### TensorBoard
+summaries_and_tensorboard.md
+graph_viz.md
+tensorboard_histograms.md
+
+### Misc
version_compat.md
faq.md
### Preparing serving inputs
-During training, an @{$input_fn$`input_fn()`} ingests data and prepares it for
-use by the model. At serving time, similarly, a `serving_input_receiver_fn()`
-accepts inference requests and prepares them for the model. This function
-has the following purposes:
+During training, an @{$premade_estimators#input_fn$`input_fn()`} ingests data
+and prepares it for use by the model. At serving time, similarly, a
+`serving_input_receiver_fn()` accepts inference requests and prepares them for
+the model. This function has the following purposes:
* To add placeholders to the graph that the serving system will feed
with inference requests.
--- /dev/null
+# TensorBoard: Visualizing Learning
+
+The computations you'll use TensorFlow for - like training a massive
+deep neural network - can be complex and confusing. To make it easier to
+understand, debug, and optimize TensorFlow programs, we've included a suite of
+visualization tools called TensorBoard. You can use TensorBoard to visualize
+your TensorFlow graph, plot quantitative metrics about the execution of your
+graph, and show additional data like images that pass through it. When
+TensorBoard is fully configured, it looks like this:
+
+
+
+<div class="video-wrapper">
+ <iframe class="devsite-embedded-youtube-video" data-video-id="eBbEDRsCmv4"
+ data-autohide="1" data-showinfo="0" frameborder="0" allowfullscreen>
+ </iframe>
+</div>
+
+This tutorial is intended to get you started with simple TensorBoard usage.
+There are other resources available as well! The [TensorBoard's GitHub](https://github.com/tensorflow/tensorboard)
+has a lot more information on TensorBoard usage, including tips & tricks, and
+debugging information.
+
+## Serializing the data
+
+TensorBoard operates by reading TensorFlow events files, which contain summary
+data that you can generate when running TensorFlow. Here's the general
+lifecycle for summary data within TensorBoard.
+
+First, create the TensorFlow graph that you'd like to collect summary
+data from, and decide which nodes you would like to annotate with
+@{$python/summary$summary operations}.
+
+For example, suppose you are training a convolutional neural network for
+recognizing MNIST digits. You'd like to record how the learning rate
+varies over time, and how the objective function is changing. Collect these by
+attaching @{tf.summary.scalar} ops
+to the nodes that output the learning rate and loss respectively. Then, give
+each `scalar_summary` a meaningful `tag`, like `'learning rate'` or `'loss
+function'`.
+
+Perhaps you'd also like to visualize the distributions of activations coming
+off a particular layer, or the distribution of gradients or weights. Collect
+this data by attaching
+@{tf.summary.histogram} ops to
+the gradient outputs and to the variable that holds your weights, respectively.
+
+For details on all of the summary operations available, check out the docs on
+@{$python/summary$summary operations}.
+
+Operations in TensorFlow don't do anything until you run them, or an op that
+depends on their output. And the summary nodes that we've just created are
+peripheral to your graph: none of the ops you are currently running depend on
+them. So, to generate summaries, we need to run all of these summary nodes.
+Managing them by hand would be tedious, so use
+@{tf.summary.merge_all}
+to combine them into a single op that generates all the summary data.
+
+Then, you can just run the merged summary op, which will generate a serialized
+`Summary` protobuf object with all of your summary data at a given step.
+Finally, to write this summary data to disk, pass the summary protobuf to a
+@{tf.summary.FileWriter}.
+
+The `FileWriter` takes a logdir in its constructor - this logdir is quite
+important, it's the directory where all of the events will be written out.
+Also, the `FileWriter` can optionally take a `Graph` in its constructor.
+If it receives a `Graph` object, then TensorBoard will visualize your graph
+along with tensor shape information. This will give you a much better sense of
+what flows through the graph: see
+@{$graph_viz#tensor-shape-information$Tensor shape information}.
+
+Now that you've modified your graph and have a `FileWriter`, you're ready to
+start running your network! If you want, you could run the merged summary op
+every single step, and record a ton of training data. That's likely to be more
+data than you need, though. Instead, consider running the merged summary op
+every `n` steps.
+
+The code example below is a modification of the
+@{$layers$simple MNIST tutorial},
+in which we have added some summary ops, and run them every ten steps. If you
+run this and then launch `tensorboard --logdir=/tmp/tensorflow/mnist`, you'll be able
+to visualize statistics, such as how the weights or accuracy varied during
+training. The code below is an excerpt; full source is
+[here](https://www.tensorflow.org/code/tensorflow/examples/tutorials/mnist/mnist_with_summaries.py).
+
+```python
+def variable_summaries(var):
+ """Attach a lot of summaries to a Tensor (for TensorBoard visualization)."""
+ with tf.name_scope('summaries'):
+ mean = tf.reduce_mean(var)
+ tf.summary.scalar('mean', mean)
+ with tf.name_scope('stddev'):
+ stddev = tf.sqrt(tf.reduce_mean(tf.square(var - mean)))
+ tf.summary.scalar('stddev', stddev)
+ tf.summary.scalar('max', tf.reduce_max(var))
+ tf.summary.scalar('min', tf.reduce_min(var))
+ tf.summary.histogram('histogram', var)
+
+def nn_layer(input_tensor, input_dim, output_dim, layer_name, act=tf.nn.relu):
+ """Reusable code for making a simple neural net layer.
+
+ It does a matrix multiply, bias add, and then uses relu to nonlinearize.
+ It also sets up name scoping so that the resultant graph is easy to read,
+ and adds a number of summary ops.
+ """
+ # Adding a name scope ensures logical grouping of the layers in the graph.
+ with tf.name_scope(layer_name):
+ # This Variable will hold the state of the weights for the layer
+ with tf.name_scope('weights'):
+ weights = weight_variable([input_dim, output_dim])
+ variable_summaries(weights)
+ with tf.name_scope('biases'):
+ biases = bias_variable([output_dim])
+ variable_summaries(biases)
+ with tf.name_scope('Wx_plus_b'):
+ preactivate = tf.matmul(input_tensor, weights) + biases
+ tf.summary.histogram('pre_activations', preactivate)
+ activations = act(preactivate, name='activation')
+ tf.summary.histogram('activations', activations)
+ return activations
+
+hidden1 = nn_layer(x, 784, 500, 'layer1')
+
+with tf.name_scope('dropout'):
+ keep_prob = tf.placeholder(tf.float32)
+ tf.summary.scalar('dropout_keep_probability', keep_prob)
+ dropped = tf.nn.dropout(hidden1, keep_prob)
+
+# Do not apply softmax activation yet, see below.
+y = nn_layer(dropped, 500, 10, 'layer2', act=tf.identity)
+
+with tf.name_scope('cross_entropy'):
+ # The raw formulation of cross-entropy,
+ #
+ # tf.reduce_mean(-tf.reduce_sum(y_ * tf.log(tf.softmax(y)),
+ # reduction_indices=[1]))
+ #
+ # can be numerically unstable.
+ #
+ # So here we use tf.losses.sparse_softmax_cross_entropy on the
+ # raw logit outputs of the nn_layer above.
+ with tf.name_scope('total'):
+ cross_entropy = tf.losses.sparse_softmax_cross_entropy(labels=y_, logits=y)
+tf.summary.scalar('cross_entropy', cross_entropy)
+
+with tf.name_scope('train'):
+ train_step = tf.train.AdamOptimizer(FLAGS.learning_rate).minimize(
+ cross_entropy)
+
+with tf.name_scope('accuracy'):
+ with tf.name_scope('correct_prediction'):
+ correct_prediction = tf.equal(tf.argmax(y, 1), tf.argmax(y_, 1))
+ with tf.name_scope('accuracy'):
+ accuracy = tf.reduce_mean(tf.cast(correct_prediction, tf.float32))
+tf.summary.scalar('accuracy', accuracy)
+
+# Merge all the summaries and write them out to /tmp/mnist_logs (by default)
+merged = tf.summary.merge_all()
+train_writer = tf.summary.FileWriter(FLAGS.summaries_dir + '/train',
+ sess.graph)
+test_writer = tf.summary.FileWriter(FLAGS.summaries_dir + '/test')
+tf.global_variables_initializer().run()
+```
+
+After we've initialized the `FileWriters`, we have to add summaries to the
+`FileWriters` as we train and test the model.
+
+```python
+# Train the model, and also write summaries.
+# Every 10th step, measure test-set accuracy, and write test summaries
+# All other steps, run train_step on training data, & add training summaries
+
+def feed_dict(train):
+ """Make a TensorFlow feed_dict: maps data onto Tensor placeholders."""
+ if train or FLAGS.fake_data:
+ xs, ys = mnist.train.next_batch(100, fake_data=FLAGS.fake_data)
+ k = FLAGS.dropout
+ else:
+ xs, ys = mnist.test.images, mnist.test.labels
+ k = 1.0
+ return {x: xs, y_: ys, keep_prob: k}
+
+for i in range(FLAGS.max_steps):
+ if i % 10 == 0: # Record summaries and test-set accuracy
+ summary, acc = sess.run([merged, accuracy], feed_dict=feed_dict(False))
+ test_writer.add_summary(summary, i)
+ print('Accuracy at step %s: %s' % (i, acc))
+ else: # Record train set summaries, and train
+ summary, _ = sess.run([merged, train_step], feed_dict=feed_dict(True))
+ train_writer.add_summary(summary, i)
+```
+
+You're now all set to visualize this data using TensorBoard.
+
+
+## Launching TensorBoard
+
+To run TensorBoard, use the following command (alternatively `python -m
+tensorboard.main`)
+
+```bash
+tensorboard --logdir=path/to/log-directory
+```
+
+where `logdir` points to the directory where the `FileWriter` serialized its
+data. If this `logdir` directory contains subdirectories which contain
+serialized data from separate runs, then TensorBoard will visualize the data
+from all of those runs. Once TensorBoard is running, navigate your web browser
+to `localhost:6006` to view the TensorBoard.
+
+When looking at TensorBoard, you will see the navigation tabs in the top right
+corner. Each tab represents a set of serialized data that can be visualized.
+
+For in depth information on how to use the *graph* tab to visualize your graph,
+see @{$graph_viz$TensorBoard: Graph Visualization}.
+
+For more usage information on TensorBoard in general, see the [TensorBoard's GitHub](https://github.com/tensorflow/tensorboard).
--- /dev/null
+# TensorBoard Histogram Dashboard
+
+The TensorBoard Histogram Dashboard displays how the distribution of some
+`Tensor` in your TensorFlow graph has changed over time. It does this by showing
+many histograms visualizations of your tensor at different points in time.
+
+## A Basic Example
+
+Let's start with a simple case: a normally-distributed variable, where the mean
+shifts over time.
+TensorFlow has an op
+[`tf.random_normal`](https://www.tensorflow.org/api_docs/python/tf/random_normal)
+which is perfect for this purpose. As is usually the case with TensorBoard, we
+will ingest data using a summary op; in this case,
+['tf.summary.histogram'](https://www.tensorflow.org/api_docs/python/tf/summary/histogram).
+For a primer on how summaries work, please see the general
+[TensorBoard tutorial](https://www.tensorflow.org/get_started/summaries_and_tensorboard).
+
+Here is a code snippet that will generate some histogram summaries containing
+normally distributed data, where the mean of the distribution increases over
+time.
+
+```python
+import tensorflow as tf
+
+k = tf.placeholder(tf.float32)
+
+# Make a normal distribution, with a shifting mean
+mean_moving_normal = tf.random_normal(shape=[1000], mean=(5*k), stddev=1)
+# Record that distribution into a histogram summary
+tf.summary.histogram("normal/moving_mean", mean_moving_normal)
+
+# Setup a session and summary writer
+sess = tf.Session()
+writer = tf.summary.FileWriter("/tmp/histogram_example")
+
+summaries = tf.summary.merge_all()
+
+# Setup a loop and write the summaries to disk
+N = 400
+for step in range(N):
+ k_val = step/float(N)
+ summ = sess.run(summaries, feed_dict={k: k_val})
+ writer.add_summary(summ, global_step=step)
+```
+
+Once that code runs, we can load the data into TensorBoard via the command line:
+
+
+```sh
+tensorboard --logdir=/tmp/histogram_example
+```
+
+Once TensorBoard is running, load it in Chrome or Firefox and navigate to the
+Histogram Dashboard. Then we can see a histogram visualization for our normally
+distributed data.
+
+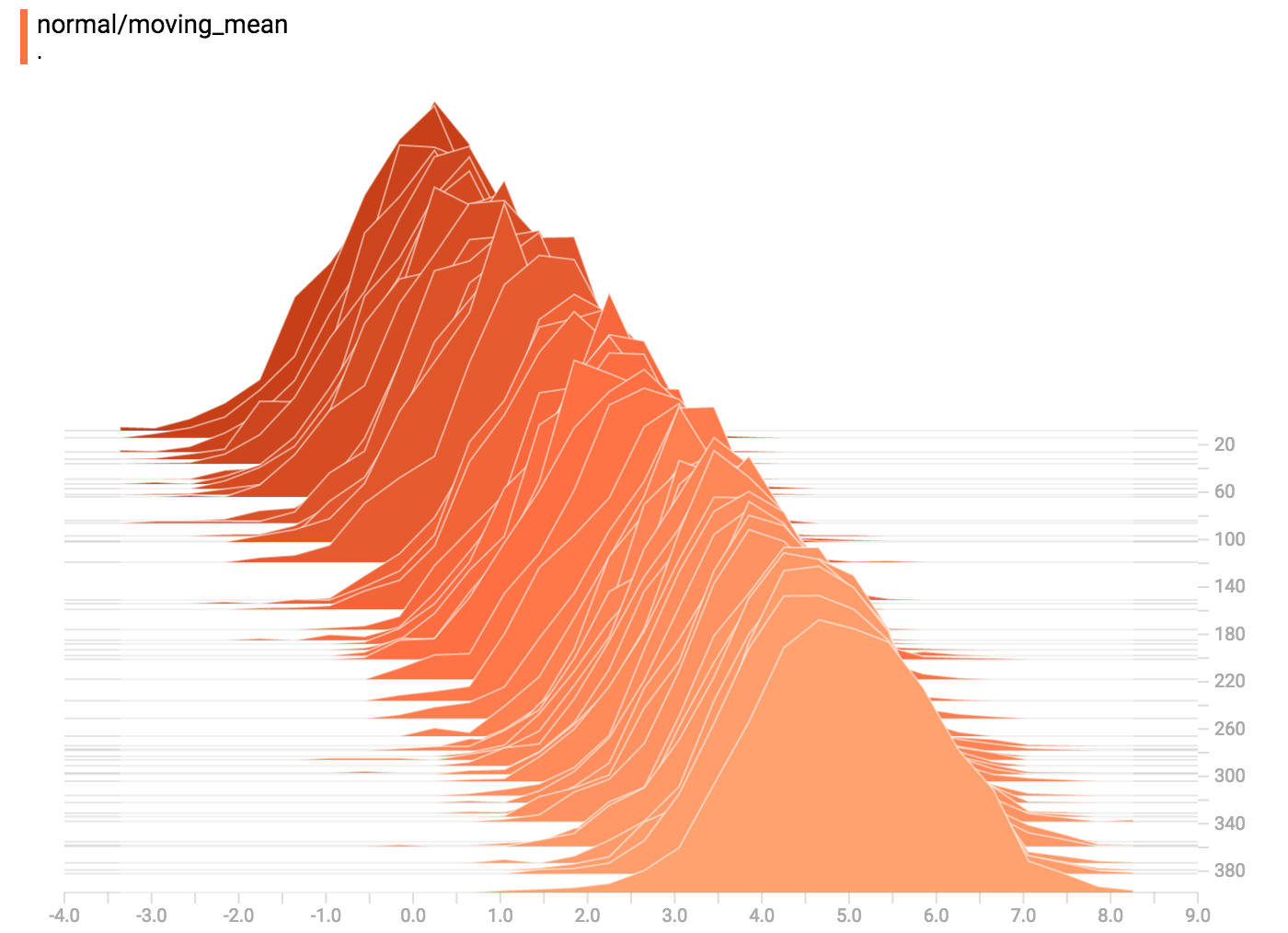
+
+`tf.summary.histogram` takes an arbitrarily sized and shaped Tensor, and
+compresses it into a histogram data structure consisting of many bins with
+widths and counts. For example, let's say we want to organize the numbers
+`[0.5, 1.1, 1.3, 2.2, 2.9, 2.99]` into bins. We could make three bins:
+* a bin
+containing everything from 0 to 1 (it would contain one element, 0.5),
+* a bin
+containing everything from 1-2 (it would contain two elements, 1.1 and 1.3),
+* a bin containing everything from 2-3 (it would contain three elements: 2.2,
+2.9 and 2.99).
+
+TensorFlow uses a similar approach to create bins, but unlike in our example, it
+doesn't create integer bins. For large, sparse datasets, that might result in
+many thousands of bins.
+Instead, [the bins are exponentially distributed, with many bins close to 0 and
+comparatively few bins for very large numbers.](https://github.com/tensorflow/tensorflow/blob/c8b59c046895fa5b6d79f73e0b5817330fcfbfc1/tensorflow/core/lib/histogram/histogram.cc#L28)
+However, visualizing exponentially-distributed bins is tricky; if height is used
+to encode count, then wider bins take more space, even if they have the same
+number of elements. Conversely, encoding count in the area makes height
+comparisons impossible. Instead, the histograms [resample the data](https://github.com/tensorflow/tensorflow/blob/17c47804b86e340203d451125a721310033710f1/tensorflow/tensorboard/components/tf_backend/backend.ts#L400)
+into uniform bins. This can lead to unfortunate artifacts in some cases.
+
+Each slice in the histogram visualizer displays a single histogram.
+The slices are organized by step;
+older slices (e.g. step 0) are further "back" and darker, while newer slices
+(e.g. step 400) are close to the foreground, and lighter in color.
+The y-axis on the right shows the step number.
+
+You can mouse over the histogram to see tooltips with some more detailed
+information. For example, in the following image we can see that the histogram
+at timestep 176 has a bin centered at 2.25 with 177 elements in that bin.
+
+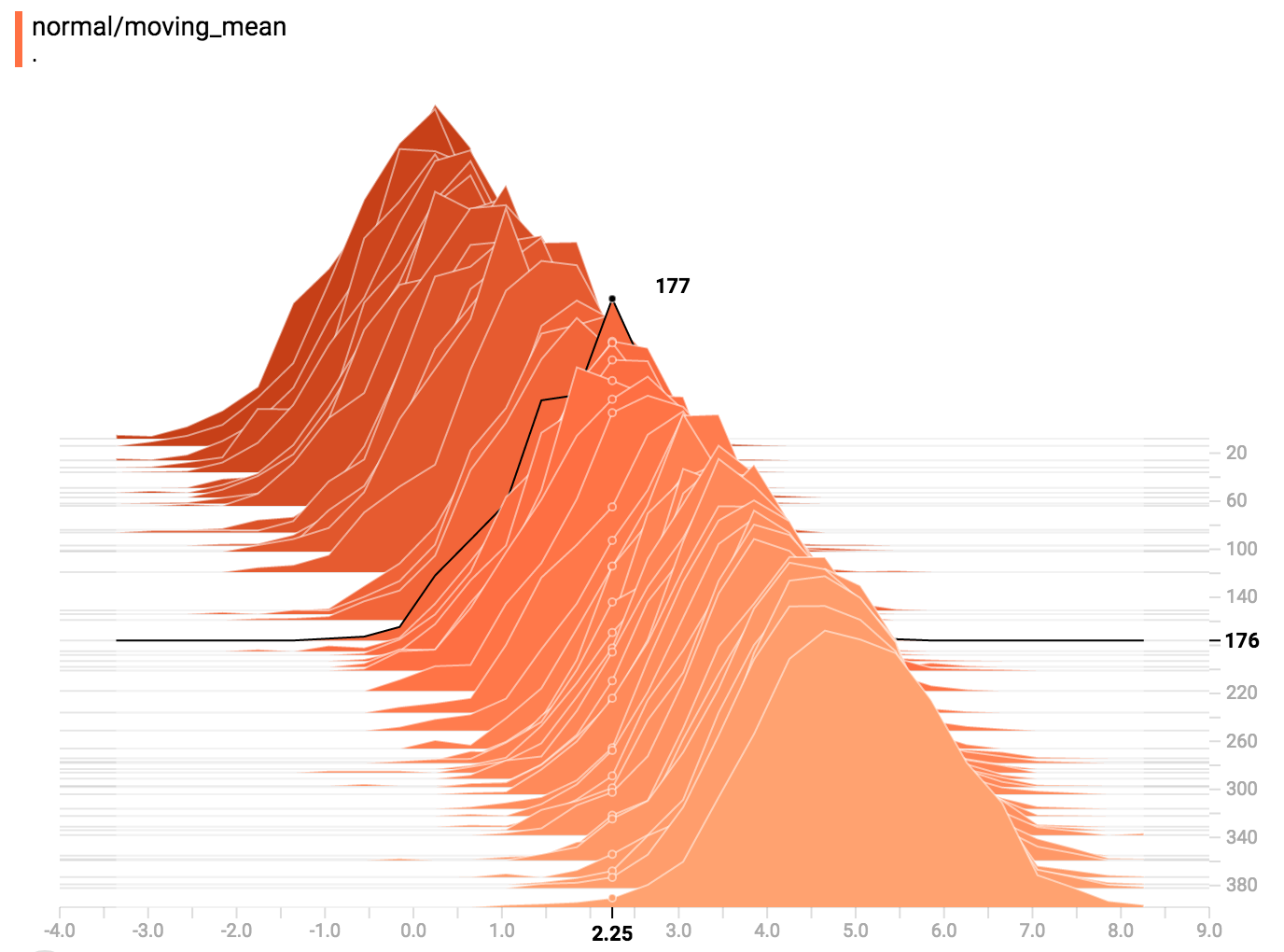
+
+Also, you may note that the histogram slices are not always evenly spaced in
+step count or time. This is because TensorBoard uses
+[reservoir sampling](https://en.wikipedia.org/wiki/Reservoir_sampling) to keep a
+subset of all the histograms, to save on memory. Reservoir sampling guarantees
+that every sample has an equal likelihood of being included, but because it is
+a randomized algorithm, the samples chosen don't occur at even steps.
+
+## Overlay Mode
+
+There is a control on the left of the dashboard that allows you to toggle the
+histogram mode from "offset" to "overlay":
+
+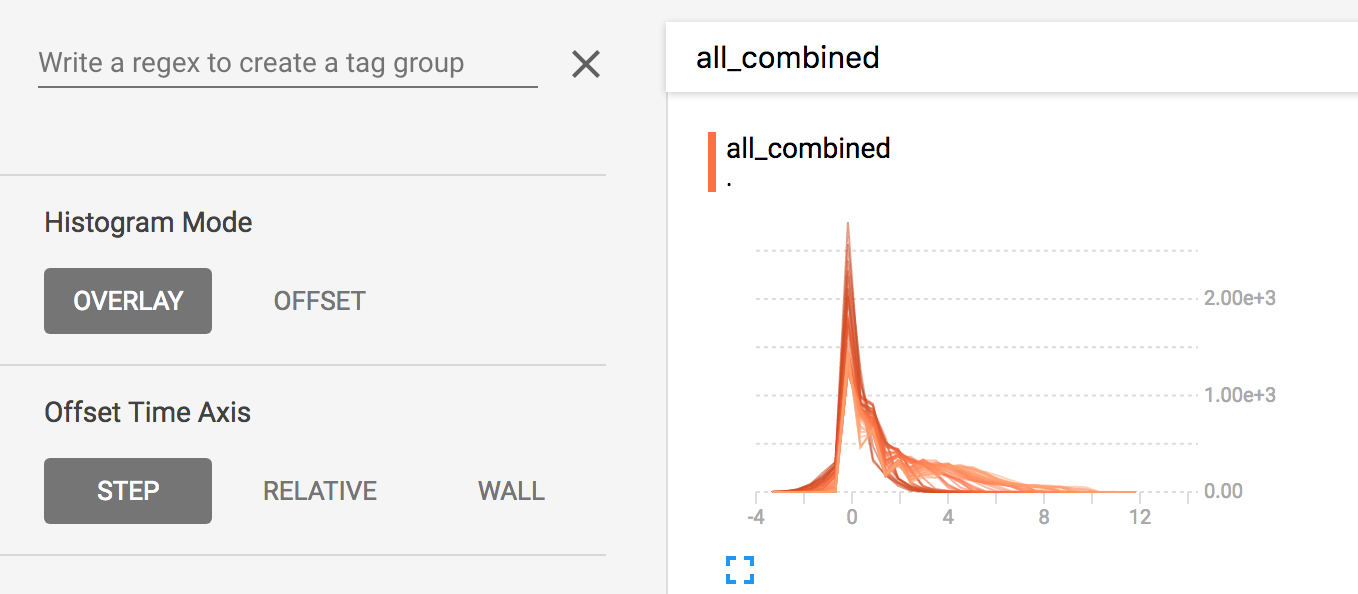
+
+In "offset" mode, the visualization rotates 45 degrees, so that the individual
+histogram slices are no longer spread out in time, but instead are all plotted
+on the same y-axis.
+
+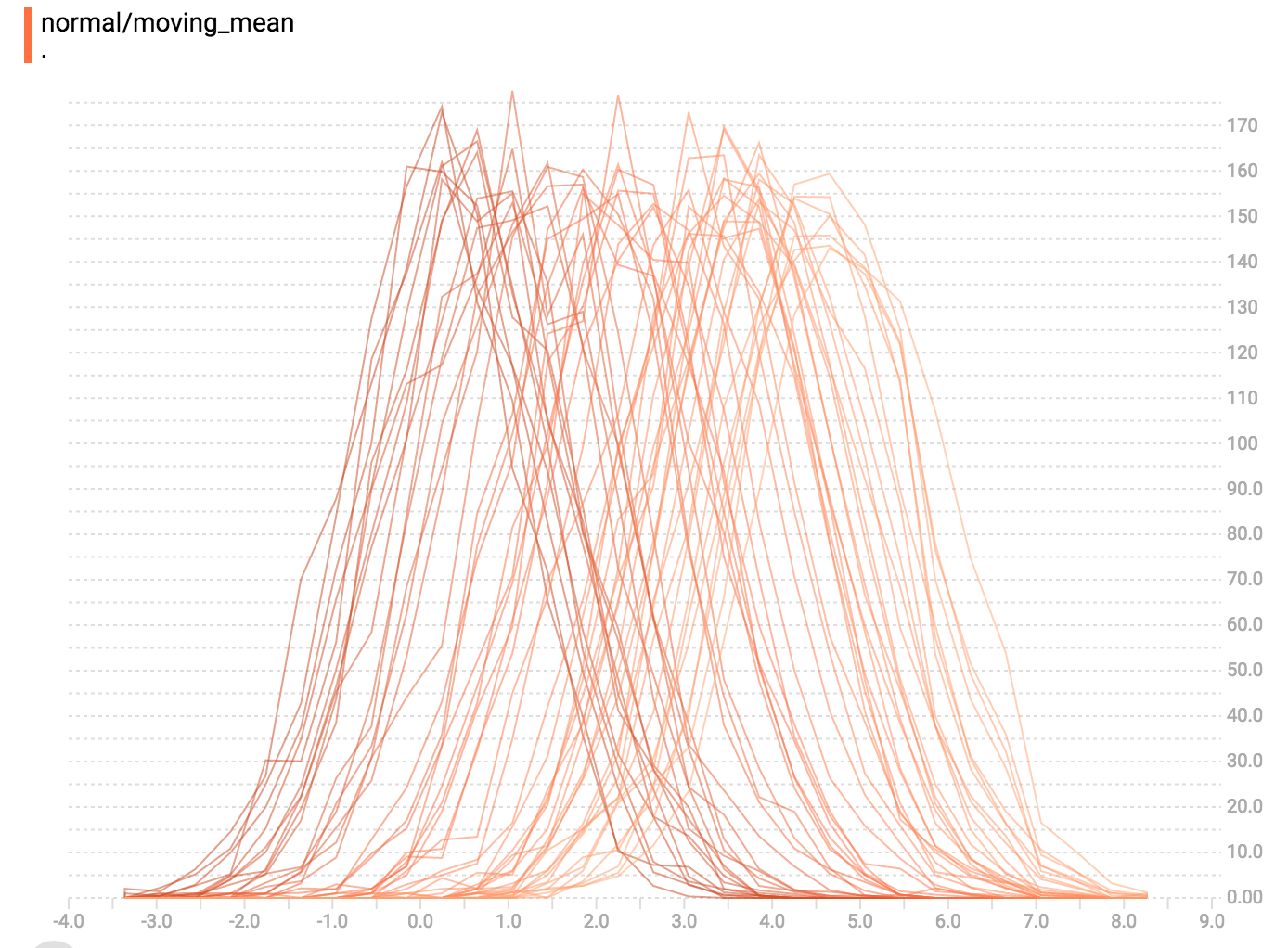
+Now, each slice is a separate line on the chart, and the y-axis shows the item
+count within each bucket. Darker lines are older, earlier steps, and lighter
+lines are more recent, later steps. Once again, you can mouse over the chart to
+see some additional information.
+
+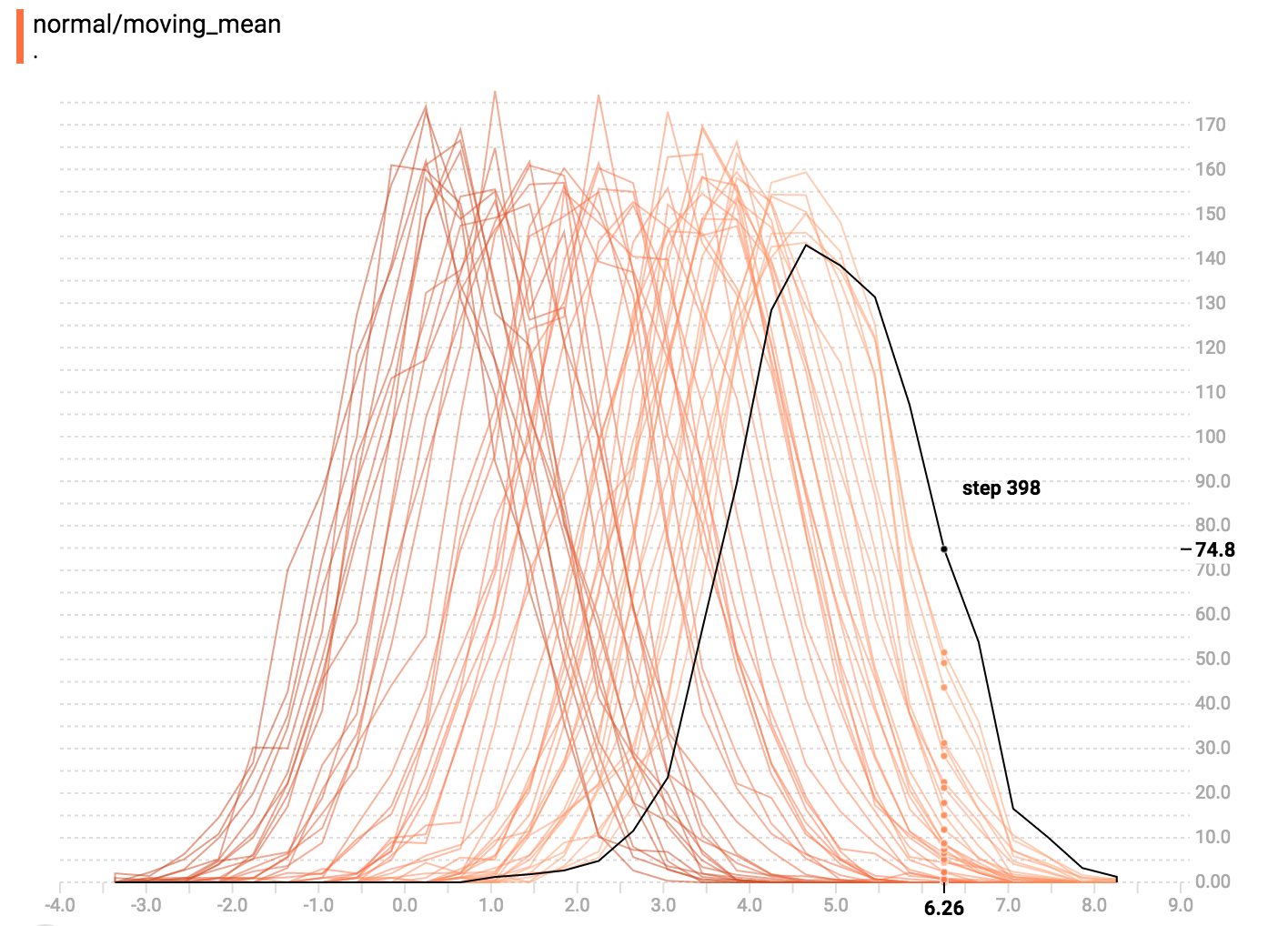
+
+In general, the overlay visualization is useful if you want to directly compare
+the counts of different histograms.
+
+## Multimodal Distributions
+
+The Histogram Dashboard is great for visualizing multimodal
+distributions. Let's construct a simple bimodal distribution by concatenating
+the outputs from two different normal distributions. The code will look like
+this:
+
+```python
+import tensorflow as tf
+
+k = tf.placeholder(tf.float32)
+
+# Make a normal distribution, with a shifting mean
+mean_moving_normal = tf.random_normal(shape=[1000], mean=(5*k), stddev=1)
+# Record that distribution into a histogram summary
+tf.summary.histogram("normal/moving_mean", mean_moving_normal)
+
+# Make a normal distribution with shrinking variance
+variance_shrinking_normal = tf.random_normal(shape=[1000], mean=0, stddev=1-(k))
+# Record that distribution too
+tf.summary.histogram("normal/shrinking_variance", variance_shrinking_normal)
+
+# Let's combine both of those distributions into one dataset
+normal_combined = tf.concat([mean_moving_normal, variance_shrinking_normal], 0)
+# We add another histogram summary to record the combined distribution
+tf.summary.histogram("normal/bimodal", normal_combined)
+
+summaries = tf.summary.merge_all()
+
+# Setup a session and summary writer
+sess = tf.Session()
+writer = tf.summary.FileWriter("/tmp/histogram_example")
+
+# Setup a loop and write the summaries to disk
+N = 400
+for step in range(N):
+ k_val = step/float(N)
+ summ = sess.run(summaries, feed_dict={k: k_val})
+ writer.add_summary(summ, global_step=step)
+```
+
+You already remember our "moving mean" normal distribution from the example
+above. Now we also have a "shrinking variance" distribution. Side-by-side, they
+look like this:
+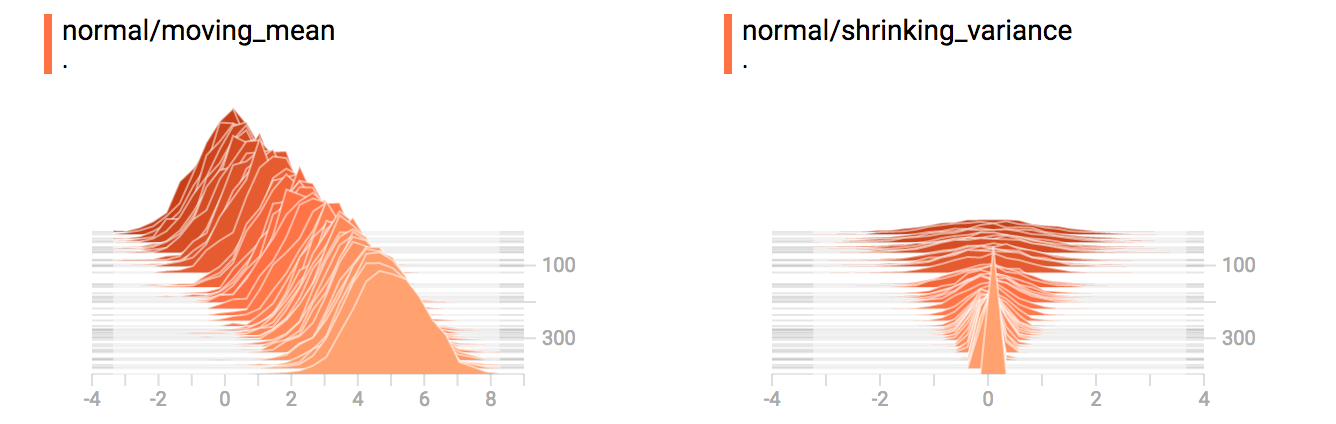
+
+When we concatenate them, we get a chart that clearly reveals the divergent,
+bimodal structure:
+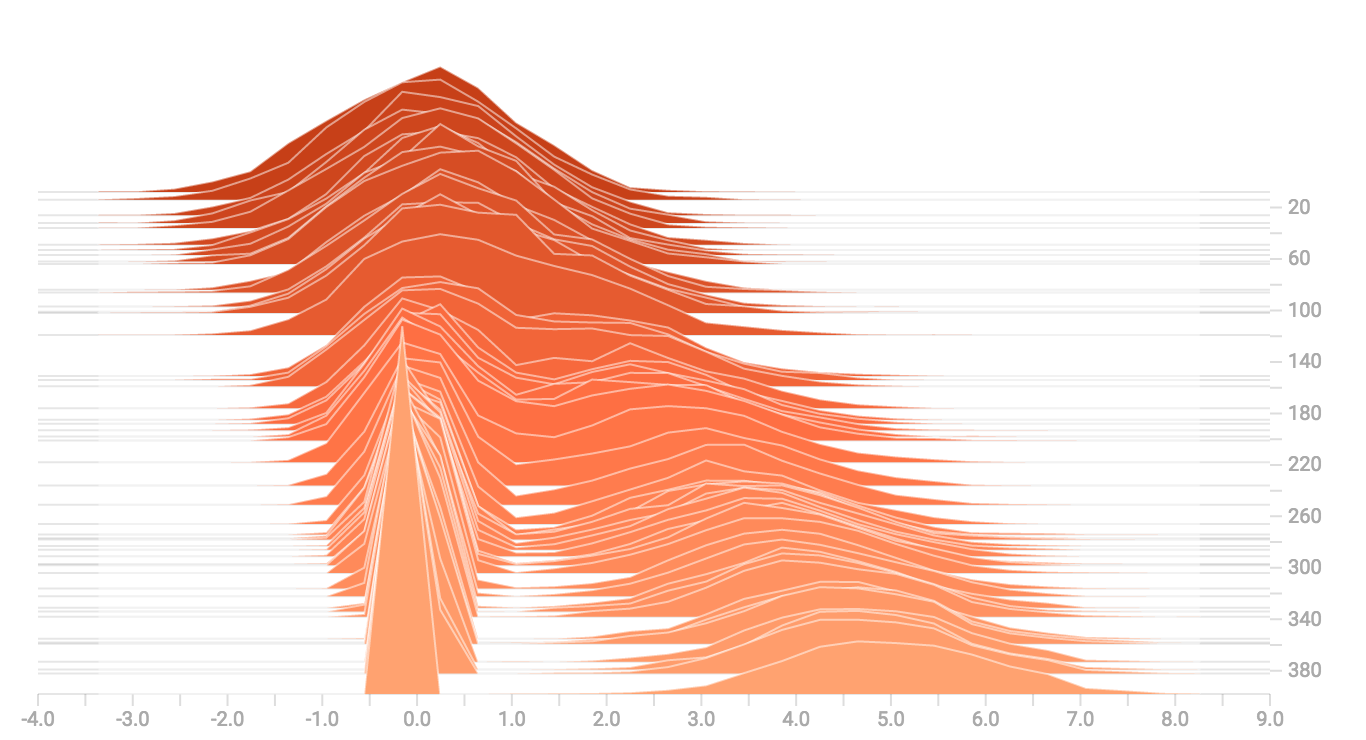
+
+## Some more distributions
+
+Just for fun, let's generate and visualize a few more distributions, and then
+combine them all into one chart. Here's the code we'll use:
+
+```python
+import tensorflow as tf
+
+k = tf.placeholder(tf.float32)
+
+# Make a normal distribution, with a shifting mean
+mean_moving_normal = tf.random_normal(shape=[1000], mean=(5*k), stddev=1)
+# Record that distribution into a histogram summary
+tf.summary.histogram("normal/moving_mean", mean_moving_normal)
+
+# Make a normal distribution with shrinking variance
+variance_shrinking_normal = tf.random_normal(shape=[1000], mean=0, stddev=1-(k))
+# Record that distribution too
+tf.summary.histogram("normal/shrinking_variance", variance_shrinking_normal)
+
+# Let's combine both of those distributions into one dataset
+normal_combined = tf.concat([mean_moving_normal, variance_shrinking_normal], 0)
+# We add another histogram summary to record the combined distribution
+tf.summary.histogram("normal/bimodal", normal_combined)
+
+# Add a gamma distribution
+gamma = tf.random_gamma(shape=[1000], alpha=k)
+tf.summary.histogram("gamma", gamma)
+
+# And a poisson distribution
+poisson = tf.random_poisson(shape=[1000], lam=k)
+tf.summary.histogram("poisson", poisson)
+
+# And a uniform distribution
+uniform = tf.random_uniform(shape=[1000], maxval=k*10)
+tf.summary.histogram("uniform", uniform)
+
+# Finally, combine everything together!
+all_distributions = [mean_moving_normal, variance_shrinking_normal,
+ gamma, poisson, uniform]
+all_combined = tf.concat(all_distributions, 0)
+tf.summary.histogram("all_combined", all_combined)
+
+summaries = tf.summary.merge_all()
+
+# Setup a session and summary writer
+sess = tf.Session()
+writer = tf.summary.FileWriter("/tmp/histogram_example")
+
+# Setup a loop and write the summaries to disk
+N = 400
+for step in range(N):
+ k_val = step/float(N)
+ summ = sess.run(summaries, feed_dict={k: k_val})
+ writer.add_summary(summ, global_step=step)
+```
+### Gamma Distribution
+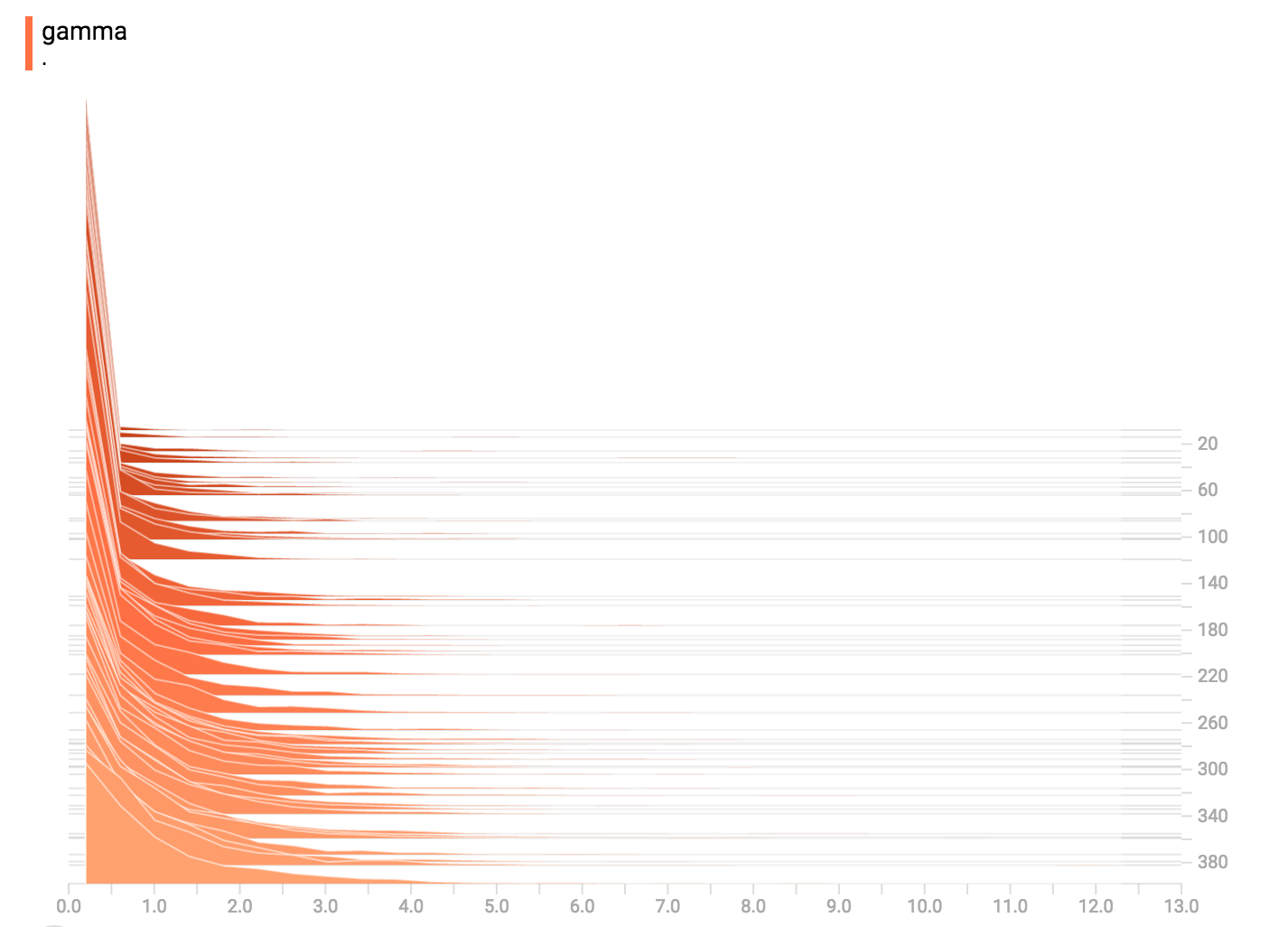
+
+### Uniform Distribution
+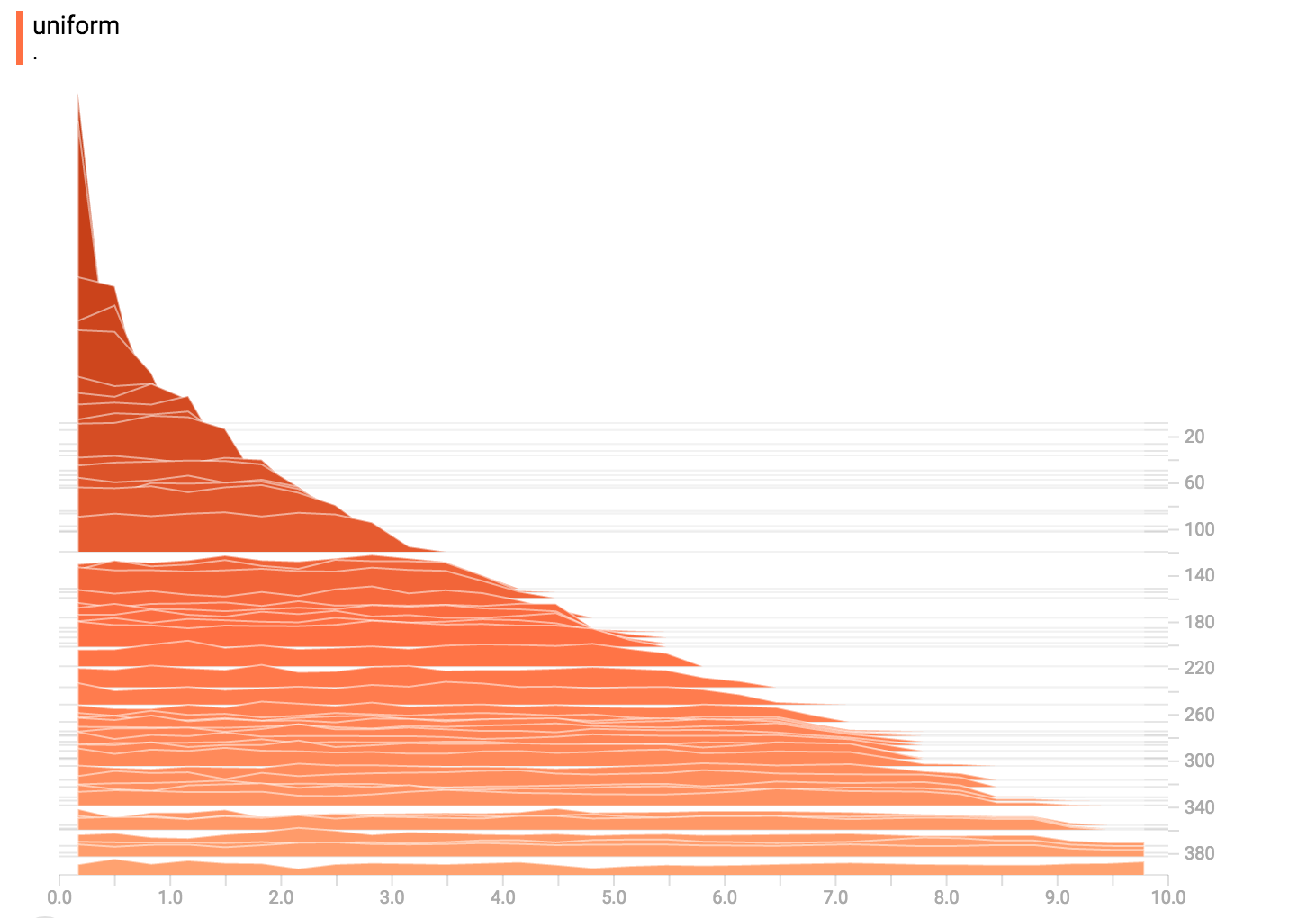
+
+### Poisson Distribution
+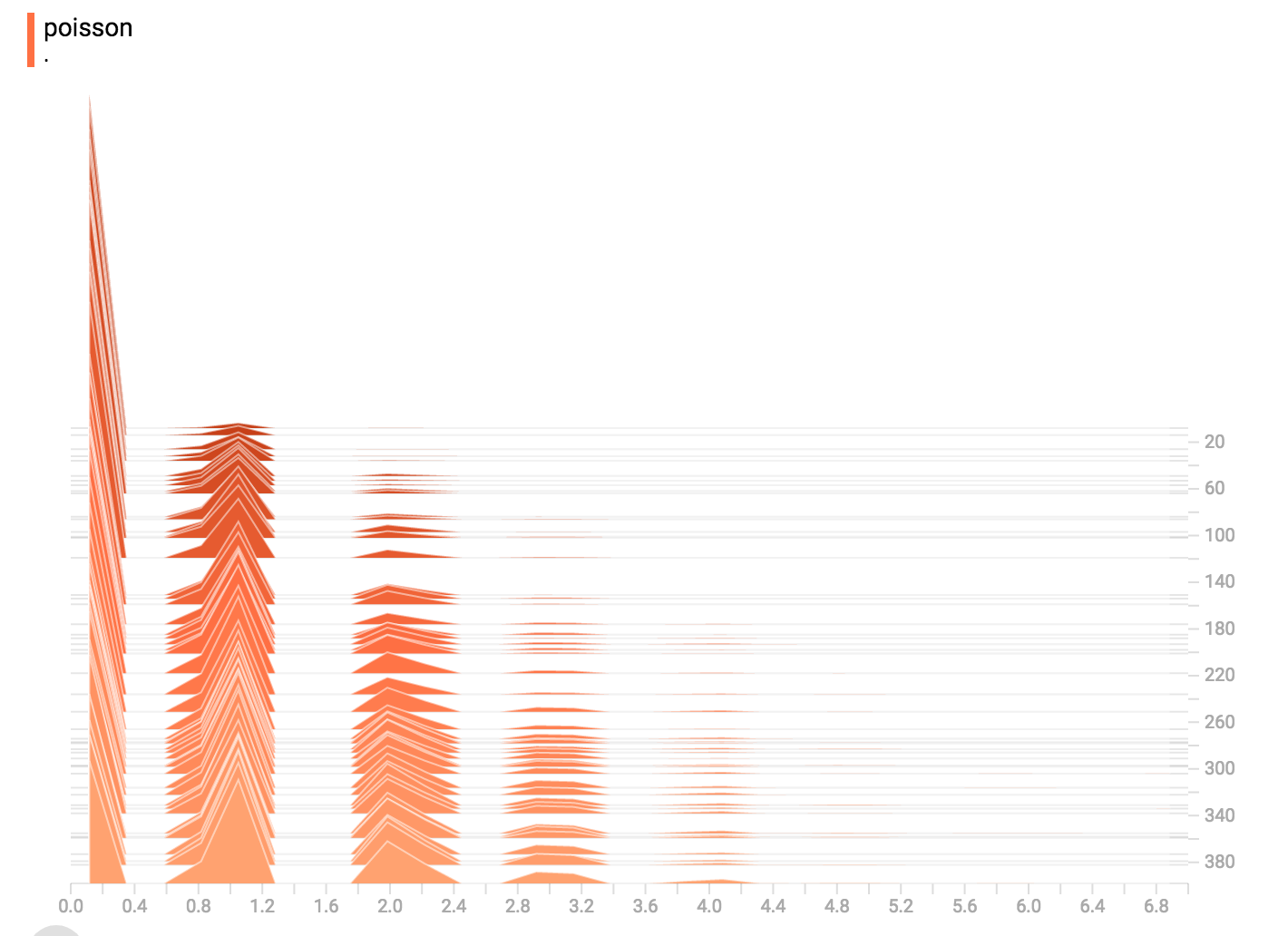
+The poisson distribution is defined over the integers. So, all of the values
+being generated are perfect integers. The histogram compression moves the data
+into floating-point bins, causing the visualization to show little
+bumps over the integer values rather than perfect spikes.
+
+### All Together Now
+Finally, we can concatenate all of the data into one funny-looking curve.
+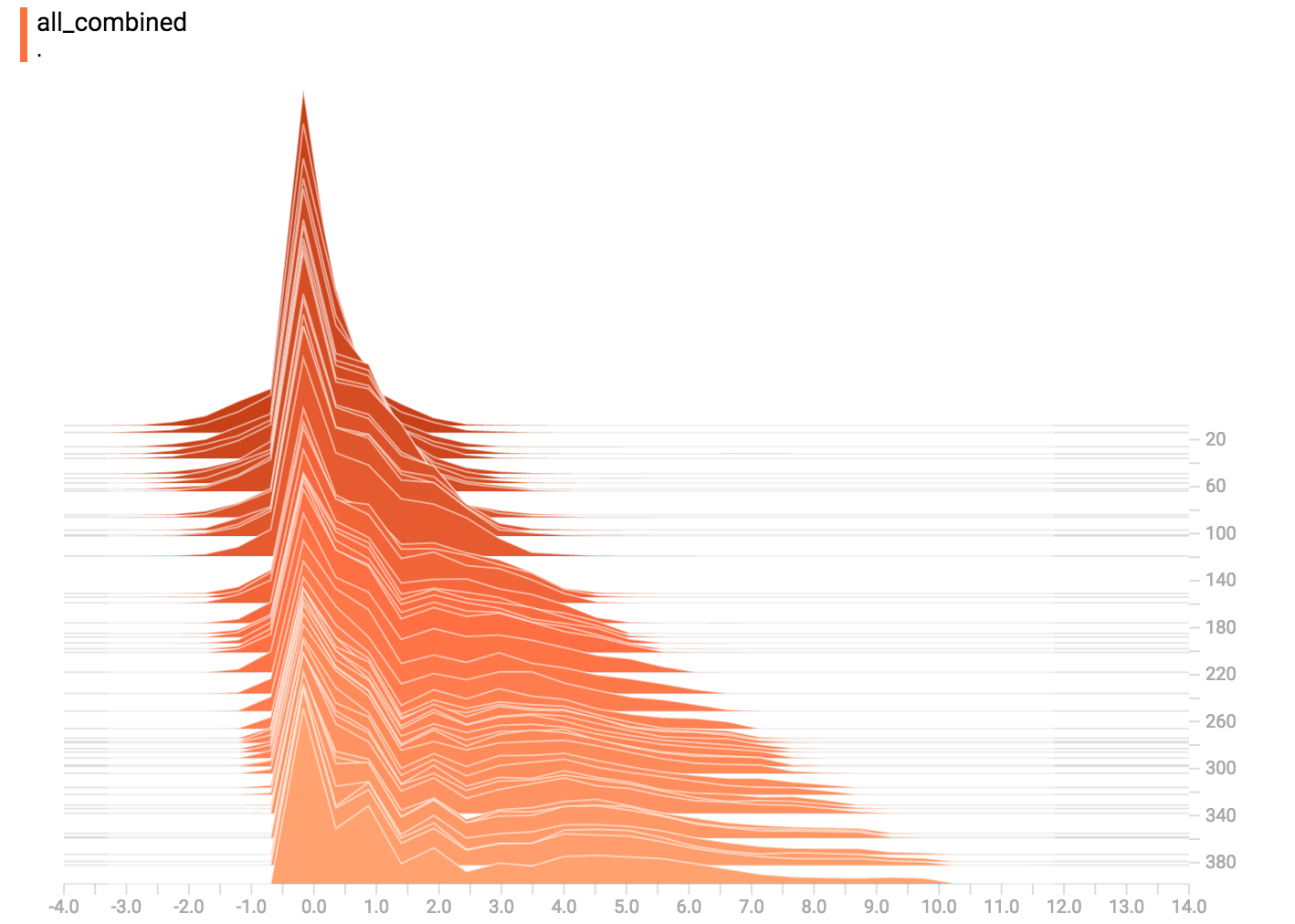
+
--- /dev/null
+# Using GPUs
+
+## Supported devices
+
+On a typical system, there are multiple computing devices. In TensorFlow, the
+supported device types are `CPU` and `GPU`. They are represented as `strings`.
+For example:
+
+* `"/cpu:0"`: The CPU of your machine.
+* `"/device:GPU:0"`: The GPU of your machine, if you have one.
+* `"/device:GPU:1"`: The second GPU of your machine, etc.
+
+If a TensorFlow operation has both CPU and GPU implementations, the GPU devices
+will be given priority when the operation is assigned to a device. For example,
+`matmul` has both CPU and GPU kernels. On a system with devices `cpu:0` and
+`gpu:0`, `gpu:0` will be selected to run `matmul`.
+
+## Logging Device placement
+
+To find out which devices your operations and tensors are assigned to, create
+the session with `log_device_placement` configuration option set to `True`.
+
+```python
+# Creates a graph.
+a = tf.constant([1.0, 2.0, 3.0, 4.0, 5.0, 6.0], shape=[2, 3], name='a')
+b = tf.constant([1.0, 2.0, 3.0, 4.0, 5.0, 6.0], shape=[3, 2], name='b')
+c = tf.matmul(a, b)
+# Creates a session with log_device_placement set to True.
+sess = tf.Session(config=tf.ConfigProto(log_device_placement=True))
+# Runs the op.
+print(sess.run(c))
+```
+
+You should see the following output:
+
+```
+Device mapping:
+/job:localhost/replica:0/task:0/device:GPU:0 -> device: 0, name: Tesla K40c, pci bus
+id: 0000:05:00.0
+b: /job:localhost/replica:0/task:0/device:GPU:0
+a: /job:localhost/replica:0/task:0/device:GPU:0
+MatMul: /job:localhost/replica:0/task:0/device:GPU:0
+[[ 22. 28.]
+ [ 49. 64.]]
+
+```
+
+## Manual device placement
+
+If you would like a particular operation to run on a device of your choice
+instead of what's automatically selected for you, you can use `with tf.device`
+to create a device context such that all the operations within that context will
+have the same device assignment.
+
+```python
+# Creates a graph.
+with tf.device('/cpu:0'):
+ a = tf.constant([1.0, 2.0, 3.0, 4.0, 5.0, 6.0], shape=[2, 3], name='a')
+ b = tf.constant([1.0, 2.0, 3.0, 4.0, 5.0, 6.0], shape=[3, 2], name='b')
+c = tf.matmul(a, b)
+# Creates a session with log_device_placement set to True.
+sess = tf.Session(config=tf.ConfigProto(log_device_placement=True))
+# Runs the op.
+print(sess.run(c))
+```
+
+You will see that now `a` and `b` are assigned to `cpu:0`. Since a device was
+not explicitly specified for the `MatMul` operation, the TensorFlow runtime will
+choose one based on the operation and available devices (`gpu:0` in this
+example) and automatically copy tensors between devices if required.
+
+```
+Device mapping:
+/job:localhost/replica:0/task:0/device:GPU:0 -> device: 0, name: Tesla K40c, pci bus
+id: 0000:05:00.0
+b: /job:localhost/replica:0/task:0/cpu:0
+a: /job:localhost/replica:0/task:0/cpu:0
+MatMul: /job:localhost/replica:0/task:0/device:GPU:0
+[[ 22. 28.]
+ [ 49. 64.]]
+```
+
+## Allowing GPU memory growth
+
+By default, TensorFlow maps nearly all of the GPU memory of all GPUs (subject to
+[`CUDA_VISIBLE_DEVICES`](https://docs.nvidia.com/cuda/cuda-c-programming-guide/index.html#env-vars))
+visible to the process. This is done to more efficiently use the relatively
+precious GPU memory resources on the devices by reducing [memory
+fragmentation](https://en.wikipedia.org/wiki/Fragmentation_\(computing\)).
+
+In some cases it is desirable for the process to only allocate a subset of the
+available memory, or to only grow the memory usage as is needed by the process.
+TensorFlow provides two Config options on the Session to control this.
+
+The first is the `allow_growth` option, which attempts to allocate only as much
+GPU memory based on runtime allocations: it starts out allocating very little
+memory, and as Sessions get run and more GPU memory is needed, we extend the GPU
+memory region needed by the TensorFlow process. Note that we do not release
+memory, since that can lead to even worse memory fragmentation. To turn this
+option on, set the option in the ConfigProto by:
+
+```python
+config = tf.ConfigProto()
+config.gpu_options.allow_growth = True
+session = tf.Session(config=config, ...)
+```
+
+The second method is the `per_process_gpu_memory_fraction` option, which
+determines the fraction of the overall amount of memory that each visible GPU
+should be allocated. For example, you can tell TensorFlow to only allocate 40%
+of the total memory of each GPU by:
+
+```python
+config = tf.ConfigProto()
+config.gpu_options.per_process_gpu_memory_fraction = 0.4
+session = tf.Session(config=config, ...)
+```
+
+This is useful if you want to truly bound the amount of GPU memory available to
+the TensorFlow process.
+
+## Using a single GPU on a multi-GPU system
+
+If you have more than one GPU in your system, the GPU with the lowest ID will be
+selected by default. If you would like to run on a different GPU, you will need
+to specify the preference explicitly:
+
+```python
+# Creates a graph.
+with tf.device('/device:GPU:2'):
+ a = tf.constant([1.0, 2.0, 3.0, 4.0, 5.0, 6.0], shape=[2, 3], name='a')
+ b = tf.constant([1.0, 2.0, 3.0, 4.0, 5.0, 6.0], shape=[3, 2], name='b')
+ c = tf.matmul(a, b)
+# Creates a session with log_device_placement set to True.
+sess = tf.Session(config=tf.ConfigProto(log_device_placement=True))
+# Runs the op.
+print(sess.run(c))
+```
+
+If the device you have specified does not exist, you will get
+`InvalidArgumentError`:
+
+```
+InvalidArgumentError: Invalid argument: Cannot assign a device to node 'b':
+Could not satisfy explicit device specification '/device:GPU:2'
+ [[Node: b = Const[dtype=DT_FLOAT, value=Tensor<type: float shape: [3,2]
+ values: 1 2 3...>, _device="/device:GPU:2"]()]]
+```
+
+If you would like TensorFlow to automatically choose an existing and supported
+device to run the operations in case the specified one doesn't exist, you can
+set `allow_soft_placement` to `True` in the configuration option when creating
+the session.
+
+```python
+# Creates a graph.
+with tf.device('/device:GPU:2'):
+ a = tf.constant([1.0, 2.0, 3.0, 4.0, 5.0, 6.0], shape=[2, 3], name='a')
+ b = tf.constant([1.0, 2.0, 3.0, 4.0, 5.0, 6.0], shape=[3, 2], name='b')
+ c = tf.matmul(a, b)
+# Creates a session with allow_soft_placement and log_device_placement set
+# to True.
+sess = tf.Session(config=tf.ConfigProto(
+ allow_soft_placement=True, log_device_placement=True))
+# Runs the op.
+print(sess.run(c))
+```
+
+## Using multiple GPUs
+
+If you would like to run TensorFlow on multiple GPUs, you can construct your
+model in a multi-tower fashion where each tower is assigned to a different GPU.
+For example:
+
+``` python
+# Creates a graph.
+c = []
+for d in ['/device:GPU:2', '/device:GPU:3']:
+ with tf.device(d):
+ a = tf.constant([1.0, 2.0, 3.0, 4.0, 5.0, 6.0], shape=[2, 3])
+ b = tf.constant([1.0, 2.0, 3.0, 4.0, 5.0, 6.0], shape=[3, 2])
+ c.append(tf.matmul(a, b))
+with tf.device('/cpu:0'):
+ sum = tf.add_n(c)
+# Creates a session with log_device_placement set to True.
+sess = tf.Session(config=tf.ConfigProto(log_device_placement=True))
+# Runs the op.
+print(sess.run(sum))
+```
+
+You will see the following output.
+
+```
+Device mapping:
+/job:localhost/replica:0/task:0/device:GPU:0 -> device: 0, name: Tesla K20m, pci bus
+id: 0000:02:00.0
+/job:localhost/replica:0/task:0/device:GPU:1 -> device: 1, name: Tesla K20m, pci bus
+id: 0000:03:00.0
+/job:localhost/replica:0/task:0/device:GPU:2 -> device: 2, name: Tesla K20m, pci bus
+id: 0000:83:00.0
+/job:localhost/replica:0/task:0/device:GPU:3 -> device: 3, name: Tesla K20m, pci bus
+id: 0000:84:00.0
+Const_3: /job:localhost/replica:0/task:0/device:GPU:3
+Const_2: /job:localhost/replica:0/task:0/device:GPU:3
+MatMul_1: /job:localhost/replica:0/task:0/device:GPU:3
+Const_1: /job:localhost/replica:0/task:0/device:GPU:2
+Const: /job:localhost/replica:0/task:0/device:GPU:2
+MatMul: /job:localhost/replica:0/task:0/device:GPU:2
+AddN: /job:localhost/replica:0/task:0/cpu:0
+[[ 44. 56.]
+ [ 98. 128.]]
+```
+
+The @{$deep_cnn$cifar10 tutorial} is a good example
+demonstrating how to do training with multiple GPUs.
* [`tensor_shape`](https://github.com/tensorflow/tensorflow/blob/master/tensorflow/core/framework/tensor_shape.proto)
* [`types`](https://github.com/tensorflow/tensorflow/blob/master/tensorflow/core/framework/types.proto)
-## What is *not* covered
+## What is *not* covered {not_covered}
Some API functions are explicitly marked as "experimental" and can change in
backward incompatible ways between minor releases. These include:
To find out more about implementing convolutional neural networks, you can jump
to the TensorFlow @{$deep_cnn$deep convolutional networks tutorial},
-or start a bit more gently with our
-@{$beginners$ML beginner} or @{$pros$ML expert}
-MNIST starter tutorials. Finally, if you want to get up to speed on research
-in this area, you can
+or start a bit more gently with our @{$layers$MNIST starter tutorial}.
+Finally, if you want to get up to speed on research in this area, you can
read the recent work of all the papers referenced in this tutorial.
# Tutorials
+
This section contains tutorials demonstrating how to do specific tasks
in TensorFlow. If you are new to TensorFlow, we recommend reading the
-documents in the "Get Started" section before reading these tutorials.
+documents in the "@{$get_started$Get Started}" section before reading
+these tutorials.
-The following tutorial explains the interaction of CPUs and GPUs on a
-TensorFlow system:
+## Images
- * @{$using_gpu$Using GPUs}
+These tutorials cover different aspects of image recognition:
-The following tutorials cover different aspects of image recognition:
+ * @{$layers}, which introduces convolutional neural networks (CNNs) and
+ demonstrates how to build a CNN in TensorFlow.
+ * @{$image_recognition}, which introduces the field of image recognition and
+ uses a pre-trained model (Inception) for recognizing images.
+ * @{$image_retraining}, which has a wonderfully self-explanatory title.
+ * @{$deep_cnn}, which demonstrates how to build a small CNN for recognizing
+ images. This tutorial is aimed at advanced TensorFlow users.
- * @{$image_recognition$Image Recognition}, which introduces the field of
- image recognition and a model (Inception) for recognizing images.
- * @{$image_retraining$How to Retrain Inception's Final Layer for New Categories},
- which has a wonderfully self-explanatory title.
- * @{$layers$A Guide to TF Layers: Building a Convolutional Neural Network},
- which introduces convolutional neural networks (CNNs) and demonstrates how
- to build a CNN in TensorFlow.
- * @{$deep_cnn$Convolutional Neural Networks}, which demonstrates how to
- build a small CNN for recognizing images. This tutorial is aimed at
- advanced TensorFlow users.
-The following tutorials focus on machine learning problems in human language:
+## Sequences
- * @{$word2vec$Vector Representations of Words}, which demonstrates how to
- create an embedding for words.
- * @{$recurrent$Recurrent Neural Networks}, which demonstrates how to use a
+These tutorials focus on machine learning problems dealing with sequence data.
+
+ * @{$recurrent}, which demonstrates how to use a
recurrent neural network to predict the next word in a sentence.
- * @{$seq2seq$Sequence-to-Sequence Models}, which demonstrates how to use a
+ * @{$seq2seq}, which demonstrates how to use a
sequence-to-sequence model to translate text from English to French.
+ * @{$recurrent_quickdraw}
+ builds a classification model for drawings, directly from the sequence of
+ pen strokes.
+ * @{$audio_recognition}, which shows how to
+ build a basic speech recognition network.
-The following tutorials focus on linear models:
+## Data representation
- * @{$linear$Large-Scale Linear Models with TensorFlow}, which introduces
- linear models and demonstrates how to build them with the high-level API.
- * @{$wide$TensorFlow Linear Model Tutorial}, which demonstrates how to solve
- a binary classification problem in TensorFlow.
- * @{$wide_and_deep$TensorFlow Wide & Deep Learning Tutorial}, which explains
- how to use the high-level API to jointly train both a wide linear model
- and a deep feed-forward neural network.
- * @{$kernel_methods$Improving Linear Models Using Explicit Kernel Methods},
+These tutorials demonstrate various data representations that can be used in
+TensorFlow.
+
+ * @{$wide}, uses
+ @{tf.feature_column$feature columns} to feed a variety of data types
+ to linear model, to solve a classification problem.
+ * @{$wide_and_deep}, builds on the
+ above linear model tutorial, adding a deep feed-forward neural network
+ component and a DNN-compatible data representation.
+ * @{$word2vec}, which demonstrates how to
+ create an embedding for words.
+ * @{$kernel_methods},
which shows how to improve the quality of a linear model by using explicit
kernel mappings.
- * @{$audio_recognition$Simple Audio Recognition}, which shows how to
- build a basic speech recognition network.
-
-The following tutorial covers building a classification model for sequences:
- * @{$recurrent_quickdraw$Classifying Drawings using Recurrent Neural Networks}
+## Non Machine Learning
-Although TensorFlow specializes in machine learning, you may also use
-TensorFlow to solve other kinds of math problems. For example:
+Although TensorFlow specializes in machine learning, the core of TensorFlow is
+a powerful numeric computation system which you can also use to solve other
+kinds of math problems. For example:
- * @{$mandelbrot$Mandelbrot Set}
- * @{$pdes$Partial Differential Equations}
+ * @{$mandelbrot}
+ * @{$pdes}
# Improving Linear Models Using Explicit Kernel Methods
+Note: This document uses a deprecated version of ${tf.estimator},
+which has a ${tf.contrib.learn.estimator$different interface}.
+It also uses other `contrib` methods whose
+${$version_compat#not_covered$API may not be stable}.
+
In this tutorial, we demonstrate how combining (explicit) kernel methods with
linear models can drastically increase the latters' quality of predictions
without significantly increasing training and inference times. Unlike dual
tutorial, we only use the train and validation splits to train and evaluate our
models respectively.
-In order to feed data to a tf.contrib.learn Estimator, it is helpful to convert
+In order to feed data to a `tf.contrib.learn Estimator`, it is helpful to convert
it to Tensors. For this, we will use an `input function` which adds Ops to the
TensorFlow graph that, when executed, create mini-batches of Tensors to be used
downstream. For more background on input functions, check
-@{$get_started/input_fn$Building Input Functions with tf.contrib.learn}. In this
-example, we will use the `tf.train.shuffle_batch` Op which, besides converting
-numpy arrays to Tensors, allows us to specify the batch_size and whether to
-randomize the input every time the input_fn Ops are executed (randomization
-typically expedites convergence during training). The full code for loading and
-preparing the data is shown in the snippet below. In this example, we use
-mini-batches of size 256 for training and the entire sample (5K entries) for
-evaluation. Feel free to experiment with different batch sizes.
+@{$get_started/premade_estimators#input_fn$this section on input functions}.
+In this example, we will use the `tf.train.shuffle_batch` Op which, besides
+converting numpy arrays to Tensors, allows us to specify the batch_size and
+whether to randomize the input every time the input_fn Ops are executed
+(randomization typically expedites convergence during training). The full code
+for loading and preparing the data is shown in the snippet below. In this
+example, we use mini-batches of size 256 for training and the entire sample
+(5K entries) for evaluation. Feel free to experiment with different batch sizes.
```python
import numpy as np
The following sections (with headings corresponding to each code block above)
dive deeper into the `tf.layers` code used to create each layer, as well as how
to calculate loss, configure the training op, and generate predictions. If
-you're already experienced with CNNs and @{$extend/estimators$TensorFlow `Estimator`s},
+you're already experienced with CNNs and @{$get_started/custom_estimators$TensorFlow `Estimator`s},
and find the above code intuitive, you may want to skim these sections or just
skip ahead to ["Training and Evaluating the CNN MNIST
Classifier"](#training-and-evaluating-the-cnn-mnist-classifier).
```
> Note: For a more in-depth look at configuring training ops for Estimator model
-> functions, see @{$extend/estimators#defining-the-training-op-for-the-model$"Defining
-> the training op for the model"} in the @{$extend/estimators$"Creating Estimations in
+> functions, see @{$get_started/custom_estimators#defining-the-training-op-for-the-model$"Defining
+> the training op for the model"} in the @{$get_started/custom_estimators$"Creating Estimations in
> tf.estimator"} tutorial.
### Add evaluation metrics
feel free to change to another directory of your choice).
> Note: For an in-depth walkthrough of the TensorFlow `Estimator` API, see the
-> tutorial @{$extend/estimators$"Creating Estimators in tf.estimator."}
+> tutorial @{$get_started/custom_estimators$"Creating Estimators in tf.estimator."}
### Set Up a Logging Hook {#set_up_a_logging_hook}
To learn more about TensorFlow Estimators and CNNs in TensorFlow, see the
following resources:
-* @{$extend/estimators$Creating Estimators in tf.estimator}. An
- introduction to the TensorFlow Estimator API, which walks through
+* @{$get_started/custom_estimators$Creating Estimators in tf.estimator}
+ provides an introduction to the TensorFlow Estimator API. It walks through
configuring an Estimator, writing a model function, calculating loss, and
defining a training op.
-* @{$pros#build-a-multilayer-convolutional-network$Deep MNIST for Experts: Building a Multilayer CNN}. Walks
- through how to build a MNIST CNN classification model *without layers* using
- lower-level TensorFlow operations.
+* @{$deep_cnn} walks through how to build a MNIST CNN classification model
+ *without estimators* using lower-level TensorFlow operations.
index.md
-using_gpu.md
+
+### Images
+layers.md
image_recognition.md
image_retraining.md
-layers.md
deep_cnn.md
-word2vec.md
+
+### Sequences
recurrent.md
-recurrent_quickdraw.md
seq2seq.md
-linear.md
+recurrent_quickdraw.md
+audio_recognition.md
+
+### Data Representation
wide.md
wide_and_deep.md
+word2vec.md
kernel_methods.md
-audio_recognition.md
+
+### Non-ML
mandelbrot.md
pdes.md
To understand this overview it will help to have some familiarity
with basic machine learning concepts, and also with
-@{$get_started/estimator$Estimators}.
+@{$get_started/premade_estimators$Estimators}.
[TOC]
## What is a linear model?
A **linear model** uses a single weighted sum of features to make a prediction.
-For example, if you have
-[data](https://archive.ics.uci.edu/ml/machine-learning-databases/adult/adult.names)
+For example, if you have [data](https://archive.ics.uci.edu/ml/machine-learning-databases/adult/adult.names)
on age, years of education, and weekly hours of
work for a population, a model can learn weights for each of those numbers so that
their weighted sum estimates a person's salary. You can also use linear models
for classification.
Some linear models transform the weighted sum into a more convenient form. For
-example,
-[**logistic regression**](https://developers.google.com/machine-learning/glossary/#logistic_regression)
-plugs the weighted sum into the logistic
+example, [**logistic regression**](https://developers.google.com/machine-learning/glossary/#logistic_regression) plugs the weighted sum into the logistic
function to turn the output into a value between 0 and 1. But you still just
have one weight for each input feature.
The input function must return a dictionary of tensors. Each key corresponds to
the name of a `FeatureColumn`. Each key's value is a tensor containing the
values of that feature for all data instances. See
-@{$input_fn$Building Input Functions} for a
+@{$premade_estimators#input_fn} for a
more comprehensive look at input functions, and `input_fn` in the
[linear models tutorial code](https://github.com/tensorflow/models/tree/master/official/wide_deep/wide_deep.py)
for an example implementation of an input function.
### Defining the model
To define the model we create a new `Estimator`. If you want to read more about
-estimators, we recommend @{$extend/estimators$this tutorial}.
+estimators, we recommend @{$get_started/custom_estimators$this tutorial}.
To build the model, we:
+++ /dev/null
-# Using GPUs
-
-## Supported devices
-
-On a typical system, there are multiple computing devices. In TensorFlow, the
-supported device types are `CPU` and `GPU`. They are represented as `strings`.
-For example:
-
-* `"/cpu:0"`: The CPU of your machine.
-* `"/device:GPU:0"`: The GPU of your machine, if you have one.
-* `"/device:GPU:1"`: The second GPU of your machine, etc.
-
-If a TensorFlow operation has both CPU and GPU implementations, the GPU devices
-will be given priority when the operation is assigned to a device. For example,
-`matmul` has both CPU and GPU kernels. On a system with devices `cpu:0` and
-`gpu:0`, `gpu:0` will be selected to run `matmul`.
-
-## Logging Device placement
-
-To find out which devices your operations and tensors are assigned to, create
-the session with `log_device_placement` configuration option set to `True`.
-
-```python
-# Creates a graph.
-a = tf.constant([1.0, 2.0, 3.0, 4.0, 5.0, 6.0], shape=[2, 3], name='a')
-b = tf.constant([1.0, 2.0, 3.0, 4.0, 5.0, 6.0], shape=[3, 2], name='b')
-c = tf.matmul(a, b)
-# Creates a session with log_device_placement set to True.
-sess = tf.Session(config=tf.ConfigProto(log_device_placement=True))
-# Runs the op.
-print(sess.run(c))
-```
-
-You should see the following output:
-
-```
-Device mapping:
-/job:localhost/replica:0/task:0/device:GPU:0 -> device: 0, name: Tesla K40c, pci bus
-id: 0000:05:00.0
-b: /job:localhost/replica:0/task:0/device:GPU:0
-a: /job:localhost/replica:0/task:0/device:GPU:0
-MatMul: /job:localhost/replica:0/task:0/device:GPU:0
-[[ 22. 28.]
- [ 49. 64.]]
-
-```
-
-## Manual device placement
-
-If you would like a particular operation to run on a device of your choice
-instead of what's automatically selected for you, you can use `with tf.device`
-to create a device context such that all the operations within that context will
-have the same device assignment.
-
-```python
-# Creates a graph.
-with tf.device('/cpu:0'):
- a = tf.constant([1.0, 2.0, 3.0, 4.0, 5.0, 6.0], shape=[2, 3], name='a')
- b = tf.constant([1.0, 2.0, 3.0, 4.0, 5.0, 6.0], shape=[3, 2], name='b')
-c = tf.matmul(a, b)
-# Creates a session with log_device_placement set to True.
-sess = tf.Session(config=tf.ConfigProto(log_device_placement=True))
-# Runs the op.
-print(sess.run(c))
-```
-
-You will see that now `a` and `b` are assigned to `cpu:0`. Since a device was
-not explicitly specified for the `MatMul` operation, the TensorFlow runtime will
-choose one based on the operation and available devices (`gpu:0` in this
-example) and automatically copy tensors between devices if required.
-
-```
-Device mapping:
-/job:localhost/replica:0/task:0/device:GPU:0 -> device: 0, name: Tesla K40c, pci bus
-id: 0000:05:00.0
-b: /job:localhost/replica:0/task:0/cpu:0
-a: /job:localhost/replica:0/task:0/cpu:0
-MatMul: /job:localhost/replica:0/task:0/device:GPU:0
-[[ 22. 28.]
- [ 49. 64.]]
-```
-
-## Allowing GPU memory growth
-
-By default, TensorFlow maps nearly all of the GPU memory of all GPUs (subject to
-[`CUDA_VISIBLE_DEVICES`](https://docs.nvidia.com/cuda/cuda-c-programming-guide/index.html#env-vars))
-visible to the process. This is done to more efficiently use the relatively
-precious GPU memory resources on the devices by reducing [memory
-fragmentation](https://en.wikipedia.org/wiki/Fragmentation_\(computing\)).
-
-In some cases it is desirable for the process to only allocate a subset of the
-available memory, or to only grow the memory usage as is needed by the process.
-TensorFlow provides two Config options on the Session to control this.
-
-The first is the `allow_growth` option, which attempts to allocate only as much
-GPU memory based on runtime allocations: it starts out allocating very little
-memory, and as Sessions get run and more GPU memory is needed, we extend the GPU
-memory region needed by the TensorFlow process. Note that we do not release
-memory, since that can lead to even worse memory fragmentation. To turn this
-option on, set the option in the ConfigProto by:
-
-```python
-config = tf.ConfigProto()
-config.gpu_options.allow_growth = True
-session = tf.Session(config=config, ...)
-```
-
-The second method is the `per_process_gpu_memory_fraction` option, which
-determines the fraction of the overall amount of memory that each visible GPU
-should be allocated. For example, you can tell TensorFlow to only allocate 40%
-of the total memory of each GPU by:
-
-```python
-config = tf.ConfigProto()
-config.gpu_options.per_process_gpu_memory_fraction = 0.4
-session = tf.Session(config=config, ...)
-```
-
-This is useful if you want to truly bound the amount of GPU memory available to
-the TensorFlow process.
-
-## Using a single GPU on a multi-GPU system
-
-If you have more than one GPU in your system, the GPU with the lowest ID will be
-selected by default. If you would like to run on a different GPU, you will need
-to specify the preference explicitly:
-
-```python
-# Creates a graph.
-with tf.device('/device:GPU:2'):
- a = tf.constant([1.0, 2.0, 3.0, 4.0, 5.0, 6.0], shape=[2, 3], name='a')
- b = tf.constant([1.0, 2.0, 3.0, 4.0, 5.0, 6.0], shape=[3, 2], name='b')
- c = tf.matmul(a, b)
-# Creates a session with log_device_placement set to True.
-sess = tf.Session(config=tf.ConfigProto(log_device_placement=True))
-# Runs the op.
-print(sess.run(c))
-```
-
-If the device you have specified does not exist, you will get
-`InvalidArgumentError`:
-
-```
-InvalidArgumentError: Invalid argument: Cannot assign a device to node 'b':
-Could not satisfy explicit device specification '/device:GPU:2'
- [[Node: b = Const[dtype=DT_FLOAT, value=Tensor<type: float shape: [3,2]
- values: 1 2 3...>, _device="/device:GPU:2"]()]]
-```
-
-If you would like TensorFlow to automatically choose an existing and supported
-device to run the operations in case the specified one doesn't exist, you can
-set `allow_soft_placement` to `True` in the configuration option when creating
-the session.
-
-```python
-# Creates a graph.
-with tf.device('/device:GPU:2'):
- a = tf.constant([1.0, 2.0, 3.0, 4.0, 5.0, 6.0], shape=[2, 3], name='a')
- b = tf.constant([1.0, 2.0, 3.0, 4.0, 5.0, 6.0], shape=[3, 2], name='b')
- c = tf.matmul(a, b)
-# Creates a session with allow_soft_placement and log_device_placement set
-# to True.
-sess = tf.Session(config=tf.ConfigProto(
- allow_soft_placement=True, log_device_placement=True))
-# Runs the op.
-print(sess.run(c))
-```
-
-## Using multiple GPUs
-
-If you would like to run TensorFlow on multiple GPUs, you can construct your
-model in a multi-tower fashion where each tower is assigned to a different GPU.
-For example:
-
-```
-# Creates a graph.
-c = []
-for d in ['/device:GPU:2', '/device:GPU:3']:
- with tf.device(d):
- a = tf.constant([1.0, 2.0, 3.0, 4.0, 5.0, 6.0], shape=[2, 3])
- b = tf.constant([1.0, 2.0, 3.0, 4.0, 5.0, 6.0], shape=[3, 2])
- c.append(tf.matmul(a, b))
-with tf.device('/cpu:0'):
- sum = tf.add_n(c)
-# Creates a session with log_device_placement set to True.
-sess = tf.Session(config=tf.ConfigProto(log_device_placement=True))
-# Runs the op.
-print(sess.run(sum))
-```
-
-You will see the following output.
-
-```
-Device mapping:
-/job:localhost/replica:0/task:0/device:GPU:0 -> device: 0, name: Tesla K20m, pci bus
-id: 0000:02:00.0
-/job:localhost/replica:0/task:0/device:GPU:1 -> device: 1, name: Tesla K20m, pci bus
-id: 0000:03:00.0
-/job:localhost/replica:0/task:0/device:GPU:2 -> device: 2, name: Tesla K20m, pci bus
-id: 0000:83:00.0
-/job:localhost/replica:0/task:0/device:GPU:3 -> device: 3, name: Tesla K20m, pci bus
-id: 0000:84:00.0
-Const_3: /job:localhost/replica:0/task:0/device:GPU:3
-Const_2: /job:localhost/replica:0/task:0/device:GPU:3
-MatMul_1: /job:localhost/replica:0/task:0/device:GPU:3
-Const_1: /job:localhost/replica:0/task:0/device:GPU:2
-Const: /job:localhost/replica:0/task:0/device:GPU:2
-MatMul: /job:localhost/replica:0/task:0/device:GPU:2
-AddN: /job:localhost/replica:0/task:0/cpu:0
-[[ 44. 56.]
- [ 98. 128.]]
-```
-
-The @{$deep_cnn$cifar10 tutorial} is a good example
-demonstrating how to do training with multiple GPUs.